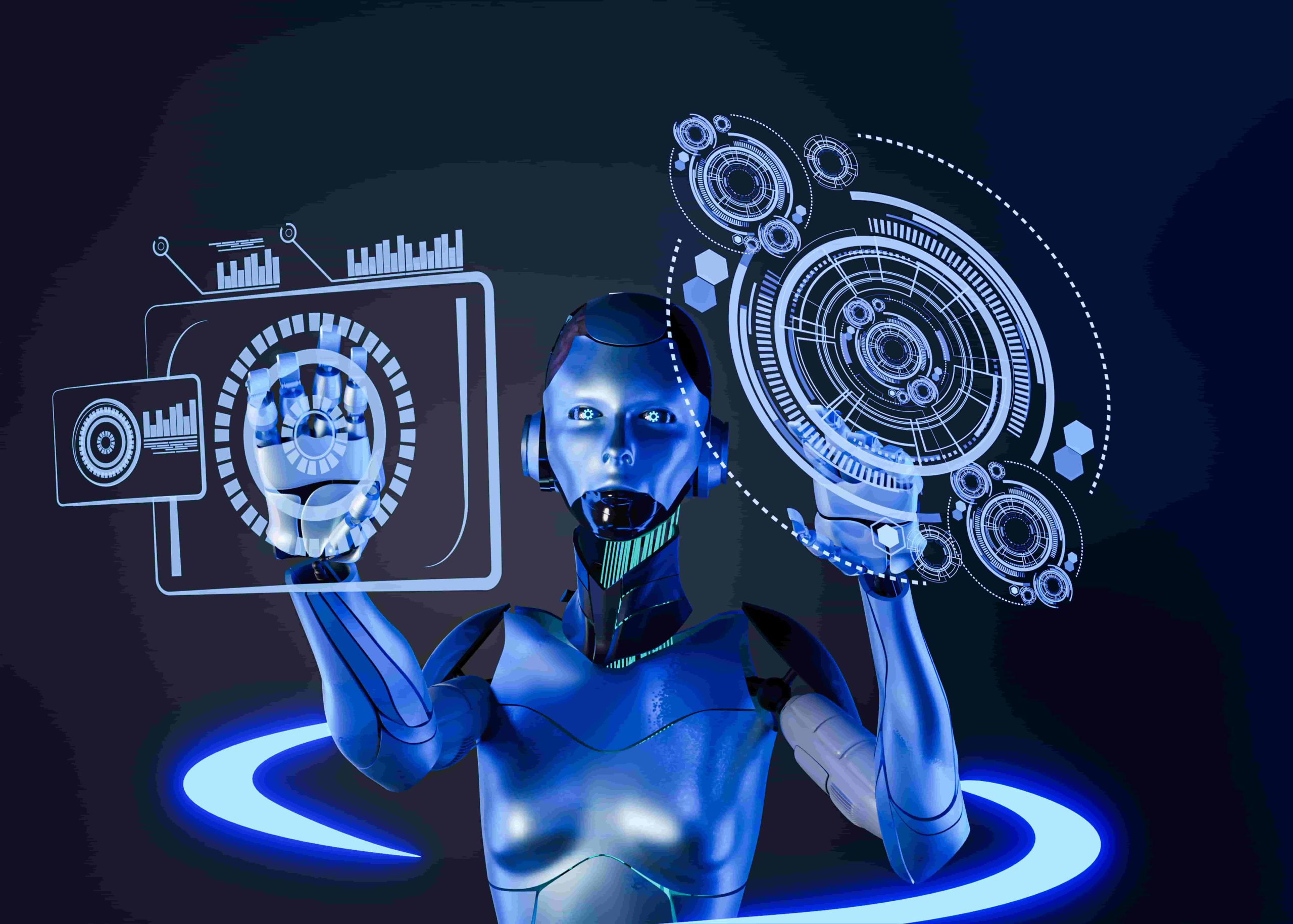
AI Workflow Challenges & Solutions | Avoid Common Pitfalls
Building AI solutions isn’t just about models. It’s about process.
AI workflows involve a mix of data collection, model training, deployment, and monitoring. But many teams hit roadblocks that delay or even derail their progress.
In this article, you’ll learn about the most common AI workflow challenges, why they happen, and practical steps to avoid them. Whether you’re a developer, data engineer, or IT manager, this guide will help you build smarter, faster, and more reliable AI systems.
