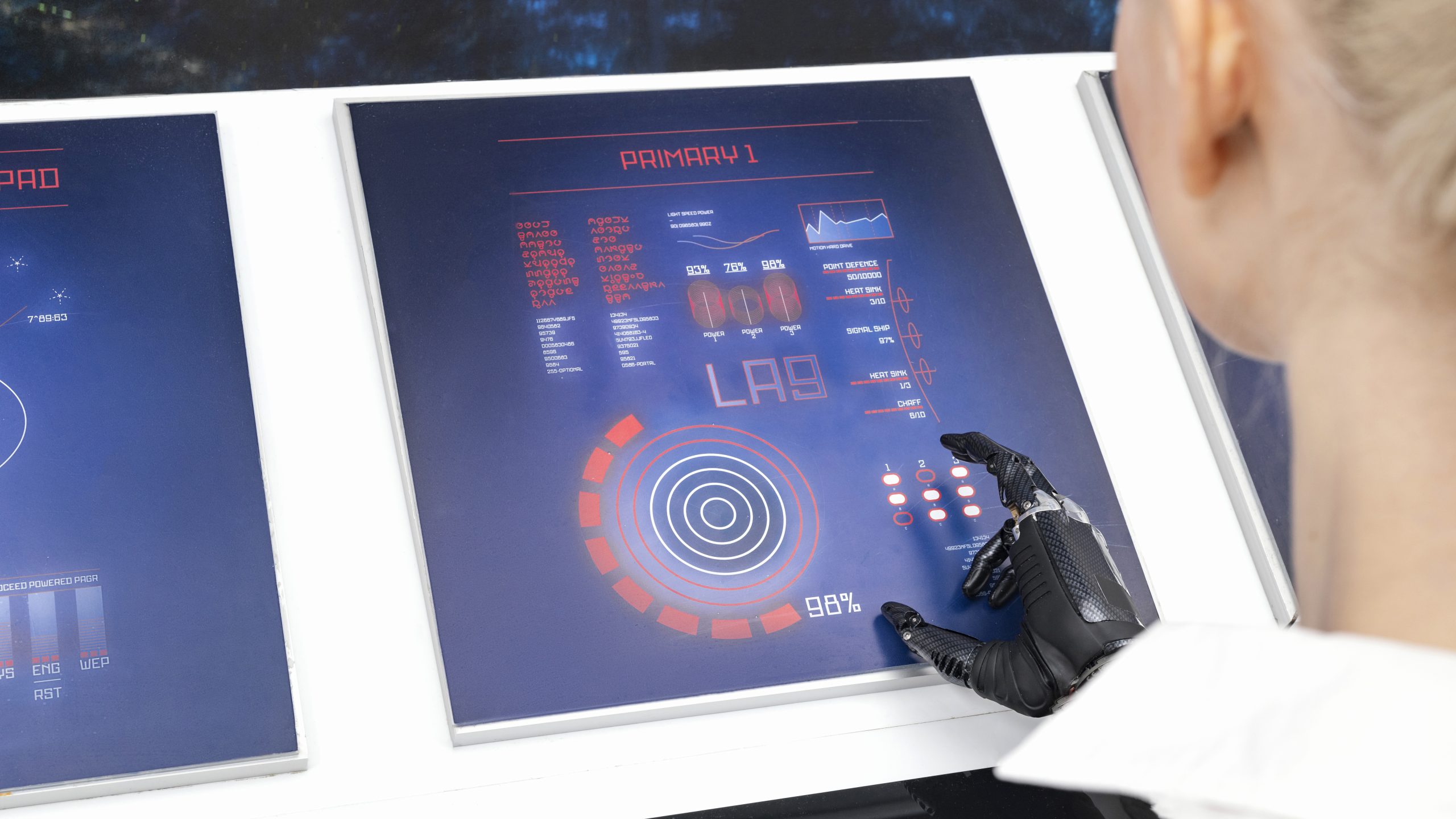
Simulation Accuracy & Adaptability Using AI in Robotics
Artificial intelligence (AI) is transforming how robots learn and function. It brings better accuracy and stronger adaptability. If you want to know how AI powers robotics simulations, you are in the right place. In this blog, you will learn how AI shapes the future of robotics.
Our goal is to provide educational content that drives traffic and generates leads for IT solutions. We will discuss the growing importance of AI in robotics simulations. You will discover real-world use cases, challenges, and future outlooks.
Understanding AI in Robotics Simulations
AI in robotics simulations helps create virtual environments where robots can learn. These environments mimic real-world conditions without risking physical damage. AI models process data to help robots make better decisions and react faster. This allows developers to refine robot behavior before real-world deployment.
Key Benefits of Simulations
- Cost Savings: Fewer physical prototypes save money and time.
- Safety: Tests happen in virtual settings, reducing accidents.
- Scalability: Developers can run multiple simulations at once.
- Efficiency: Simulations speed up training cycles and updates.
AI enhances each of these benefits. It uses data to predict outcomes with higher accuracy. This leads to better robots that adapt to changing needs.
How AI Enhances Accuracy in Robotics Simulations
Advanced Data Analysis
First, AI algorithms analyze large sets of simulation data. They detect patterns that might go unnoticed by human eyes. This helps robots fine-tune their movements and actions.
