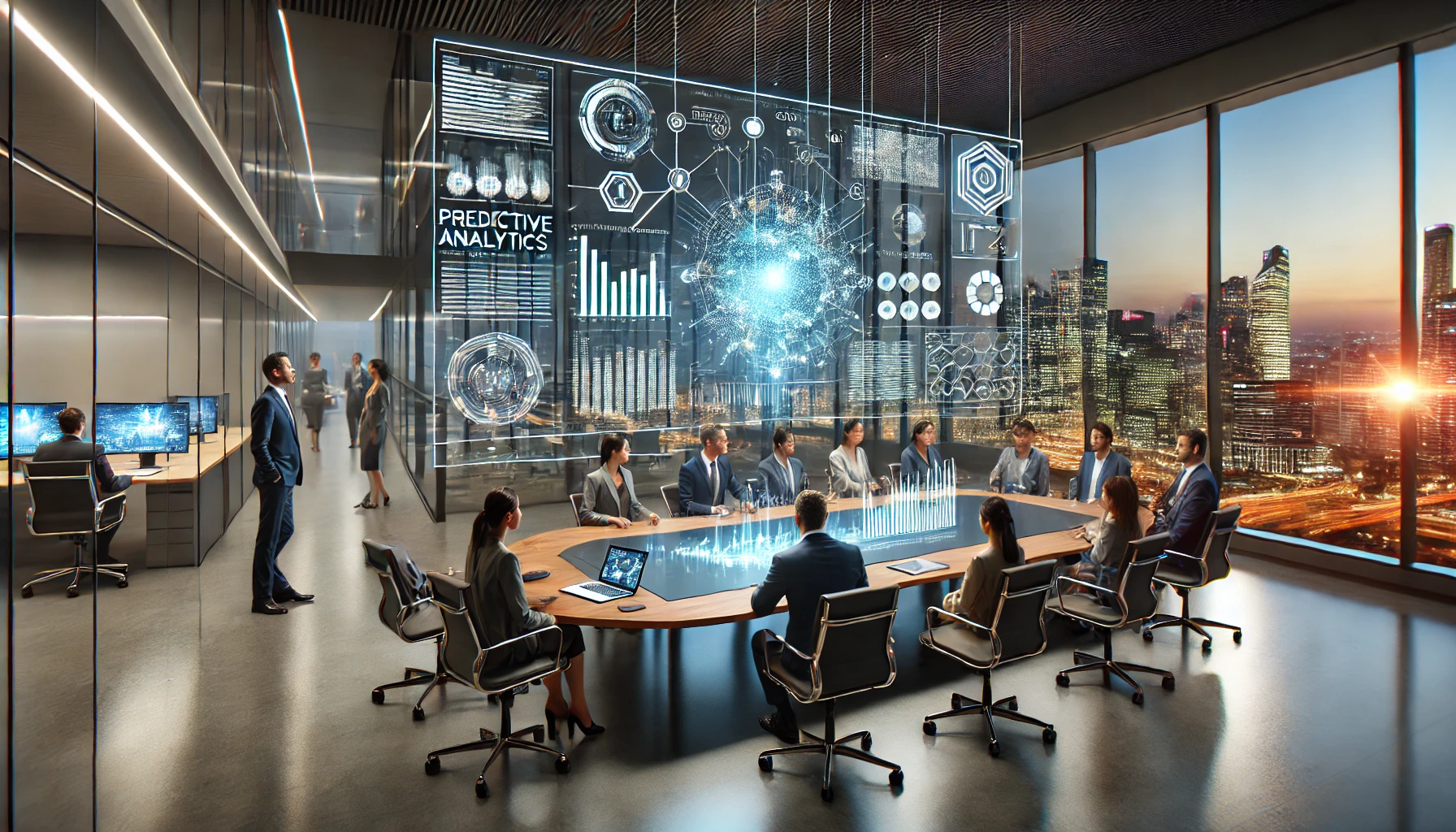
The Role of Predictive Analytics in IT Strategy
Predicting the future used to be guesswork. Today, IT leaders can rely on data-driven insights. Predictive analytics offers a proactive way to anticipate potential challenges and plan solutions in advance. In this blog post, you will learn how predictive analytics can transform IT strategy, drive efficiency, and create a competitive edge.
Introduction
IT environments grow more complex each day. They involve hardware, software, networks, and cloud resources. Without a clear strategy, even small disruptions can lead to huge losses. Predictive analytics helps you spot red flags early and take action before problems escalate.
In this article, we will examine the role of predictive analytics in IT strategy. You will see how it differs from traditional analytics. You will discover its benefits and practical applications. By the end, you will have a roadmap for implementation that can align IT operations with business goals.
Understanding Predictive Analytics for IT Strategy
What Is Predictive Analytics?
Predictive analytics uses historical data and algorithms to forecast future outcomes. It goes beyond just describing past events. Instead, it focuses on spotting patterns and trends to guide forward-looking decisions. Data mining, statistical modeling, and machine learning all come together to make these forecasts possible.
