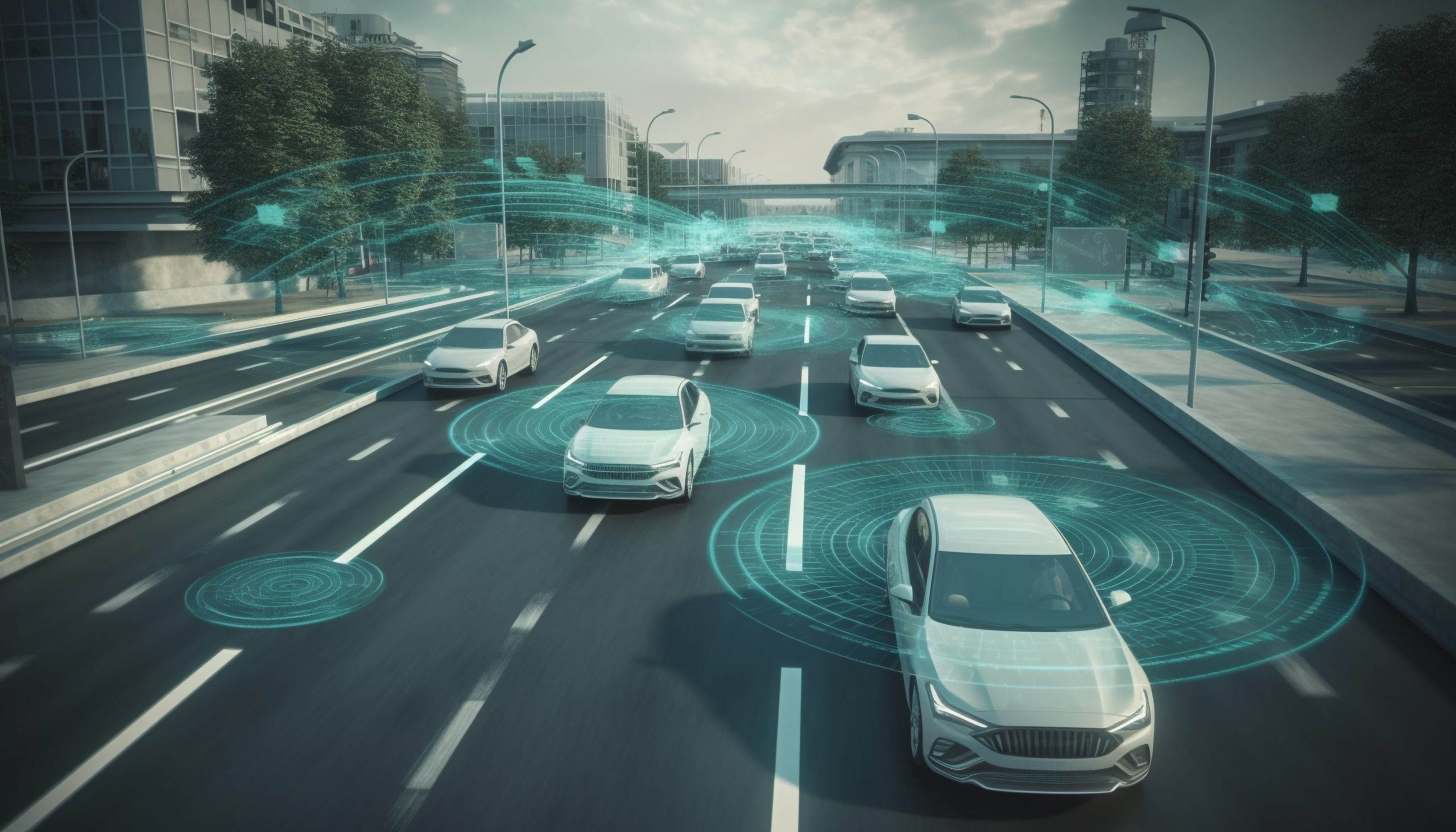
The Challenges of Simulating Real-World Traffic for Smart Cities
Simulating real-world traffic is not simple. Modern cities are complex environments. Traffic involves cars, buses, bikes, and pedestrians. Planners need accurate models to shape smarter urban development.
In this blog post, you will learn about the challenges of simulating traffic for smart cities. You will also discover solutions that drive effective urban planning. Our purpose is to provide educational content, drive traffic, and generate leads for those interested in smart city development.
Understanding Why Real-World Traffic Matters in Smart City Development
Accurate traffic simulations are essential for city growth. They help predict congestion and guide better infrastructure investments. They also show how new policies affect road use.
These simulations form the base of smart city planning. First, they reveal the best ways to allocate budget for roads and transit systems. Next, they highlight where footpaths, bike lanes, and parking spaces are most needed.
1. Accurate Congestion Forecasts
Congestion affects commute times, air quality, and public safety. Predicting congestion hotspots is key for smart city planners. Without proper estimates, solutions might fail when it matters most.
2. Improved Public Transportation
City officials often test new bus routes and train schedules. Traffic simulations give them a low-risk way to see potential outcomes. This helps prevent wasteful spending on projects that won’t work.
Key Challenges in Simulating Real-World Traffic for Urban Planning
Accurate traffic models require more than basic equations. Real cities involve unpredictable elements. The process needs advanced tools and high-quality data.
These challenges affect decision-making in smart city projects. Next, we’ll explore the most common obstacles and ways to address them.
