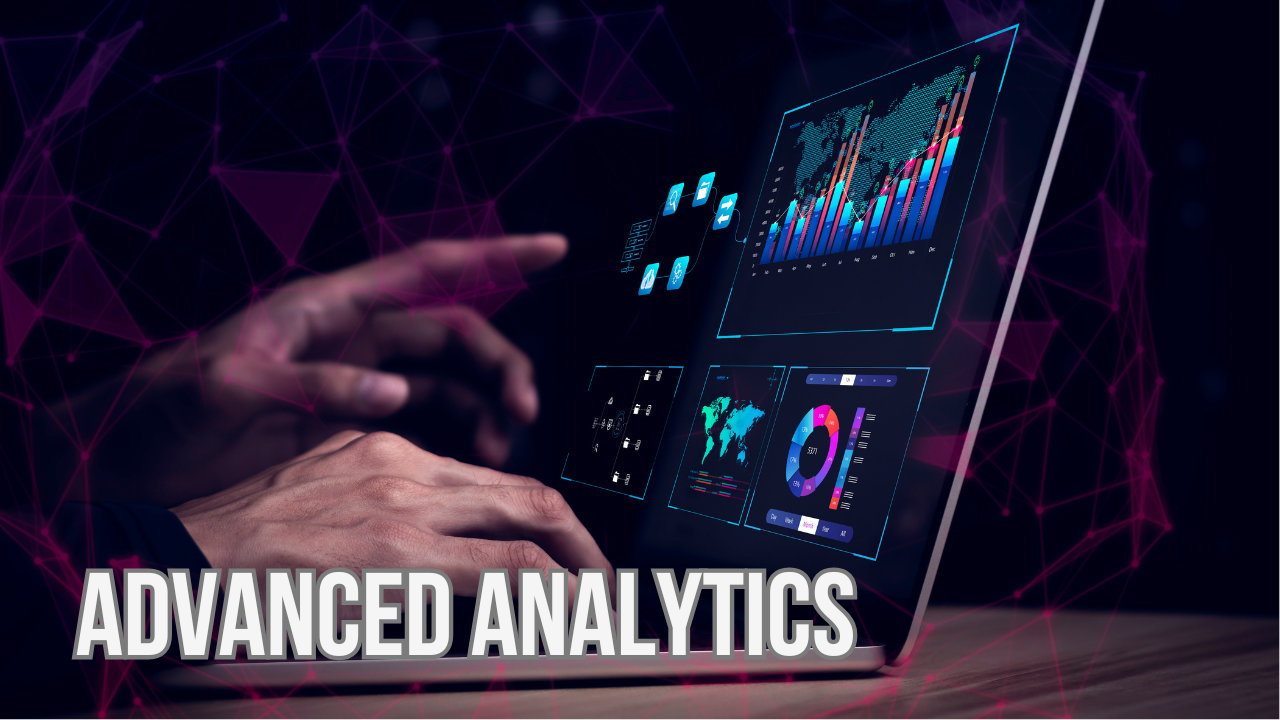
Advanced Analytics
Advanced analytics helps us turn raw data into meaningful insights. It goes beyond simple reporting. It uncovers trends, patterns, and opportunities hidden in big data. In this post, you will learn what advanced analytics is, why it matters, and how it can transform your decision-making.
First, we’ll define advanced analytics and highlight its difference from basic business intelligence. Next, we’ll look at the benefits it brings to businesses of all sizes. Finally, we’ll explore real-world use cases, best practices for implementation, and tips on choosing the right tools.
What Is Advanced Analytics?
Advanced analytics uses complex methods to extract deeper insights from data. It often involves predictive and prescriptive techniques. It goes far beyond standard reporting and dashboards. This includes analyzing past behavior, forecasting future events, and recommending best actions.
Significance in Today’s Data-Driven World
- Better Forecasting: Predict future trends with more accuracy.
- Risk Reduction: Identify potential problems early on.
- Efficiency Gains: Streamline processes through data-backed decisions.