Predictive analytics in IT strategy involves using these insights to improve operations and reduce risks. Traditional IT analytics often looks at past incidents to understand what happened. Predictive analytics, on the other hand, helps you decide what to do next. This proactive stance can mean the difference between smooth operations and major disruptions.
Key Components of Predictive Analytics
- Data Collection and Preparation
First, you must gather data from various sources. These sources can include system logs, network performance metrics, and user activity reports. Data must then be cleansed and standardized to ensure accuracy and consistency.
- Statistical Modeling and Algorithms
Next, analysts apply statistical techniques to detect patterns in the data. These might include regression analysis, decision trees, or time-series models. The goal is to find meaningful relationships that can predict likely outcomes.
- Machine Learning and AI
Machine learning and AI enable models to learn from data as conditions evolve. Over time, these algorithms can refine their predictions based on new data or changes in the IT environment.
- Data Visualization and Interpretation
Finally, results should be presented in easy-to-understand formats. Charts, dashboards, and reports help decision-makers interpret findings. When insights are clear, it is simpler to take appropriate actions.
Traditional IT Analytics vs. Predictive Analytics
Traditional analytics focuses on describing what has already happened. Teams often act after issues arise. Predictive analytics, however, shifts the focus to preventing issues by anticipating future scenarios. This approach fosters proactive planning rather than reactive damage control. In today’s fast-paced environment, forward-thinking strategies can give your organization a distinct advantage.
Benefits of Integrating Predictive Analytics into IT Strategy
Proactive Risk Management
One of the most significant benefits of predictive analytics for IT strategy is proactive risk management. Complex systems can fail for many reasons. Security breaches, hardware breakdowns, and capacity limits can all cause problems. By using predictive models, IT teams can identify potential disruptions long before they happen.
Examples of Risk Mitigation
Predicting Security Threats and Vulnerabilities
Machine learning can detect unusual network activities. It can also flag suspicious login attempts or data flows. Early detection reduces the impact of cyberattacks.
Forecasting System Failures and Outages
Historical data on server performance can reveal signs of impending failures. These signs could be spikes in CPU usage or repeated crashes. Addressing these warning signals early prevents costly downtime.
Identifying Capacity Constraints
By forecasting resource usage, IT leaders can plan upgrades or migrations. This ensures the system won’t be overwhelmed when usage increases.
Optimized Resource Allocation
Predictive analytics can help IT teams manage budgets more effectively. It can guide decisions on infrastructure, software licenses, and staffing. Instead of allocating resources based on gut feelings, leaders can base these decisions on data patterns and forecasts.
Resource Optimization Strategies
Forecasting IT Infrastructure Requirements
By tracking network load, storage usage, and processing needs, teams can plan for scaling. This prevents bottlenecks and ensures smoother operations.
Optimizing Software Licensing
Software licenses can be expensive. By predicting usage, organizations avoid over- or under-licensing. This saves money and ensures staff have the tools they need.
Predicting Staffing Needs
Skills gaps can slow productivity. Predictive analytics can estimate future project demands. This helps leaders decide whether to hire new talent or train current staff.
Enhanced IT Efficiency
Efficiency in IT means doing more with fewer resources. Predictive analytics can highlight areas where operations can be streamlined. Automated alerts for potential incidents reduce manual checks and free staff for high-value tasks.
Efficiency Improvements through Predictive Analytics
Automating Routine Tasks and Incident Management
Predictive models can trigger scripts that fix minor issues automatically. This lowers the workload for IT support teams.
Optimizing System Performance and Resource Utilization
With predictive insights, IT can configure servers and networks for peak efficiency. This boosts performance and reduces costs.
Predictive Maintenance to Minimize Downtime
Regular maintenance is easier when guided by accurate predictions. Identifying failing components early allows you to schedule repairs without affecting users.
Improved Business Alignment
An IT strategy that aligns with business goals can drive revenue and improve customer experiences. Predictive analytics helps IT teams better understand customer behavior, market shifts, and organizational objectives. This ensures that IT decisions support broader company strategies rather than operating in isolation.
Use Cases of Predictive Analytics in IT Strategy
Real-world examples show how predictive analytics in IT strategy can deliver tangible results. Different industries benefit in different ways. Here are some of the most impactful applications.
Industry-Specific Examples
Finance
Predictive models catch suspicious activities in real time. They also help in credit risk assessments. In addition, banks use these models for personalized offers to high-value customers.
Healthcare
Hospitals and clinics use forecasts to manage patient admissions. This allows them to optimize staff schedules and resources. Predictive analytics can also identify disease trends to support public health measures.
Retail
Retailers keep customers loyal by predicting churn and personalizing offers. They also optimize their supply chains by using sales forecasts. This prevents both stock shortages and overstock issues.
