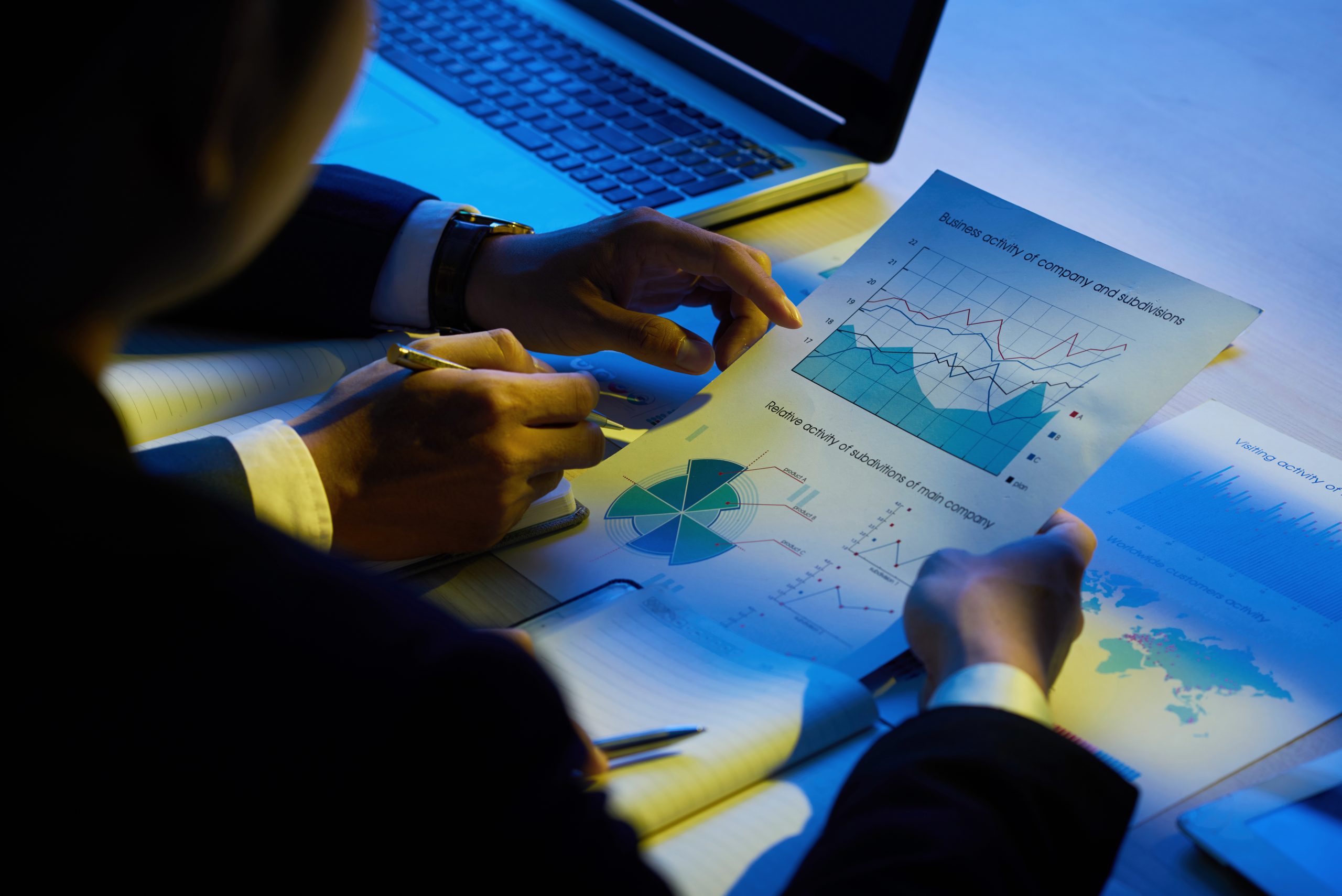
HPC and AI in Financial Modeling: Predicting Market Trends
Financial markets generate vast amounts of data every second. Traditional methods struggle to keep up, but High-Performance Computing (HPC) and Artificial Intelligence (AI) are changing the game. These technologies help financial analysts make more accurate predictions, mitigate risks, and seize profitable opportunities. In this article, you’ll learn how HPC and AI enhance financial modeling and their impact on market trend prediction.
