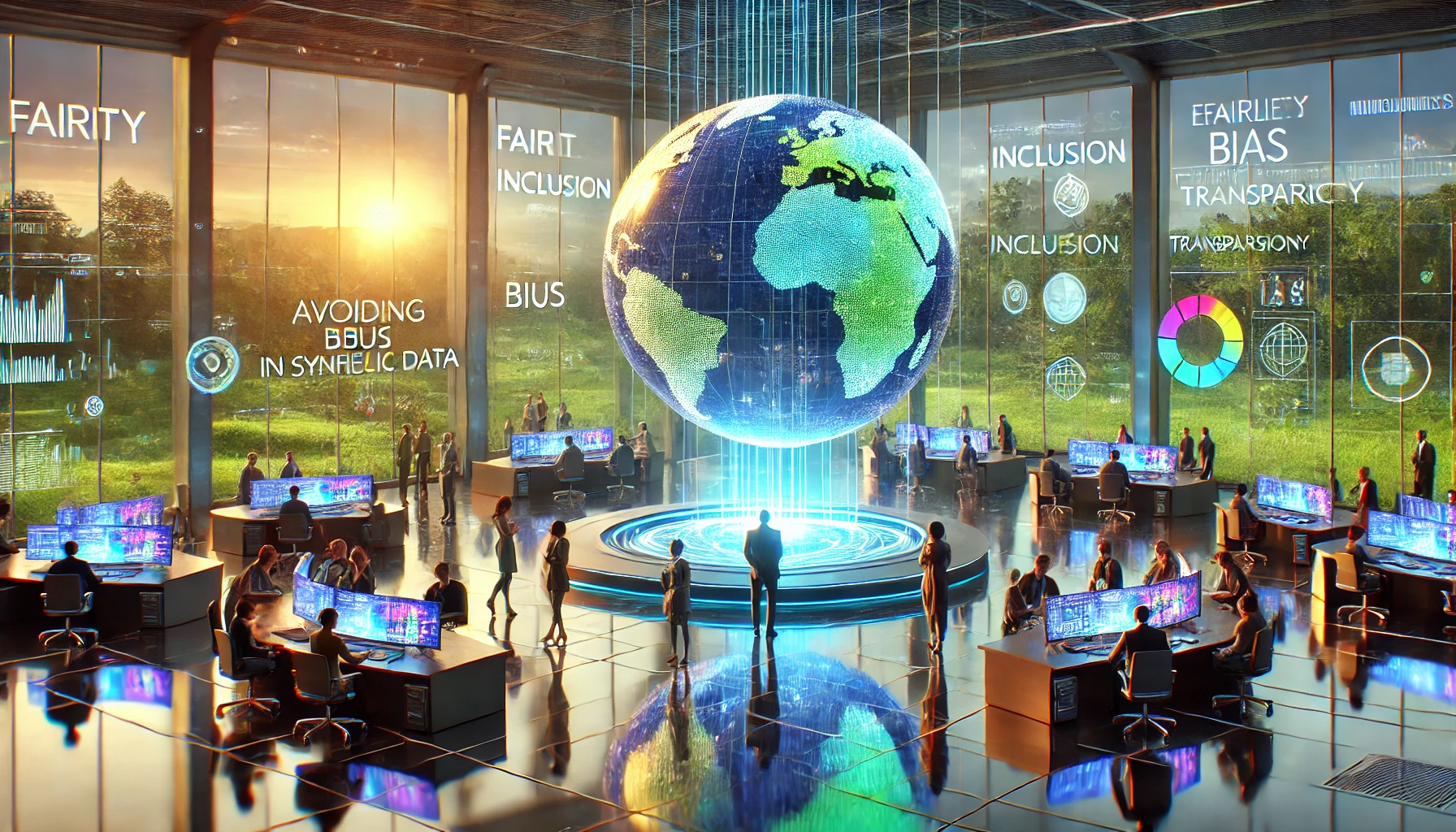
Making sure synthetic data is not biased
Synthetic data plays a big role in modern AI and machine learning. It can help protect privacy. It can also speed up data-driven projects. Yet, there’s a hidden challenge: bias can sneak into synthetic data, causing unfair outcomes.
