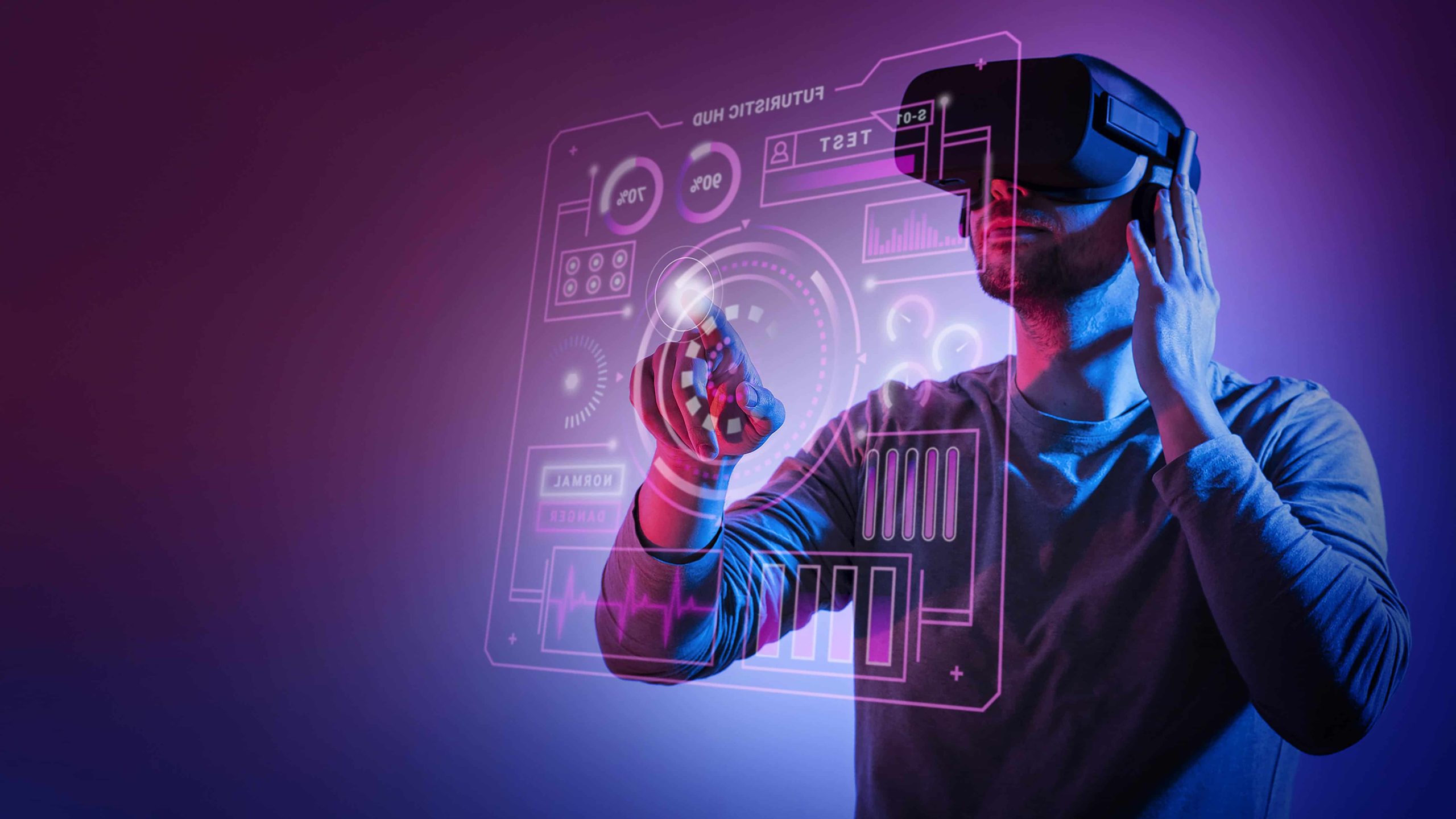
MLOps 2.0: The Future of Machine Learning Operations
Machine learning has evolved fast, but now a bigger shift is happening. MLOps 2.0 is transforming how we manage machine learning operations. In this blog, you’ll learn what MLOps 2.0 is, how it differs from earlier models, and what it means for businesses and developers alike. Let’s dive into the future of machine learning.