1. Data Collection and Validation
Traffic data comes from sensors, cameras, GPS devices, and surveys. Gathering this data can be expensive and time-consuming. Even after collection, the data might be incomplete.
Validating data is equally tough. Officials must ensure accuracy by comparing multiple sources. If the data is flawed, simulations can lead to poor decisions.
2. Modeling Human Behavior
Drivers and pedestrians act in unpredictable ways. This makes it hard to replicate real-world traffic scenarios. People may change routes based on factors like accidents or bad weather.
Behavioral models must account for these quick decisions. Small errors in assumptions can throw off the entire simulation. As a result, capturing human unpredictability remains a major hurdle.
3. Integrating Multiple Modes of Transport
Smart cities promote cycling, walking, and public transit. A robust traffic simulation includes all these modes. Mixing cars and bikes on the same road can lead to complex patterns.
City planners must also model parking demand, ride-sharing, and micro-mobility like e-scooters. These elements add more layers to simulations. Missing any one factor can reduce the model’s overall accuracy.
Tools and Techniques for Effective Traffic Simulations
Despite these obstacles, many tools help tackle the challenges of simulating real-world traffic scenarios for smart cities. Advanced software platforms combine data analysis with predictive modeling. They offer a glimpse into different planning outcomes.
Below are proven methods to improve simulation accuracy. Finally, these approaches support urban planners in making better decisions.
1. Agent-Based Modeling
Agent-based models simulate individual “agents.” Each agent follows specific rules. These agents interact and influence each other in real-time.
This method reflects human behavior more accurately. For example, one driver might choose a different route when seeing a traffic jam ahead. Agent-based modeling can capture these changes in detail.
2. Machine Learning Enhancements
Machine learning can find hidden patterns in large datasets. City planners use these insights to improve predictions. By training models on historical traffic data, forecasts become more precise.
However, machine learning models must be trained carefully. Planners need reliable data sources that represent various conditions. Otherwise, algorithms might produce biased or incomplete results.
3. Real-Time Data Integration
Real-time data offers up-to-the-minute updates on road conditions. Traffic monitoring tools can feed simulations with live sensor information. This shows how city networks respond to sudden events.
Examples include:
- Accidents: Detours and lane closures
- Weather changes: Rain or snow impacting speeds
- Public events: Concerts causing temporary congestion
Integrating this data makes simulations more dynamic. This helps city planners make quick adjustments when conditions change.
Strategies to Overcome Smart City Traffic Simulation Challenges
Smart city projects can succeed with proper planning and teamwork. Overcoming simulation challenges is easier when stakeholders cooperate. Below are ways to address these obstacles head-on.
1. Collaborative Partnerships
Partnerships between governments, tech firms, and researchers improve data sharing. Each group brings specialized knowledge. Governments have access to planning documents, while tech firms offer analytics platforms.
Researchers often contribute new modeling techniques. Sharing resources reduces costs and avoids data silos. This leads to more accurate simulations that benefit everyone.
2. Continuous Feedback Loops
Traffic conditions evolve as cities grow. Creating a feedback loop allows planners to update simulations frequently. This ensures the models stay relevant over time.
For example, city officials might compare real-world sensor readings with simulation outputs. Any mismatch reveals potential flaws. They then refine the model to fix these errors.
3. Pilot Programs and Testbeds
Small-scale projects let planners test solutions before citywide adoption. Using a limited area or district helps identify problems early. This reduces risks and cost overruns.
For instance, a pilot program might focus on one busy intersection. By monitoring outcomes, planners see how well the model matches reality. Successful testbeds can then expand to the broader city.
Conclusion
Simulating real-world traffic for smart cities is challenging. Planners must deal with messy data, human unpredictability, and multiple transport modes. These factors complicate the quest for precise and reliable models.
Yet, solutions are emerging. Tools like agent-based modeling, machine learning, and real-time data integration show promise. Collaboration, continuous feedback, and pilot programs can further refine these simulations. With the right strategies, cities can design safer, more efficient networks for everyone.
Frequently Asked Questions
1. What is traffic simulation for smart cities?
Traffic simulation for smart cities uses computer models to mimic real-world road conditions. This helps officials plan roads, public transportation, and services.
2. Why is accurate traffic data so important?
Accurate data ensures reliable predictions. Incomplete or faulty data can lead to wrong conclusions and costly mistakes in urban planning.
3. How do simulations account for human behavior?
Some models use agent-based methods, where each driver or pedestrian is an individual agent. These agents make decisions based on set rules, which reflect real human actions.
4. Why integrate real-time data?
Real-time data shows current traffic conditions. This helps planners see immediate changes and predict how events like accidents or concerts will impact travel.
5. How can cities start using traffic simulations?
Many cities begin with small pilot programs. They gather data, test the model, and refine it before rolling out solutions on a citywide scale.
Author Profile
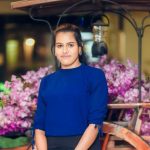
- Online Media & PR Strategist
- Hello there! I'm Online Media & PR Strategist at NeticSpace | Passionate Journalist, Blogger, and SEO Specialist
Latest entries
Artificial InteligenceApril 30, 2025Master Prompt Engineering Techniques for Better AI Output
HPC and AIApril 30, 2025AI and HPC in Gaming: Realistic Virtual Worlds Today
Robotics SimulationApril 30, 2025How Robotics Simulation Agriculture Is Changing Farming
VirtualizationApril 30, 2025Future-Proof Virtualization Strategy for Emerging Tech