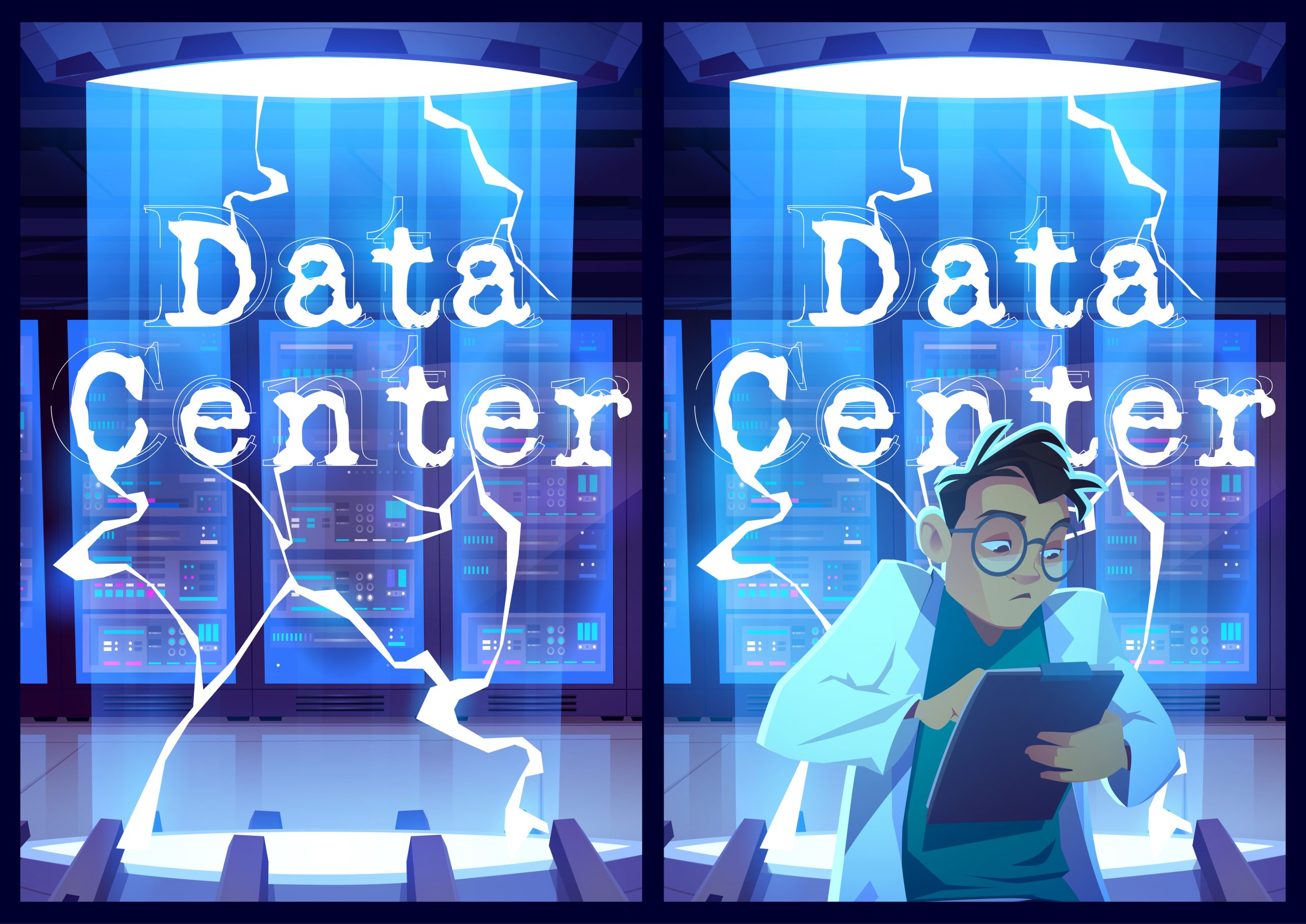
How to Transition from a Data Analyst to a Data Scientist
The purpose of this article is to drive traffic, generate leads, and provide educational content. You will learn the practical steps needed to move from a data analyst role to a data scientist position. This guide will help you gain new skills, build a strong portfolio, and succeed in the data science field.
Data science is one of the fastest-growing careers today. Many data analysts aspire to transition to a data scientist role for better opportunities. This article will show you the essential skills, resources, and steps for making that leap.
Why Transition from Data Analyst to Data Scientist?
Data analysts often work with structured data to create reports and insights. However, data scientists delve deeper to build predictive models and uncover patterns. Understanding why you want to transition from a data analyst to a data scientist is key.
First, data scientists usually handle more complex tasks. They explore unstructured data and design advanced solutions. Next, they apply machine learning algorithms to predict future trends. This combination of math, coding, and problem-solving leads to innovations that drive business growth.