What Is MLOps 2.0 in Machine Learning Operations?
First, let’s define MLOps 2.0. It’s the next generation of machine learning operations that focuses on automation, scalability, and better collaboration between teams. While MLOps 1.0 was about managing machine learning models, MLOps 2.0 is about creating a fully automated system from data gathering to deployment.
-
Automated pipelines: Models are trained, tested, and deployed without human intervention.
-
Scalable infrastructure: Cloud-native tools allow for handling thousands of models.
-
Collaboration-first approach: Data scientists, DevOps, and business teams work closely using integrated tools.
For more on cloud-native MLOps tools, check out Google Cloud AI solutions.
Key Features of MLOps 2.0 in Machine Learning
Next, let’s look at the critical features that define MLOps 2.0:
1. End-to-End Automation in Machine Learning Operations
Automation is no longer optional. In MLOps 2.0, every stage — data preparation, model training, validation, and deployment — is automated.
Benefits include:
-
Faster model updates
-
Less manual error
-
More time for innovation
2. Model Monitoring and Observability for Machine Learning Operations
Monitoring models after deployment is a top priority. MLOps 2.0 improves model observability with real-time performance tracking.
Key aspects:
-
Drift detection
-
Automatic retraining
-
Performance dashboards
3. Continuous Integration and Continuous Deployment (CI/CD) in Machine Learning Operations
CI/CD pipelines now extend to machine learning models. This enables faster updates and quick rollbacks when needed.
With CI/CD:
-
Bugs are caught early
-
Deployment becomes safer
-
Teams can scale operations easily
Learn how Microsoft Azure MLOps helps build CI/CD for ML models.
Challenges to Overcome in MLOps 2.0 for Machine Learning Operations
Finally, it’s important to know the challenges. Although MLOps 2.0 brings improvements, teams still face hurdles:
-
Data security and privacy concerns
-
Need for specialized skills
-
Managing large and complex model ecosystems
Organizations must invest in training and secure infrastructure to fully benefit from MLOps 2.0.
How to Prepare for the Future of Machine Learning Operations
Preparation is key to success with MLOps 2.0. Here are some steps:
1. Build Cross-Functional Teams for Machine Learning
A good team has data scientists, machine learning engineers, and DevOps experts. Collaboration is at the heart of MLOps 2.0.
2. Invest in Cloud Solutions for Machine Learning Operations
Cloud platforms offer scalability and ready-to-use MLOps tools. They reduce the burden of managing infrastructure.
3. Focus on Compliance and Ethics in Machine Learning
Ethical AI is becoming crucial. Ensure your models are transparent, unbiased, and compliant with regulations.
For ethical AI practices, visit IBM’s Trustworthy AI page.
FAQs
What is the difference between MLOps 1.0 and MLOps 2.0?
MLOps 2.0 focuses on end-to-end automation, continuous monitoring, and better collaboration, unlike MLOps 1.0, which was model-centric.
Why is MLOps 2.0 important for businesses?
It helps deploy machine learning models faster, reduces manual errors, and ensures better model performance after deployment.
How can I get started with MLOps 2.0 in machine learning?
Start by automating your workflows, building cross-functional teams, and using cloud-native tools for scalability.
Author Profile
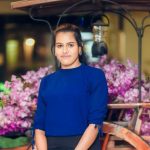
- Online Media & PR Strategist
- Hello there! I'm Online Media & PR Strategist at NeticSpace | Passionate Journalist, Blogger, and SEO Specialist
Latest entries
Data AnalyticsJune 13, 2025Future of Data Warehousing in Big Data
AI InterfaceJune 13, 2025Aligning AI Developments with Corporate Goals in the AI Era
HPC and AIJune 13, 2025HPC Architecture Taking to the Next Level
Quantum ComputingJune 13, 2025Ethical Issues in Quantum Tech: Privacy, Jobs, and Policy