Traditional business intelligence focuses on describing what happened in the past. Advanced analytics explains why it happened and what will happen next. This future-oriented approach helps organizations stay competitive in changing markets.
Benefits of Implementing Advanced Analytics
Organizations see many benefits when they embrace advanced analytics. Here are a few ways it can help:
- Improved Decision-Making
Leaders make choices based on data, not guesswork. This minimizes errors and boosts confidence. - Enhanced Forecasting
Advanced analytics uses predictive models to project likely outcomes. This helps teams plan resources and budgets effectively. - Risk Mitigation
By spotting problems early, businesses can avoid costly mistakes. They can also act quickly to fix them. - Problem Anticipation
Some tools go beyond prediction to suggest the best response. This gives companies a roadmap for tackling potential issues.
Real-World Examples
- Retail: Personalized product recommendations that boost sales.
- Finance: Fraud detection algorithms that spot suspicious behavior.
- Healthcare: Early disease prediction to guide preventive care.
Understanding Advanced Analytics Techniques
Types of Advanced Analytics
- Cluster Analytics
Groups data points based on similar traits.
Identifies natural segments or groups within large datasets. - Cohort Analytics
Examines how a group behaves over time.
Useful for analyzing customer retention or engagement. - Predictive Analytics
Uses past data to forecast future outcomes.
Often relies on machine learning to produce accurate predictions. - Prescriptive Analytics
Goes a step further by recommending actions.
Optimizes results by suggesting the most effective approach.
Key Techniques and Technologies
- Data Mining
Uncovers patterns and relationships in large datasets.
Helps businesses see hidden trends or outliers. - Machine Learning
Allows systems to learn from data without direct programming.
Automates tasks like classification, regression, and clustering. - Statistical Analysis
Uses statistical methods to interpret and validate data.
Helps confirm whether findings are significant or just random. - Text Analytics
Extracts meaning from unstructured text, like emails or social media posts.
Identifies sentiment, keywords, and emerging themes. - Big Data Analytics
Handles massive and diverse datasets.
Uses distributed computing tools like Apache Spark or Hadoop. - Other Useful Techniques
- Sentiment Analysis: Gauges public opinion about brands or topics.
- Complex Event Processing: Reacts to events in real time.
- Time Series Analysis: Examines data points collected over time.
- Data Visualization: Creates charts and graphs to present insights clearly.
Advanced Analytics in Action: Use Cases
Industry Applications of Advanced Analytics
- Healthcare
- Disease prediction to identify at-risk patients.
- Personalized treatment using patient data for tailored plans.
- Hospital operations optimization for better resource use.
- Finance
- Fraud detection with machine learning models.
- Risk management through credit scoring and market analysis.
- Customer segmentation to personalize banking services.
- Retail
- Demand forecasting to maintain optimal stock levels.
- Pricing optimization based on competitor and customer data.
- Supply chain management to reduce delivery delays.
- Manufacturing
- Predictive maintenance to fix machines before breakdowns.
- Quality control to identify defects in production lines.
- Production optimization for higher efficiency.
- Other Industries
- Transportation: Route optimization to reduce travel times.
- Energy: Smart grid analytics for resource distribution.
- Telecommunications: Network analytics for fault detection.
Real-World Success Stories
- Airline Pricing: Some airlines use predictive models to adjust ticket prices in real time. They factor in demand, seasonality, and competitor rates, leading to higher revenue.
- E-Commerce Personalization: Online stores often suggest products based on browsing and purchase history. Personalized experiences can boost sales and customer satisfaction.
- City Traffic Management: Some cities collect traffic data to analyze congestion patterns. They use these insights to optimize traffic signals and reduce commute times.
Implementing Advanced Analytics: A Guide
Building Your Advanced Analytics Strategy
- Define Business Objectives
Align analytics goals with company strategy.
Identify key performance indicators (KPIs) to track progress. - Data Assessment and Preparation
Collect, clean, and transform data for better accuracy.