Cross-Industry Applications
- Predictive Maintenance
For any business with complex machinery, predictive maintenance is crucial. It lowers operational costs and boosts equipment reliability.
- Security Analytics
Organizations across all sectors use predictive analytics to detect breaches and attacks early. This reduces the risk of reputational damage.
- Capacity Planning
Every industry can benefit from accurate capacity planning for their IT infrastructure. This avoids slow service and ensures readiness for peak loads.
Implementing Predictive Analytics in Your IT Strategy
Predictive analytics can seem daunting. But with the right approach, you can smoothly integrate it into your IT strategy. Below is a structured, step-by-step guide.
Key Steps for Implementation
- Define Clear Business Objectives and KPIs
First, determine what you want to achieve. Are you focusing on reducing downtime, boosting security, or optimizing costs? Identify the metrics that matter to your business. These objectives will shape the scope of your predictive analytics project.
- Identify Relevant Data Sources and Ensure Data Quality
Next, gather data from your systems, logs, and external tools. Clean and validate this data to remove errors. Reliable data is the foundation of accurate models.
- Select Appropriate Modeling Techniques and Tools
Then, choose algorithms suited to your use case. Common options include linear regression, decision trees, and neural networks. Many software tools offer built-in machine learning capabilities. Evaluate them based on ease of use, cost, and integration options.
- Develop and Deploy Predictive Models
After finalizing your models, integrate them into your IT workflows. For instance, you can embed models into monitoring dashboards. You can also use them in automation tools that trigger proactive alerts.
- Monitor Model Performance and Continuously Refine
Finally, your models will need updates as conditions change. Regularly track their accuracy and adjust parameters as needed. Ongoing maintenance ensures they remain relevant over time.
Choosing the Right Tools and Technologies
Selecting the right tools can make or break your predictive analytics journey. You need platforms that fit your organization’s size, budget, and technical skill level. Popular cloud-based solutions like AWS, Azure, or Google Cloud offer scalable machine learning services. Open-source libraries (such as TensorFlow or scikit-learn) provide flexibility but require more in-house expertise.
Some organizations prefer specialized dashboards and balanced scorecards. Tools like **Spider Impact** integrate predictive analytics into broader performance management. This helps in linking IT metrics to strategic business goals. Commercial software can offer user-friendly interfaces and dedicated support, but may have higher costs.
Conclusion
Predictive analytics is more than just a trend. It’s a strategic tool that can give IT leaders foresight and confidence. By leveraging historical data and advanced modeling, organizations can anticipate problems, optimize resources, and align IT efforts with business objectives. In a world where technology fuels every aspect of operations, predictive analytics offers a competitive edge.
Now is the time to embrace this forward-thinking approach. Start small by identifying a key issue or a single business objective. Then build on your success with broader initiatives. With careful planning, the transformative power of predictive analytics can reshape your IT strategy for the better.
FAQs
1. What Are the Most Common Challenges in Implementing Predictive Analytics for IT Strategy?
A major challenge is data quality. Incomplete or inaccurate data will yield weak predictions. Another challenge is selecting the right algorithms and tools. Without proper expertise, you risk misinterpreting results. Lastly, gaining stakeholder buy-in can be difficult if teams are wary of new processes or fear automation.
2. What Skills Are Needed to Build and Manage Predictive Models for IT?
Data science, machine learning, and programming are important skills. Familiarity with tools like Python, R, or specialized analytics software is also helpful. Strong analytical thinking is key to interpreting results. Additionally, knowledge of IT infrastructure ensures that models align with technical realities.
3. How Can Organizations Measure the ROI of Predictive Analytics in IT?
ROI can be measured by looking at reduced downtime, improved security, and better resource utilization. Financial metrics like cost savings or revenue growth are also relevant. Project-specific metrics, such as faster incident resolution times, can offer direct evidence of the impact. Surveys of user satisfaction and productivity gains provide further proof of value.
4. What Are Some Best Practices for Ensuring Data Security and Privacy When Using Predictive Analytics?
Always encrypt data in transit and at rest. Limit access to sensitive information using role-based permissions. Follow compliance regulations like GDPR or HIPAA when handling personal data. It’s also essential to maintain audit logs and conduct regular security assessments. By respecting privacy and implementing strong security measures, organizations can safely reap the benefits of predictive analytics.
Author Profile
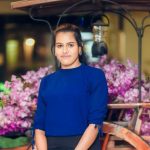
- Online Media & PR Strategist
- Hello there! I'm Online Media & PR Strategist at NeticSpace | Passionate Journalist, Blogger, and SEO Specialist
Latest entries
HPC and AIApril 30, 2025AI and HPC in Gaming: Realistic Virtual Worlds Today
Robotics SimulationApril 30, 2025How Robotics Simulation Agriculture Is Changing Farming
VirtualizationApril 30, 2025Future-Proof Virtualization Strategy for Emerging Tech
Simulation and ModelingApril 30, 2025Chaos Engineering: Build Resilient Systems with Chaos Monkey