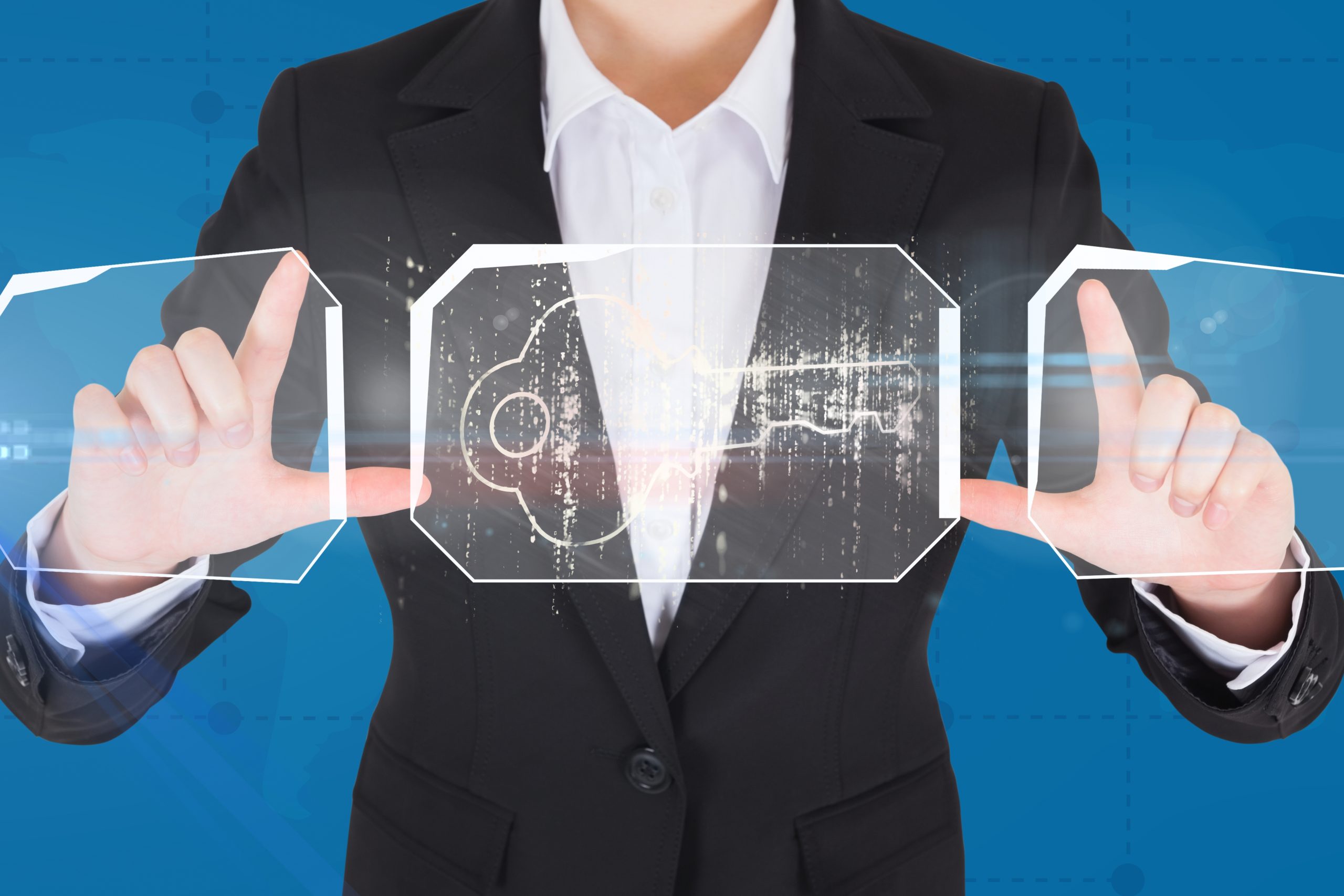
Generative Systems and Responsible AI Guidelines
AI is evolving fast, and it’s important to know how to use it responsibly. In this post, you’ll learn what Generative Systems and Responsible AI Guidelines are, how they impact our digital world, and what steps developers and companies should take to stay ethical.
By the end, you’ll understand the basics, the risks, the benefits, and the rules that help keep AI in check.
What Are AI and generative systems guidelines?
AI and generative systems guidelines refer to how AI tools create content and the rules to ensure they do so ethically. These systems use machine learning models to produce text, images, music, and more. But with power comes responsibility.