Next, machine learning refines decision-making. Robots learn from mistakes without causing real-world harm. The result is precise and efficient motion control.
Predictive Modeling
AI-driven predictive models forecast how a robot might behave. They consider different variables like speed, friction, or weight. By predicting outcomes, engineers can make tweaks early in the design phase.
Finally, these predictions lead to safer and more effective robots. When the design hits the real world, fewer surprises occur. This means less downtime and more reliable performance.
How AI Improves Adaptability in Robotics Simulations
Machine Learning for Real-Time Adjustments
Robots need to adapt on the fly. AI-powered simulations teach them to adjust when the environment changes. For example, a warehouse robot might face a sudden obstacle. Machine learning algorithms guide it to find a new path right away.
This adaptability is key to success in dynamic environments. AI ensures robots do not freeze or fail when challenges pop up. Instead, they keep working with minimal human input.
Reinforcement Learning for Continuous Improvement
Reinforcement learning is a branch of AI that rewards correct actions. In a simulated environment, each success triggers a positive reward. Each failure triggers a correction or penalty.
This cycle repeats until the robot optimizes its behavior. The goal is to create a self-improving system. As a result, robots evolve to handle complex tasks. Over time, they learn faster and become more resourceful.
Practical Applications of AI in Enhancing Robotics Simulations
Autonomous Vehicles
Robotic cars and drones rely on AI for safe navigation. Simulations let them practice in various road or sky conditions. They learn to avoid collisions and obey rules of travel.
Industrial Automation
In factories, robots often perform repetitive tasks. AI makes these tasks smarter and reduces errors. Simulations help test new production layouts before changes reach the assembly line.
Healthcare Robotics
Robotic surgeries are now more precise thanks to AI. Surgeons can simulate complex procedures for training and planning. This increases patient safety and promotes better outcomes.
Space Exploration
Robots sent to Mars or the Moon need to handle extreme environments. AI-driven simulations prepare them for low gravity, dusty surfaces, and temperature swings. This ensures missions go smoothly with minimal risk.
Challenges and Future Outlook
Limited Data and Complex Models
Developers often struggle to gather real-world data. Complex AI models require massive amounts of information. In some cases, data scarcity hinders model accuracy. Synthetic data can fill the gap, but it is not always perfect.
Ethical and Legal Issues
As AI takes a bigger role, ethical concerns grow. What happens if a robot malfunctions and hurts someone? Who is responsible for the AI’s decisions? These questions require clear laws and guidelines.
Future of Robotics Simulations
Despite challenges, the future looks bright. Advancements in hardware and software will improve AI accuracy. We will see more self-learning robots that can handle diverse tasks. Simulations will become even more realistic and immersive.
Best Practices for Implementing AI in Robotics Simulations
-
Start Small
Begin with simple AI tasks. Validate in a controlled virtual environment. -
Use Quality Data
Gather accurate data from sensors and real-world tests. This ensures better AI predictions. -
Iterate Quickly
Make changes fast and run simulations often. Rapid feedback loops yield faster improvements. -
Maintain Security
Protect simulation platforms from cyber threats. Breaches can harm robots and data integrity. -
Plan for Scalability
As AI grows, so will your simulation needs. Choose tools that can expand with your requirements.
Conclusion
AI in robotics simulations is changing how we design and train machines. It boosts accuracy through predictive modeling. It also builds adaptability through real-time learning. From autonomous vehicles to healthcare robots, AI has a massive impact.
By exploring AI-driven simulations, businesses can save time and money. They also reduce risk and improve performance. If you aim to stay ahead in robotics, AI is no longer optional. It is the key to better, smarter, and safer robot systems.
FAQ: Common Questions on AI in Robotics Simulations
1. How does AI improve the accuracy of robotic simulations?
AI analyzes large data sets to spot patterns and errors. This helps robots learn precise actions. It then refines these actions through machine learning models.
2. Is it expensive to integrate AI into simulations?
Costs vary, but starting small can keep budgets in check. Cloud-based tools and open-source frameworks also help reduce expenses.
3. Can AI help robots adapt to new tasks?
Yes. AI uses real-time adjustments and reinforcement learning. These methods teach robots to learn from new challenges and shift strategies on the fly.
4. What types of industries benefit most from AI in robotics simulations?
Industries like manufacturing, healthcare, transportation, and space exploration see major gains. Any field that values safety and efficiency can benefit.
5. How do we handle security concerns in AI-driven robotics?
Implement strict data protection and secure coding practices. Regular audits also help you identify and fix vulnerabilities quickly.
Author Profile
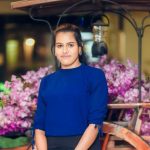
- Online Media & PR Strategist
- Hello there! I'm Online Media & PR Strategist at NeticSpace | Passionate Journalist, Blogger, and SEO Specialist
Latest entries
Robotics SimulationApril 30, 2025How Robotics Simulation Agriculture Is Changing Farming
VirtualizationApril 30, 2025Future-Proof Virtualization Strategy for Emerging Tech
Simulation and ModelingApril 30, 2025Chaos Engineering: Build Resilient Systems with Chaos Monkey
Digital Twin DevelopmentApril 30, 2025How to Ensure Data Synchronization Twins Effectively