How HPC and AI Improve Financial Modeling
1. High-Speed Data Processing with HPC
HPC enables financial institutions to process massive datasets in real-time. Financial markets generate terabytes of data from transactions, social media, and economic indicators. HPC systems use parallel processing to analyze this data rapidly, improving the accuracy of financial models.
Key Benefits of HPC in Financial Modeling:
- Faster Computation: Reduces time for complex calculations from hours to minutes.
- Risk Analysis: Processes multiple risk scenarios simultaneously.
- Backtesting Strategies: Tests trading algorithms on historical data quickly.
2. AI for Pattern Recognition and Prediction
AI algorithms detect patterns in historical data and use them to predict future trends. Machine learning (ML) models analyze price movements, market sentiment, and external factors like geopolitical events.
How AI Enhances Market Trend Predictions:
- Deep Learning Models: Identify complex patterns in stock prices.
- Natural Language Processing (NLP): Analyzes news, earnings reports, and social media sentiment.
- Reinforcement Learning: Adapts trading strategies based on market changes.
3. Combining AI and HPC for Superior Market Insights
By integrating HPC and AI, financial firms can create more sophisticated models. AI-powered algorithms generate insights, while HPC handles the large-scale computations.
Use Cases in Finance:
- Algorithmic Trading: AI-powered trading bots execute trades in milliseconds.
- Fraud Detection: Analyzes millions of transactions to spot anomalies.
- Portfolio Optimization: Suggests the best asset allocations based on real-time data.
Challenges and Considerations
While HPC and AI provide numerous benefits, they also present challenges:
- Data Privacy & Security: Handling vast amounts of sensitive financial data requires robust cybersecurity measures.
- Computational Costs: HPC infrastructure and AI models demand significant investments.
- Regulatory Compliance: Financial regulations impact how AI can be used in trading and modeling.
The Future of HPC and AI in Finance
As technology advances, HPC and AI will become even more integral to financial modeling. Quantum computing, improved ML algorithms, and better data integration techniques will further refine market trend predictions.
What’s Next?
- Quantum Computing: Offers unprecedented processing power for financial models.
- Enhanced AI Explainability: Helps traders understand AI-driven decisions.
- More Real-Time Analysis: Reduces latency in financial predictions.
FAQs
1. How does AI predict stock market trends?
AI uses machine learning models to analyze historical data, market sentiment, and external events to predict future trends.
2. Is HPC necessary for financial modeling?
Yes, HPC enables high-speed data processing, essential for analyzing vast financial datasets efficiently.
3. Can AI replace human financial analysts?
AI enhances analysts’ capabilities but does not replace them. Human oversight is crucial for interpreting AI-generated insights.
4. What industries beyond finance use HPC and AI?
Other industries include healthcare, engineering, climate modeling, and cybersecurity, where complex data processing is needed.
Author Profile
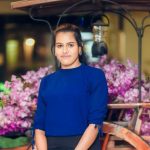
- Online Media & PR Strategist
- Hello there! I'm Online Media & PR Strategist at NeticSpace | Passionate Journalist, Blogger, and SEO Specialist
Latest entries
Data AnalyticsJune 13, 2025Future of Data Warehousing in Big Data
AI InterfaceJune 13, 2025Aligning AI Developments with Corporate Goals in the AI Era
HPC and AIJune 13, 2025HPC Architecture Taking to the Next Level
Quantum ComputingJune 13, 2025Ethical Issues in Quantum Tech: Privacy, Jobs, and Policy