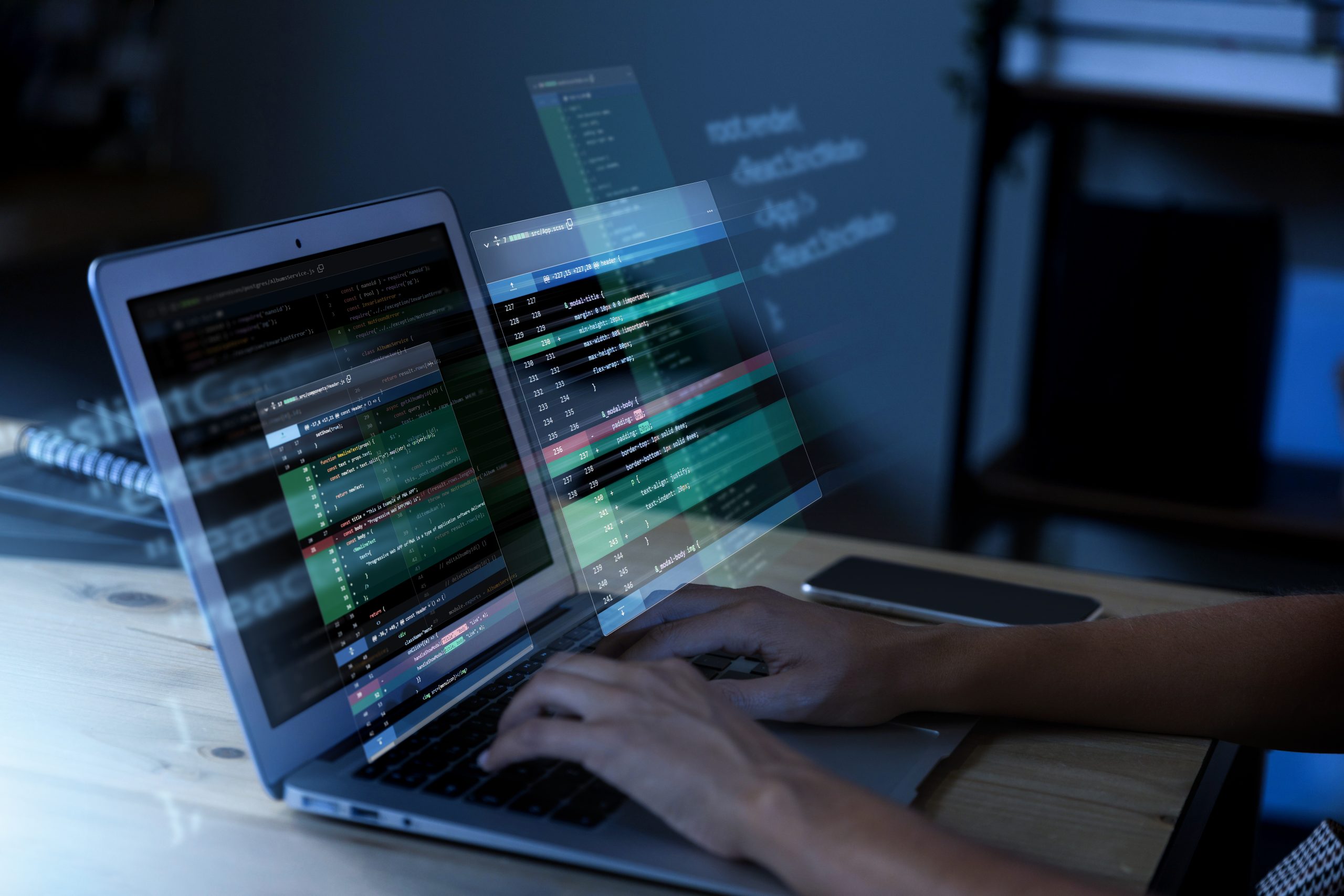
Deepfake Scientific Data: AI-Generated Fraud in Research
Scientific research is under a new kind of threat—deepfake scientific data.
AI tools now make it easy to create fake graphs, images, and simulations that can pass as real scientific results. This trend is causing serious concern in the research community.
In this article, you’ll learn what deepfake scientific data is, why it’s dangerous, how it spreads, and what can be done to stop it.
What Is Deepfake Scientific Data and Why It Matters
Deepfake scientific data is any research image, chart, or simulation created or altered using AI tools to mislead.