What Is an AI Workflow?
An AI workflow refers to the structured steps used to build and manage AI systems. These usually include:
-
Collecting and preparing data
-
Choosing the right model
-
Training and testing the model
-
Deploying the model to production
-
Monitoring and maintaining performance
Every step sounds simple—until it breaks.
Common AI Workflow Challenges and How to Avoid Them
1. Poor Data Quality and Preparation
AI workflow challenges often begin with data.
Why It Happens
-
Incomplete or missing data
-
Duplicate or inconsistent records
-
Data from different sources not aligned
How to Avoid It
-
Set up clear data validation rules
-
Use automated tools for cleaning and transformation
-
Standardize formats before feeding data to the model
Consider using open-source data cleaning tools or platforms like OpenRefine to streamline this stage.
2. Misalignment Between Teams
Collaboration issues are among the biggest AI workflow challenges.
Why It Happens
-
Data teams and developers work in silos
-
Business goals are not clearly defined
-
Communication gaps between departments
How to Avoid It
-
Use centralized project management tools
-
Set shared KPIs from the start
-
Involve business teams in planning sessions
Collaborative platforms like Jira or Confluence can help unify workflows.
3. Inadequate Model Versioning and Tracking
Lack of model tracking leads to confusion and inefficiency.
Why It Happens
-
No version control for models or experiments
-
Changes are not well documented
-
Reproducing results becomes hard
How to Avoid It
-
Use tools like MLflow or DVC for versioning
-
Keep track of experiments, parameters, and performance
-
Log every model change in a shared system
4. Deployment Bottlenecks
A trained model is useless if you can’t deploy it.
Why It Happens
-
Lack of deployment infrastructure
-
Manual handoffs between teams
-
Inconsistent environments
How to Avoid It
-
Automate deployment with CI/CD pipelines
-
Use containerization (e.g., Docker) for consistency
-
Leverage MLOps platforms for smooth rollouts
cloud services like AWS SageMaker or Azure ML can speed up deployment.
5. Monitoring and Maintenance Neglect
Even perfect models break over time.
Why It Happens
-
No monitoring in place
-
Data drift or concept drift ignored
-
No retraining schedule
How to Avoid It
-
Set up real-time monitoring dashboards
-
Use alerts for drops in accuracy or data changes
-
Plan retraining cycles into your workflow
Best Practices to Overcome AI Workflow Challenges
Here are some general tips that help overcome many AI workflow challenges:
-
Start small and scale gradually
-
Document every step and decision
-
Use consistent naming, formats, and tracking tools
-
Include fail-safes for every critical step
-
Encourage continuous learning among team members
Frequently Asked Questions (FAQ)
What is the biggest challenge in AI workflows?
One of the biggest AI workflow challenges is managing poor data quality and inconsistency, which affects all later stages.
How do I manage multiple models efficiently?
Use model versioning tools and MLOps platforms that allow you to track, update, and rollback models easily.
Is automation important in AI workflows?
Yes. Automating tasks like deployment and monitoring helps reduce human error and saves time.
What tools can help avoid common AI workflow pitfalls?
Tools like MLflow, DVC, Docker, and cloud-based platforms can reduce friction across stages of your AI pipeline.
Conclusion
AI workflows can be powerful—but only if managed well. Avoiding common AI workflow challenges can improve model accuracy, reduce downtime, and save your team countless hours.
Focus on quality data, team alignment, automation, and ongoing monitoring. With the right tools and mindset, you’ll set your AI projects up for long-term success.
For more tips on managing tech workflows and IT best practices, explore Google’s AI best practices or Microsoft’s AI guidelines.
Author Profile
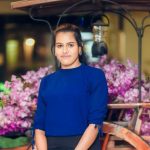
- Online Media & PR Strategist
- Hello there! I'm Online Media & PR Strategist at NeticSpace | Passionate Journalist, Blogger, and SEO Specialist
Latest entries
Data AnalyticsJune 13, 2025Future of Data Warehousing in Big Data
AI InterfaceJune 13, 2025Aligning AI Developments with Corporate Goals in the AI Era
HPC and AIJune 13, 2025HPC Architecture Taking to the Next Level
Quantum ComputingJune 13, 2025Ethical Issues in Quantum Tech: Privacy, Jobs, and Policy