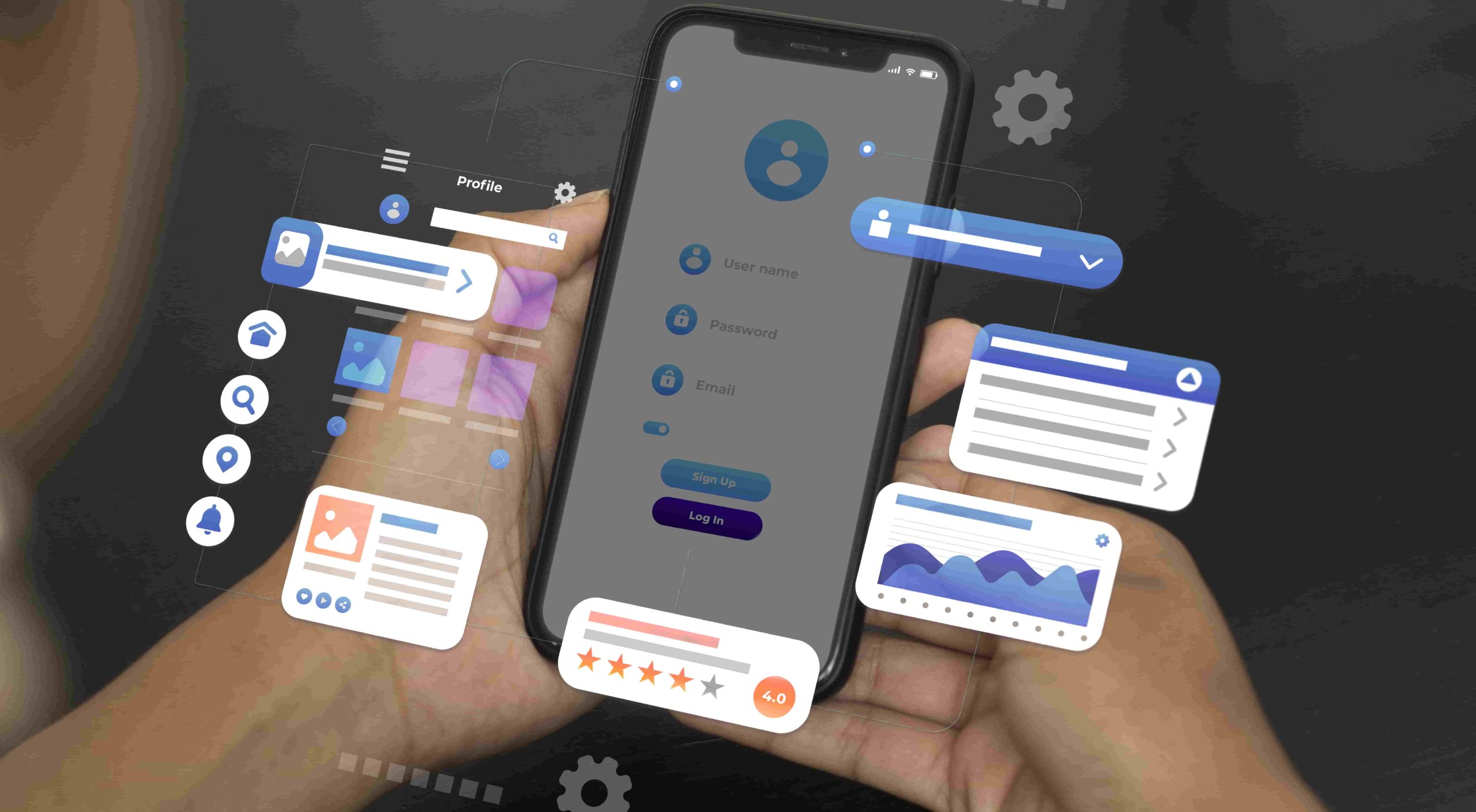
Smarter Apps Using IoT with MLOps Integration
The future of connected devices is not just smart — it’s intelligent. Integrating IoT with MLOps is transforming how we build, deploy, and maintain smart applications. In this blog, you’ll learn how combining IoT and MLOps creates efficient, scalable, and adaptive systems, especially in industries like manufacturing, healthcare, agriculture, and logistics.
We’ll break down what IoT MLOps means, how it works, benefits, real-world uses, and how you can apply it in your systems. Let’s get started.
What Is IoT with MLOps Integration?
