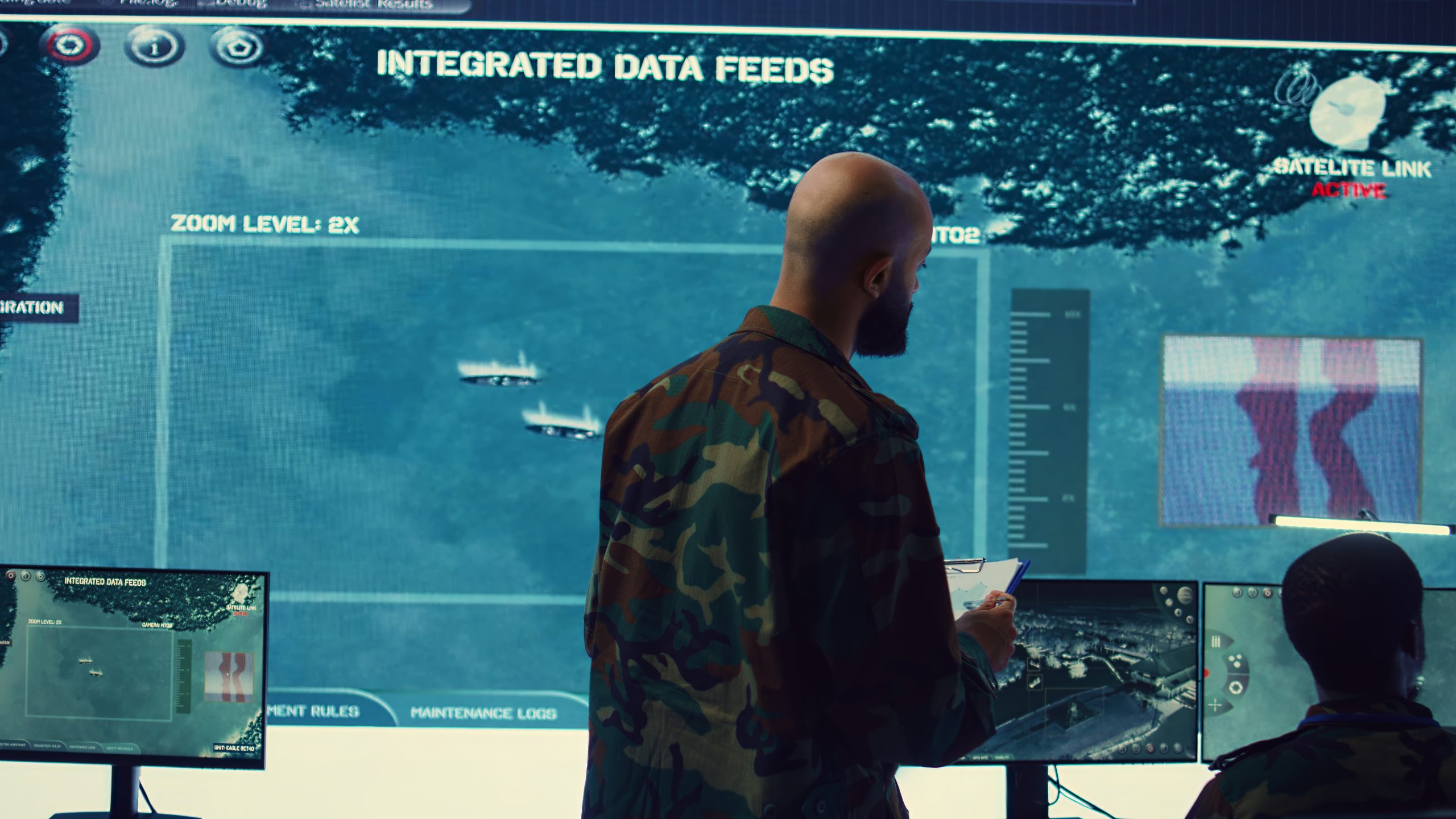
The Role of Big Data in Enhancing CAE Simulations
Big Data is changing how engineers run simulations. Modern CAE workflows rely on large datasets for accurate insights. This article explains how Big Data analytics fits into CAE simulation processes. You will learn how data-driven approaches improve decisions, reduce costs, and shorten product design cycles.
Why Big Data Matters for CAE Simulations
Big Data refers to the massive volumes of information generated every day. Engineering firms collect data from sensors, tests, and field reports. Handling this data in CAE simulations provides realistic models that reflect real-world conditions.
Shorter design cycles are a key benefit. Traditional simulations took days, or sometimes weeks, to run. With Big Data tools, engineers can process large amounts of data quickly. This speed means more accurate prototypes and faster time to market.
Factors Driving Big Data Integration
- Advanced Sensors: Modern equipment records detailed operating conditions.
- Connectivity: Connected devices let engineers gather data in real time.
- Data Storage: Cloud platforms store and manage large datasets economically.
These factors fuel the role of Big Data in enhancing CAE simulations for better decision-making.
How Big Data Analytics Integrates with CAE Workflows
First, data is collected from various sources. These include machine logs, historical performance records, and lab tests. Next, advanced analytics tools process this data to find patterns and trends.