Why These Guidelines Matter
-
They help protect users from bias, misinformation, and harm.
-
They keep companies accountable for their AI tools.
-
They build trust in AI technologies.
Without these guardrails, generative AI can cause legal, ethical, and even financial problems.
Benefits of Generative Systems and Responsible AI Guidelines
There are many advantages to using AI and generative systems guidelines. When applied properly, these guidelines:
Help Build Ethical AI
-
Reduce harmful outputs (like hate speech).
-
Prevent plagiarism and intellectual property misuse.
Encourage Transparency
-
Make AI behavior easier to understand.
-
Require clear documentation for AI models.
Support Regulation
-
Align with laws like the EU AI Act and OECD AI Principles.
Risks Without Generative Systems and Responsible AI Guidelines
Without Generative Systems and Responsible AI Guidelines, organizations may face:
Security and Privacy Issues
-
Sensitive data leaks
-
Deepfakes and fraud
Ethical Violations
-
Racial and gender bias
-
Unintended harmful content
Legal Problems
-
Non-compliance with privacy laws like GDPR
-
Copyright infringement
That’s why adopting responsible AI practices is no longer optional.
Core Principles of AI and generative systems guidelines
Here are the most important principles for managing Generative Systems and Responsible AI Guidelines:
1. Transparency
Let users know how and why AI systems work.
2. Fairness
Avoid discrimination based on race, gender, or background.
3. Privacy
Protect user data and ensure it’s not used without consent.
4. Accountability
Assign clear responsibility for AI decisions and outcomes.
5. Reliability
Ensure the system performs safely under all conditions.
These values are the foundation of ethical AI development.
Implementing Generative Systems and Responsible AI Guidelines
To follow AI and generative systems guidelines, here are steps developers and organizations can take:
Create an AI Ethics Policy
This policy should define acceptable use cases, data handling, and auditing processes.
Monitor Outputs Regularly
Use human oversight to catch and fix risky or harmful content.
Provide Opt-Out Options
Give users control over how AI interacts with their data.
Train Teams on AI Ethics
Ensure all stakeholders understand the principles and consequences.
For more tips, check out Microsoft’s Responsible AI Resource Center.
Best Practices for Following Generative Systems and Responsible AI Guidelines
Generative Systems and Responsible AI Guidelines are most effective when integrated into the AI lifecycle. Here’s how:
During Design
-
Include diverse datasets
-
Document design goals
During Development
-
Use explainable AI models
-
Test for fairness and accuracy
After Deployment
-
Log AI actions and decisions
-
Update models as laws or standards change
These practices help build a sustainable and trustworthy AI ecosystem.
Real-World Examples of Generative Systems and Responsible AI Guidelines
ChatGPT by OpenAI
Follows strong safety checks and user feedback loops.
Google Bard
Implements user warnings and restricted content generation.
Adobe Firefly
Only trains models on licensed or public domain images.
These tools all reflect AI and generative systems guidelines in action.
See How AI Data Visualization Simplifies User Interfaces.
FAQs
What are Generative Systems?
AI models that create content like text, images, or code.
Why are Responsible AI Guidelines important?
They reduce risks, ensure fairness, and meet legal requirements.
Who sets these guidelines?
Tech companies, governments, and organizations like the OECD and UNESCO.
Are there any laws for AI use?
Yes, regulations like the EU AI Act and U.S. AI Bill of Rights are shaping how AI is governed.
The Future of AI with Generative Systems and Responsible AI Guidelines
The future of AI depends on how responsibly we develop it. AI and generative systems guidelines are essential to creating safe, fair, and useful technologies.
By understanding and applying these principles, businesses can innovate without compromising user safety or trust.
Author Profile
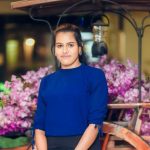
- Online Media & PR Strategist
- Hello there! I'm Online Media & PR Strategist at NeticSpace | Passionate Journalist, Blogger, and SEO Specialist
Latest entries
Data AnalyticsJune 13, 2025Future of Data Warehousing in Big Data
AI InterfaceJune 13, 2025Aligning AI Developments with Corporate Goals in the AI Era
HPC and AIJune 13, 2025HPC Architecture Taking to the Next Level
Quantum ComputingJune 13, 2025Ethical Issues in Quantum Tech: Privacy, Jobs, and Policy