Finally, a data scientist role often brings higher salaries and more impact. With specialized skills, you can stand out in the job market. This advantage can lead to leadership opportunities in the field.
Develop the Right Skills to Transition to Data Scientist
Programming Languages
To transition from a data analyst to a data scientist, you should learn programming languages like Python or R. These languages are vital for data manipulation, model building, and automation. Start with Python if you want a simpler syntax and a vast community.
Statistical Knowledge
Statistical analysis is a core component of data science. You should master concepts like hypothesis testing, regression, and Bayesian statistics. This foundation helps you interpret results and build reliable models.
Machine Learning Fundamentals
Understanding machine learning algorithms is essential for a data scientist role. Study supervised and unsupervised learning techniques. Learn to implement algorithms such as linear regression, decision trees, and random forests. Practice on real-world datasets to solidify your knowledge.
Gain Hands-On Experience in Data Science
Personal Projects
Hands-on experience is the best way to refine your data scientist skills. Work on personal projects that involve data cleaning, feature engineering, and model selection. Showcase your findings in a clear, concise manner.
Kaggle Competitions
Kaggle is a popular platform for data science competitions. You can improve your skills by solving real problems with complex data. Competing also helps you learn best practices from peers and experienced professionals.
Online Courses and Certifications
Enroll in online courses that focus on data science and machine learning. Look for recognized certifications such as those offered by Coursera or edX. These programs teach industry-standard practices and keep you updated on new trends.
Build a Data Scientist Portfolio
A strong portfolio is crucial when transitioning from a data analyst to a data scientist. Employers want to see tangible results. Show that you can manage data pipelines, develop models, and communicate findings.
Showcase Your Projects
Include different types of projects that highlight your programming and analytical abilities. For example, projects on natural language processing or image classification demonstrate versatility. Explain the problems you solved and the tools you used.
Highlight Tools and Techniques
Make sure you list the libraries and frameworks you are comfortable with. Examples include Pandas, NumPy, scikit-learn, and TensorFlow. Emphasize how you used these tools to streamline data preprocessing and model building.
Networking and Mentorship for a Smooth Transition
Networking can speed up your transition from data analyst to data scientist. Attend local meetups or conferences to connect with like-minded professionals. Share your experiences, learn from others, and discover emerging trends.
Seek out a mentor with experience in data science. A good mentor can guide you, provide feedback, and help you avoid common mistakes. Mentorship often leads to opportunities that boost your career.
LinkedIn groups and online forums also offer valuable support. Ask questions, exchange ideas, and stay current on industry updates. Active participation builds credibility and helps you stand out.
FAQ: Transitioning from Data Analyst to Data Scientist
1. How long does it take to transition from a data analyst to a data scientist?
It depends on your current skills and how fast you learn. Some people make the move in six months, while others take a year or more. Consistent practice and targeted learning can speed up your progress.
2. Do I need a master’s degree to become a data scientist?
A master’s degree can be helpful but is not always required. Many successful data scientists learned through online courses, personal projects, and industry experience.
3. How important are math and statistics for data scientists?
They are very important. Statistics is the backbone of modeling and inference. A solid math foundation helps you understand algorithms and optimize results.
4. Which tools should I focus on to transition to a data scientist role?
Focus on Python or R for programming, SQL for database queries, and machine learning libraries like scikit-learn or TensorFlow. Choose tools that align with your project goals.
5. Can I transition from a data analyst to a data scientist if I have no machine learning experience?
Yes. Start by learning basic machine learning concepts, then apply them to simple projects. Gradually move on to more advanced techniques.
Conclusion
Transitioning from a data analyst to a data scientist is a rewarding process. You will build advanced technical skills, tackle challenging problems, and create more value for your organization. Focus on expanding your programming, statistics, and machine learning abilities. Gain real-world experience through personal projects, competitions, and online courses. With a solid portfolio and strong network, you can stand out in the data science job market.
Remember, every step you take helps you grow. Follow a structured plan, keep learning, and never hesitate to ask for guidance. The data science world is waiting for your insights and expertise.
Author Profile
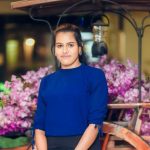
- Online Media & PR Strategist
- Hello there! I'm Online Media & PR Strategist at NeticSpace | Passionate Journalist, Blogger, and SEO Specialist
Latest entries
VirtualizationApril 30, 2025Future-Proof Virtualization Strategy for Emerging Tech
Simulation and ModelingApril 30, 2025Chaos Engineering: Build Resilient Systems with Chaos Monkey
Digital Twin DevelopmentApril 30, 2025How to Ensure Data Synchronization Twins Effectively
Scientific VisualizationApril 30, 2025Deepfake Scientific Data: AI-Generated Fraud in Research