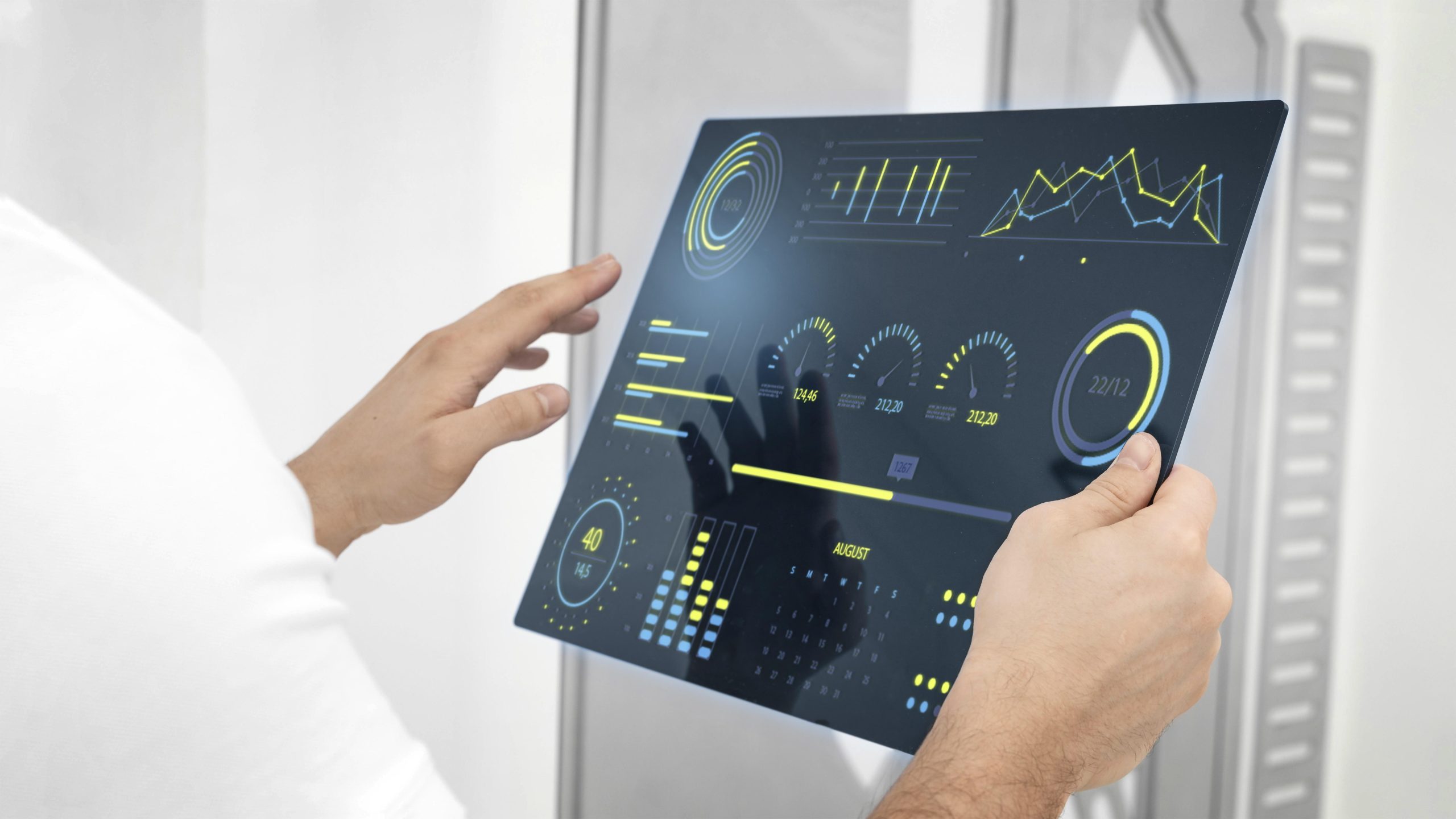
Tracking performance and errors in AI workflows
Artificial Intelligence (AI) workflows are essential for automating tasks, processing large datasets, and making intelligent decisions. However, ensuring they run smoothly requires robust monitoring techniques. Without proper tracking, errors can go unnoticed, leading to costly inefficiencies.
In this article, we’ll explore the best practices for AI workflow monitoring, helping you improve performance, detect errors early, and optimize your AI systems for peak efficiency.