Finally, engineers feed these insights into CAE software. This results in more accurate simulation models. They can predict stresses, temperature changes, or fluid behavior with greater precision. Data analytics also detects anomalies that might impact product safety.
Steps to Implement Big Data in CAE
- Data Collection
- Gather sensor data, test results, and production records.
- Data Cleaning
- Remove duplicates and outliers to ensure accuracy.
- Analytics Modeling
- Use machine learning or statistical methods to find trends.
- Simulation Input
- Incorporate insights into CAE tools for refined simulations.
This process ensures that CAE simulations remain grounded in real-world performance data.
Key Benefits of Using Big Data in CAE
Big Data offers several clear advantages. It sharpens models, cuts costs, and improves teamwork across departments. Here are some core benefits that highlight the role of Big Data in enhancing CAE simulations.
1. Improved Accuracy
Data-driven models mirror real-world behavior. This reduces the need for repeated physical testing. When simulations align with real conditions, developers can trust the results more.
2. Reduced Design Costs
Better accuracy means fewer prototypes. Engineers quickly spot weaknesses in a virtual environment. This lowers material and labor costs. It also shortens the time needed to move from concept to final product.
3. Faster Decision-Making
Teams can analyze performance results in near real time. Rapid insights help engineers respond to production changes or unexpected faults. In turn, managers can make informed choices on design tweaks and production plans.
4. Enhanced Collaboration
Sharing data between teams breaks down silos. Manufacturing, design, and quality departments can view the same data. This common ground fosters better communication and speeds up problem-solving.
Common Tools and Techniques for Big Data Analytics in CAE
Several technologies make it possible to process huge datasets. Cloud computing provides scalable servers that handle fluctuating workloads. On-premise solutions still exist, but cloud platforms are becoming more popular.
1. Distributed Computing
Big Data analytics often uses distributed systems like Hadoop or Spark. These tools split large tasks into smaller jobs. Engineers can then run them in parallel on multiple nodes. This boosts efficiency and cuts computation time.
2. Machine Learning Models
Statistical methods and machine learning algorithms help find hidden patterns. For example, anomaly detection can spot potential part failures. Predictive analytics can forecast product performance under different conditions.
3. Visualization Platforms
Tools like Tableau or Power BI transform raw data into charts and dashboards. Engineers see trends at a glance. This makes it easier to share findings with non-technical stakeholders.
Challenges and Best Practices
While Big Data in CAE simulations offers benefits, it also presents hurdles. Storage costs may surge if data is stored inefficiently. Data privacy and security rules need clear protocols.
Common Challenges
- Data Quality: Inconsistent data leads to flawed conclusions.
- Complexity: Handling many data types (images, logs, sensor readings) can be tricky.
- Skill Gaps: Teams may need specialized training in data analytics.
Best Practices
- Create Data Standards
- Ensure consistent formatting, naming, and labeling.
- Adopt Secure Storage
- Use encryption and access controls to protect valuable data.
- Invest in Training
- Train engineers and analysts on data tools.
- Prioritize Scalable Solutions
- Choose tools that can grow with your organization.
Conclusion
Big Data analytics is redefining CAE simulations. It delivers realistic results, speeds decision-making, and reduces costs. By collecting, cleaning, and analyzing large datasets, engineers gain a deeper understanding of product behavior. This leads to more dependable simulations and stronger products in the market.
When integrated properly, Big Data transforms CAE workflows from guesswork into data-driven insights. Your next step might be adopting tools like Hadoop, Spark, or cloud-based platforms. These solutions can help you unlock the full potential of engineering data. The path to better simulations starts with the right data strategy.
FAQ Section
1. What is Big Data in CAE simulations?
Big Data in CAE involves using large data sets from sensors, tests, and real-world operations. This data refines simulation models for improved accuracy.
2. How does Big Data improve decision-making in CAE?
It offers quick access to insights on component behavior. Engineers can spot design flaws, optimize production, and minimize risks.
3. What tools help manage Big Data for CAE?
Platforms like Hadoop or Spark handle distributed computing. Visualization tools like Tableau help translate complex data into understandable charts.
4. Are there security concerns with Big Data in CAE?
Yes. Large data sets can contain sensitive information. Best practices include encryption, restricted access, and regular audits.
5. Is Big Data necessary for all CAE projects?
Not always. Smaller projects with limited data may not need advanced analytics. However, complex designs benefit greatly from Big Data insights.
Author Profile
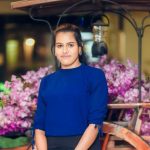
- Online Media & PR Strategist
- Hello there! I'm Online Media & PR Strategist at NeticSpace | Passionate Journalist, Blogger, and SEO Specialist
Latest entries
HPC and AIApril 30, 2025AI and HPC in Gaming: Realistic Virtual Worlds Today
Robotics SimulationApril 30, 2025How Robotics Simulation Agriculture Is Changing Farming
VirtualizationApril 30, 2025Future-Proof Virtualization Strategy for Emerging Tech
Simulation and ModelingApril 30, 2025Chaos Engineering: Build Resilient Systems with Chaos Monkey