Remove duplicates and fill data gaps to ensure consistent results. - Technology Selection
Evaluate tools that fit your needs and budget.
Consider factors like data volume, complexity, and existing infrastructure.
Building Your Team
Data experts drive successful analytics projects. Skilled professionals in data science, data engineering, and statistics are crucial. Existing staff may need training to work with new tools or methods. If needed, hire or partner with specialists who have experience in advanced analytics.
Fostering a Data-Driven Culture
A data-driven culture values facts over gut feelings. This requires management support and continuous learning. Everyone should understand basic data concepts and trust analytics results. Encourage employees to use data in everyday decision-making.
Advanced Analytics Tools and Platforms
Open-Source Tools
- R
- Great for statistical analysis and data visualization.
- Large library of packages for every industry need.
- Python
- Popular for machine learning and AI projects.
- Has rich libraries like TensorFlow, PyTorch, and scikit-learn.
- Apache Spark
- Handles big data processing at scale.
- Offers libraries for SQL, machine learning, and graph analytics.
Proprietary Tools
- SAS
- Known for its powerful analytics capabilities.
- Offers solutions for data mining and predictive modeling.
- IBM Cognos
- Provides enterprise reporting and dashboards.
- Integrates well with other IBM products.
- Microsoft Azure Machine Learning
- Delivers cloud-based machine learning services.
- Connects with other Azure services for data storage and processing.
Selection Criteria
- Scalability: How well does the tool handle growing data?
- Ease of Use: Do you have the staff or skills to operate it?
- Integration: Does it fit your existing tech stack?
- Cost: Is the total cost of ownership within your budget?
These considerations help you pick solutions that match your goals. Conduct proof-of-concept trials if needed. This ensures the tool works with your unique data.
Conclusion
The Future of Advanced Analytics
Advanced analytics continues to evolve. New breakthroughs in machine learning and artificial intelligence will drive smarter models. Improved data quality and larger datasets will unlock deeper insights. We can also expect more user-friendly interfaces that reduce technical barriers.
Organizations that leverage advanced analytics can respond faster to changes in the market. This dynamic approach encourages innovation. As analytics becomes more widespread, companies must stay informed about industry updates. This ensures they keep a competitive edge.
Frequently Asked Questions (FAQs)
- What is the difference between advanced analytics and business intelligence?
Business intelligence focuses on reporting past data. Advanced analytics uses techniques like predictive and prescriptive modeling for future insights. - What are the most important skills for an advanced analytics professional?
Key skills include statistics, programming (Python or R), data engineering, and machine learning. Communication skills are also vital. - How do I get started with implementing advanced analytics in my organization?
Begin by defining clear objectives. Next, evaluate data readiness. Then choose suitable tools and build a skilled team. - What are some common challenges in adopting advanced analytics?
Major obstacles include poor data quality, lack of skilled personnel, and weak organizational support. Address these early for smoother adoption. - What are the latest trends in advanced analytics?
Current trends involve cloud-based analytics, real-time data processing, automated machine learning, and deeper integration with AI.
Are you ready to unlock the potential of advanced analytics? Take the next step by exploring free online resources or connecting with industry experts. Consider our Advanced Analytics Services for hands-on support. Reach out today and transform your data into a powerful asset.
Author Profile
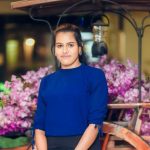
- Online Media & PR Strategist
- Hello there! I'm Online Media & PR Strategist at NeticSpace | Passionate Journalist, Blogger, and SEO Specialist
Latest entries
HPC and AIApril 30, 2025AI and HPC in Gaming: Realistic Virtual Worlds Today
Robotics SimulationApril 30, 2025How Robotics Simulation Agriculture Is Changing Farming
VirtualizationApril 30, 2025Future-Proof Virtualization Strategy for Emerging Tech
Simulation and ModelingApril 30, 2025Chaos Engineering: Build Resilient Systems with Chaos Monkey