These visuals may look authentic, but they often distort or fabricate results. Unlike harmless AI-generated art, fake scientific visuals can cause real-world harm.
For example, a fake graph in a cancer study could affect treatment development. Similarly, a false simulation in an engineering paper might lead to unsafe product designs.
How Deepfake Scientific Data Spreads in Research
First, AI makes it easy to create realistic but false visuals. Tools like GANs (Generative Adversarial Networks) can generate data that looks legitimate.
Next, researchers who want to publish quickly—or commit fraud—may insert these fake visuals into papers.
Finally, peer reviewers may not catch the issue, especially when visuals appear complex or technical. As a result, deepfake scientific data gets published and spreads through citations.
Warning Signs of Deepfake Scientific Data
Visual Clues in Images and Graphs
-
Repeated patterns or elements
-
Inconsistent lighting or resolution
-
Odd background textures
Metadata Red Flags
-
Missing creation date or device info
-
Identical metadata across unrelated studies
-
Format changes that don’t match submission guidelines
Statistical Anomalies
-
Overly smooth data trends
-
Reused values across multiple experiments
-
Lack of random variation where expected
These signs often appear together in papers using deepfake.
How Experts Detect Deepfake Scientific Data Today
To protect research integrity, experts use several strategies.
AI-Powered Screening Tools
Tools like ImageTwin and Proofig scan thousands of research papers for reused or altered images. They flag high-risk visuals for further review.
Improved Peer Review Training
Many journals are training reviewers to look for visual inconsistencies. This includes spotting unnatural image edits or duplicate graphs.
Open Data Practices
When researchers share their raw data and code, it becomes easier to detect manipulation. This transparency discourages the use of deepfake.
Real Examples: When Fake Research Goes Too Far
In 2023, over 600 academic papers were retracted due to manipulated visuals. Many of these were traced back to deepfake scientific data.
For instance, a set of medical studies used identical images of tumors across unrelated research. These images were generated using AI and escaped initial review.
Another case involved physics simulations that never ran in reality. Instead, the visuals were designed by AI to support made-up results.
Preventing Future Deepfake Scientific Data Fraud
Strengthen Submission Standards
Journals are now requiring authors to submit original image files and explain how visuals were created.
Enforce Author Accountability
More publishers are asking for image licenses, researcher IDs (like ORCID), and conflict-of-interest disclosures.
Promote Ethical AI Use
Research institutions are starting to offer training on ethical data creation and the dangers of deepfake.
By applying these steps, the risk of AI-driven fraud can be reduced.
Why Research Integrity Depends on This Fight
Unchecked, deepfake can destroy the credibility of scientific literature.
Once fake results make it into textbooks or influence real-world decisions, the damage is hard to undo.
That’s why fighting this threat isn’t optional—it’s necessary for the future of trustworthy science.
FAQ
What is deepfake scientific data?
It’s AI-generated or altered research visuals designed to mislead or fabricate study results.
How can you detect deepfake scientific data?
By using tools like ImageTwin, checking metadata, and training peer reviewers to look for visual fraud.
What happens if deepfake data is published?
It may lead to retractions, loss of funding, and harmful decisions based on false results.
How can researchers protect their work?
By using original visuals, sharing raw data, and avoiding suspicious AI tools for image creation.
Why is this problem growing now?
AI tools are becoming more powerful and accessible, making fraud easier to commit and harder to detect.
Stay Alert, Stay Ethical
The rise of deepfake scientific data is a real and urgent threat.
However, with better tools, smarter review processes, and a commitment to ethical research, we can detect and prevent fraud before it spreads.
Everyone in the research community has a role to play—from authors to editors to readers. By staying informed, we defend the future of science. deepfakesweb.com
Author Profile
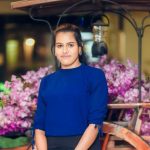
- Online Media & PR Strategist
- Hello there! I'm Online Media & PR Strategist at NeticSpace | Passionate Journalist, Blogger, and SEO Specialist
Latest entries
Data AnalyticsJune 13, 2025Future of Data Warehousing in Big Data
AI InterfaceJune 13, 2025Aligning AI Developments with Corporate Goals in the AI Era
HPC and AIJune 13, 2025HPC Architecture Taking to the Next Level
Quantum ComputingJune 13, 2025Ethical Issues in Quantum Tech: Privacy, Jobs, and Policy