In this post, you will learn how to recognize these risks. You will also discover strategies to prevent bias in synthetic data. By the end, you will have a clear plan to keep your data fair, transparent, and ethical.
Understanding Bias in Synthetic Data
Bias is not just a concern for real-world datasets. Synthetic data can also reflect unbalanced or skewed patterns. These patterns may come from the methods used to create the data or the original data itself.
What is Bias?
Bias is a systematic error that affects outcomes. In AI, biased data can lead to unfair decisions and incorrect predictions. For example, if synthetic data underrepresents a certain group, the AI model may perform poorly for that group.
How Bias Slips into Synthetic Data
1. Inherited Bias: If you train a generative model on biased real data, the resulting synthetic data may repeat those patterns.
2. Algorithmic Choices: The techniques or parameters used to create synthetic data can amplify hidden imbalances.
3. Limited Diversity: Failing to include a wide range of real-world scenarios can cause synthetic datasets to exclude important variations.
These issues highlight the importance of careful oversight. Even artificial data can mirror harmful stereotypes.
Types of Biases Relevant to Synthetic Data
Synthetic data generation might seem like it offers a blank slate. However, biases can creep in through several channels. Here are some common examples:
Sampling Bias
Sampling bias occurs when the synthetic data does not represent the true diversity of the real world. For instance, if your original dataset is mostly from one region, your synthetic data might reflect only that region’s patterns. This uneven distribution can lead to distorted insights.
Algorithmic Bias
Algorithmic bias arises from the way models are designed or trained. Some synthetic data generators rely on assumptions that may not hold for all populations. If those assumptions are flawed, the final data will reflect those flaws. The result is a distorted version of reality.
Modeling Bias
Modeling bias appears when the data generation process oversimplifies complex relationships. Imagine a system that merges multiple sources but overlooks vital nuances. The synthetic dataset may omit key features and produce one-size-fits-all patterns. This can hurt model performance in real-world tasks.
Mitigating Bias in Synthetic Data Generation
Preventing bias in synthetic data demands proactive steps. It’s not enough to trust the model to “figure it out.” Each stage in the pipeline needs oversight to ensure fairness.
Start with Diverse Training Data
Diversity is critical from day one. Gather input data that covers various demographics, geographies, and conditions. The more representative the initial dataset, the less likely synthetic data will exclude significant groups.
Employ Fairness-Aware Algorithms
Some models are built to reduce bias. These fairness-aware algorithms adjust sampling methods and correct known imbalances. They aim to create data that reflects real-world distributions without amplifying skew.
Use Pre-Processing Techniques
Consider balancing or re-sampling methods before generating synthetic data. These approaches help even out class imbalances. They also reduce the chance that synthetic data will overrepresent a majority class.
Rely on Post-Processing Adjustments
No generation process is perfect. After producing synthetic data, analyze it for signs of bias. If you spot an issue, adjust the final dataset. This extra layer of refinement can prevent unfair outcomes.
Emphasize Transparency and Explainability
Document each step you take in generating synthetic data. Make your methods clear, and share them with relevant stakeholders. Explainable synthetic data processes help others understand how biases might arise and how you tackled them.
The Role of Ethical Frameworks and Guidelines
Ethical considerations matter at every step of data science. Synthetic data is no exception. Guidelines help teams stay on track and maintain public trust.
Established Principles for Responsible AI
Privacy: Protect individual information by design.
Fairness: Strive to treat all groups equally.
Accountability: Be prepared to answer for any harm caused by data misuse.
Transparency: Share details about how data is collected, generated, and used.
Why Ethical Frameworks Matter
Frameworks set clear rules for behavior. They remind us that technology can affect society in major ways. By following well-known guidelines, teams can prove their commitment to ethical data practices.
Aligning Synthetic Data with Regulations
Some regions have strict data privacy laws. Synthetic data may fall under these regulations if it could still reveal sensitive information. Always review local rules before sharing or selling any generated dataset. This helps ensure compliance and maintains trust.