IoT with MLOps refers to combining Internet of Things (IoT) devices with Machine Learning Operations (MLOps) workflows. This allows real-time data from sensors to be processed, analyzed, and turned into actionable insights using machine learning models — all in an automated and repeatable way.
Key Concepts:
-
IoT devices collect data through sensors and send it to cloud or edge platforms.
-
MLOps manages the end-to-end lifecycle of ML models — from training to deployment.
-
Combined, they enable smart applications that learn and improve over time.
Why Use IoT with MLOps in Smart Applications?
Bringing together IoT MLOps solves a major challenge — how to turn raw data into value without manual steps. Here’s why it’s important:
Benefits of Integration:
-
Real-Time Decisions: Smart systems respond instantly based on sensor data.
-
Automation: ML models are trained and deployed automatically.
-
Scalability: Supports thousands of devices with minimal human effort.
-
Improved Accuracy: Models update frequently with new data.
-
Faster Deployment: Reduces model lifecycle from weeks to hours.
How to Integrate IoT MLOps Step-by-Step
1. Connect Devices to Data Pipeline
Use protocols like MQTT or HTTP to stream sensor data from IoT devices to cloud platforms like AWS IoT, Azure IoT, or Google Cloud IoT.
2. Ingest and Store Data
Store incoming data using time-series databases like InfluxDB, or cloud storage like Amazon S3.
3. Build and Train ML Models
Use platforms like TensorFlow, PyTorch, or Scikit-learn to train models on historical data.
4. Deploy Models via MLOps Tools
Tools like MLflow, Kubeflow, and SageMaker help automate:
-
Version control
-
Model deployment
-
Performance tracking
5. Monitor and Update Models
Use monitoring tools to detect drift and retrain models regularly. This keeps the IoT with MLOps system accurate.
Real-World Use Cases of IoT MLOps
1. Predictive Maintenance in Manufacturing
-
IoT sensors monitor equipment health.
-
MLOps models predict failure before it happens.
2. Smart Agriculture
-
Sensors track soil, weather, and crops.
-
ML models optimize irrigation and fertilization.
3. Remote Health Monitoring
-
Wearables collect patient data.
-
Models detect anomalies like irregular heart rates.
Challenges in Integrating IoT MLOps
1. Data Security
Keeping both IoT data and ML models secure is critical. Use encryption, secure APIs, and access controls.
2. Edge vs Cloud
Decide whether to run models on the edge (near the device) or in the cloud based on latency and bandwidth needs.
3. Model Maintenance
Automation is key. Use MLOps pipelines to detect when a model is outdated and retrain it.
Best Tools for Managing IoT MLOps
Top Platforms to Consider:
-
AWS IoT + SageMaker
-
Azure IoT Hub + Azure ML
-
Google Cloud IoT + Vertex AI
-
Edge Impulse for edge-based AI
-
Kubeflow for open-source MLOps
These platforms help you scale IoT MLOps efficiently while offering integrations, security, and automation.
FAQ
What is the difference between IoT and MLOps?
IoT collects and sends data from devices, while MLOps manages machine learning model workflows.
Can I use IoT data to train ML models?
Yes. That’s the foundation of integrating IoT with MLOps — using real-time sensor data to build smarter systems.
Do I need the cloud for MLOps?
Not always. You can run MLOps pipelines at the edge using lighter tools if latency is a concern.
Is IoT with MLOps only for big companies?
No. Tools like Edge Impulse and open-source frameworks make it accessible for startups and small businesses too.
Future-Proof Smart Applications IoT with MLOps
Combining IoT with MLOps allows smart systems to not only collect data but also learn and act on it. This integration improves automation, accuracy, and efficiency in industries worldwide.
If you’re building smart apps — whether for farming, factories, or fitness — you’ll need to adopt IoT MLOps to stay competitive.
Start small, choose the right tools, and scale as needed. The future of intelligent systems starts with this integration.
Author Profile
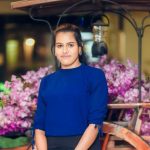
- Online Media & PR Strategist
- Hello there! I'm Online Media & PR Strategist at NeticSpace | Passionate Journalist, Blogger, and SEO Specialist
Latest entries
VirtualizationApril 30, 2025Future-Proof Virtualization Strategy for Emerging Tech
Simulation and ModelingApril 30, 2025Chaos Engineering: Build Resilient Systems with Chaos Monkey
Digital Twin DevelopmentApril 30, 2025How to Ensure Data Synchronization Twins Effectively
Scientific VisualizationApril 30, 2025Deepfake Scientific Data: AI-Generated Fraud in Research