Why AI Workflow Monitoring is Important
AI models and workflows can encounter issues such as:
- Data drift impacting model accuracy
- Performance bottlenecks slowing down processes
- Uncaught errors leading to incorrect results
- Resource overuse causing excessive costs
By implementing proper monitoring techniques, businesses can proactively detect these problems and maintain a smooth AI pipeline.
Key Best Practices for AI Workflow Monitoring
1. Establish Clear Performance Metrics
To effectively monitor AI workflows, define key performance indicators (KPIs). These metrics should align with your business goals and technical requirements.
Common AI Workflow KPIs:
- Accuracy & Precision: Measures how well AI models perform.
- Latency & Processing Time: Tracks execution speed.
- Resource Utilization: Monitors CPU, GPU, and memory usage.
- Error Rate: Detects failures and misclassifications.
By tracking these KPIs, you can identify performance trends and optimize workflows accordingly.
2. Implement Real-Time Monitoring Tools
Real-time monitoring enables immediate detection of issues, preventing disruptions before they escalate.
Recommended Monitoring Tools:
- Prometheus – Open-source tool for real-time analytics
- Grafana – Visualization tool for AI performance metrics
- ELK Stack (Elasticsearch, Logstash, Kibana) – Logs and event monitoring
- TensorBoard – Performance tracking for machine learning models
Using these tools, AI teams can set up alerts, visualize trends, and troubleshoot bottlenecks.
3. Automate Error Detection and Alerts
AI workflows should include automated error detection systems. Automated alerts help teams respond quickly to issues, minimizing downtime.
Best Practices for Automated Alerts:
- Set up threshold-based alerts (e.g., if accuracy drops below 90%)
- Configure notifications via Slack, email, or SMS
- Integrate anomaly detection algorithms to catch unexpected issues
4. Monitor Data Quality and Integrity
Poor data quality can degrade AI performance. Continuous monitoring ensures data consistency and reliability.
Data Quality Metrics to Track:
- Missing Values: Identifies gaps in datasets
- Data Drift: Detects shifts in input data distribution
- Duplicate Entries: Ensures unique, accurate records
- Outliers: Flags anomalous data points
Using tools like Great Expectations or Pandas Profiling, teams can automate data validation checks.
5. Use Logging and Auditing for Debugging
Comprehensive logs allow engineers to trace AI failures and optimize performance.
Best Logging Practices:
- Store structured logs with timestamps and severity levels
- Use log aggregators like Fluentd or Logstash
- Maintain an audit trail for compliance and debugging
6. Optimize Resource Utilization
AI workflows often require high computational power. Monitoring resource usage can reduce costs and enhance efficiency.
How to Optimize Resources:
- Use auto-scaling for cloud-based AI workloads
- Optimize hyperparameters to reduce model complexity
- Monitor GPU/CPU usage with Kubernetes or AWS CloudWatch
Balancing performance with cost efficiency ensures sustainable AI deployment.
Frequently Asked Questions (FAQ)
1. What is AI workflow monitoring?
AI workflow monitoring involves tracking AI models and processes to ensure they operate efficiently, detect errors, and optimize performance.
2. What are the best tools for AI monitoring?
Popular tools include Prometheus, Grafana, ELK Stack, and TensorBoard, which provide real-time insights and performance analytics.
3. How can I detect data drift in AI workflows?
Data drift can be detected using monitoring tools that compare input data distribution over time, such as Great Expectations or built-in model monitoring solutions.
4. Why is real-time monitoring important for AI?
Real-time monitoring helps catch errors and performance drops instantly, preventing costly issues and ensuring smooth AI operation.
5. What KPIs should I track in AI workflow monitoring?
Key metrics include accuracy, processing time, error rate, and resource utilization.
Conclusion
Effective AI workflow monitoring ensures smooth operations, high performance, and quick error resolution. By leveraging real-time monitoring, automating alerts, and optimizing resources, businesses can maintain robust AI workflows.
Implement these best practices today to enhance your AI system’s reliability and efficiency!
Author Profile
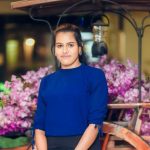
- Online Media & PR Strategist
- Hello there! I'm Online Media & PR Strategist at NeticSpace | Passionate Journalist, Blogger, and SEO Specialist
Latest entries
Digital Twin DevelopmentApril 30, 2025How to Ensure Data Synchronization Twins Effectively
Scientific VisualizationApril 30, 2025Deepfake Scientific Data: AI-Generated Fraud in Research
Data AnalyticsApril 30, 2025What Is Data Mesh Architecture and Why It’s Trending
Rendering and VisualizationApril 30, 2025Metaverse Rendering Challenges and Opportunities