Case Studies: Synthetic Data and Ethical Successes
Real-life examples show how synthetic data can improve AI without harming vulnerable groups. Below are a few success stories that highlight best practices.
Healthcare Research Example
A hospital needed a patient dataset to test new treatment models. Instead of using real patient records, they created synthetic data that preserved general patterns. Privacy stayed intact, and researchers found no major biases in disease prevalence or outcomes. Their approach combined fairness-aware generation techniques with thorough post-processing checks.
Financial Services Example
A bank wanted to analyze loan applications without exposing real customer data. They used a diverse dataset of past applications and built a generator to produce synthetic samples. The team tracked potential biases by comparing real data metrics to synthetic data metrics. Adjustments were made to ensure loan approval rates did not skew unfairly by demographic.
Tech Startup Example
A startup developed a tool to detect fraud in e-commerce. They used synthetic data to train their machine learning models. They also tested for unbalanced patterns against different user types. This let them refine their system to avoid bias against new customers or small businesses. Through continuous updates and ethical frameworks, they maintained fairness at scale.
Future Trends and Challenges
The field of synthetic data is evolving fast. As technology grows more advanced, so do the ethical dilemmas.
More Realistic Synthetic Data
Generative models, like advanced deep learning networks, can create datasets that closely mimic reality. This realism is valuable but also dangerous. If synthetic data looks too real, it could unintentionally reveal identities or hidden patterns.
Constant Monitoring and Evaluation
Bias is not static. Social norms change, and so do data patterns. Teams need regular checks to catch new forms of bias. Automated tools can help track shifts in data distribution over time.
Better Bias Detection Techniques
Research into bias detection is ongoing. New methods will help identify subtle or emerging forms of skew. For instance, advanced analytics can measure disparities between synthetic and real data across various demographic splits.
Stricter Regulations
As synthetic data gains popularity, lawmakers may introduce stricter rules. This can include certification for ethical AI or mandatory bias audits. Staying ahead of these rules is key to maintaining trust and avoiding penalties.
Conclusion
Ethical considerations are essential when generating synthetic data. Even data that appears anonymous can still contain biases. These biases may harm certain groups, skew AI outcomes, or raise privacy concerns.
You can reduce these risks by using fairness-aware algorithms and thorough validation steps. Document each stage of your process, from the original dataset to final adjustments. This transparency supports accountability and fosters trust.
Above all, keep monitoring your synthetic data. Technology changes fast. So do patterns in real-world data. By staying vigilant and prioritizing fairness, you can ensure that synthetic data remains a positive force in AI development.
FAQs
1. What is the difference between real and synthetic data?
Real data comes from actual events, people, or sensors. Synthetic data is artificially generated to mimic real data patterns without exposing sensitive details.
2. How can I detect bias in my synthetic data?
Use statistical tests and metrics that compare different groups. Look for discrepancies in model performance or representation across demographics.
3. What are the most effective techniques for mitigating bias in synthetic data?
Combine fairness-aware algorithms with data balancing, post-processing adjustments, and transparency. Also, compare synthetic data distributions to real-world benchmarks.
4. Are there any regulations or standards regarding ethical synthetic data generation?
Regulations vary by region. Some data privacy laws may still apply if synthetic data can be linked back to real individuals. Keep an eye on evolving guidelines and industry best practices.
5. Where can I find resources to learn more about ethical AI development?
Many organizations publish free guidelines and research. Look for reputable sources like academic institutions, AI ethics committees, or professional societies in your field.
Author Profile
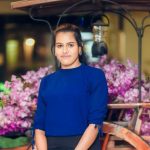
- Online Media & PR Strategist
- Hello there! I'm Online Media & PR Strategist at NeticSpace | Passionate Journalist, Blogger, and SEO Specialist
Latest entries
Artificial InteligenceApril 30, 2025Master Prompt Engineering Techniques for Better AI Output
HPC and AIApril 30, 2025AI and HPC in Gaming: Realistic Virtual Worlds Today
Robotics SimulationApril 30, 2025How Robotics Simulation Agriculture Is Changing Farming
VirtualizationApril 30, 2025Future-Proof Virtualization Strategy for Emerging Tech