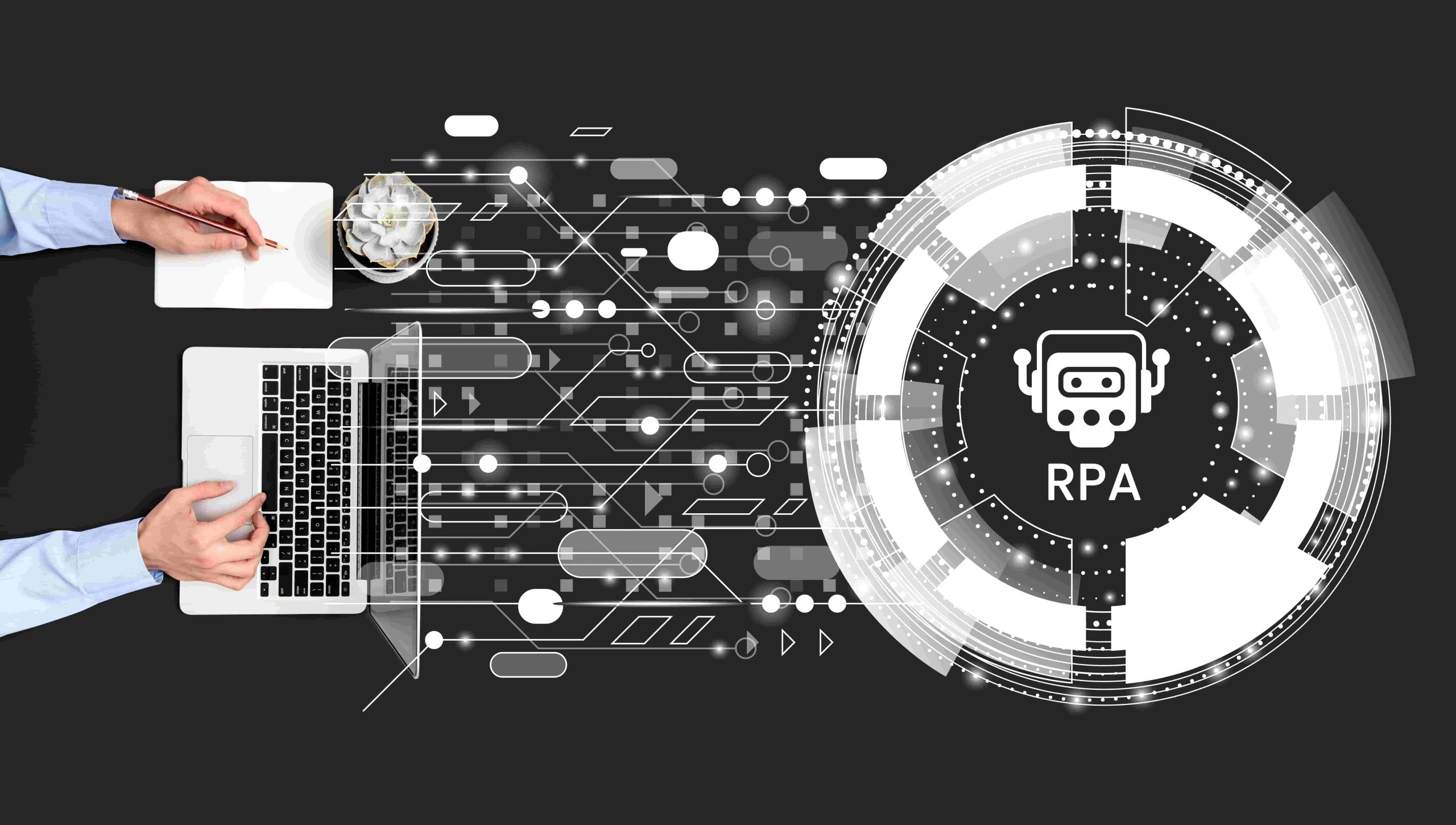
The Future of MLOps with Low-Code and No-Code AI Platforms
Introduction: How AI Development is Getting Easier
The world of AI is moving fast. But building machine learning (ML) solutions still takes time, skill, and coding knowledge. That’s changing.
Today, low-code and no-code AI platforms are making it easier for teams to create and manage machine learning workflows. This change is having a huge impact on MLOps—a field focused on streamlining the deployment and monitoring of ML models.
In this blog post, you’ll learn:
-
What MLOps is and why it matters
-
How low-code and no-code AI platforms are reshaping MLOps
-
The benefits and challenges of this new trend
-
Key platforms and tools driving the shift
-
What the future looks like for businesses and developers
Let’s dive in.
What Is MLOps and Why It Matters
MLOps (Machine Learning Operations) is a set of practices that help data science and IT teams deploy, monitor, and maintain ML models in production. It brings together machine learning, DevOps, and data engineering.
Why it matters:
-
It ensures ML models run smoothly in real-world environments
-
It reduces downtime and improves accuracy
-
It helps teams scale their AI projects
But MLOps has challenges. Traditional MLOps pipelines require coding skills, complex tools, and cross-team collaboration. That’s where low-code and no-code AI platforms come in.
How Low-Code and No-Code AI Platforms Support MLOps
Low-code and no-code AI platforms allow users to build and deploy machine learning models with minimal or no programming. These tools use drag-and-drop interfaces and built-in automation.
Key Ways They Support MLOps:
-
Faster Development
Teams can build ML models in days instead of weeks. No need to write lines of code. -
Wider Access
Business users, analysts, and non-developers can now contribute to AI projects. -
Simplified Deployment
Many platforms offer one-click deployment features with version control and monitoring. -
Automation
These platforms automate testing, retraining, and model validation steps.
Example: Microsoft Azure ML and Google AutoML are making MLOps more accessible with visual tools and auto-ML pipelines.
Benefits of Using Low-Code and No-Code in MLOps
1. Lower Barrier to Entry
Organizations don’t need large, highly technical teams. This reduces hiring costs and speeds up projects.
2. Faster Time to Market
AI solutions can move from idea to production faster, helping businesses stay competitive.
3. Improved Collaboration
Non-technical team members can participate in model design and feedback.
4. Easier Maintenance
These platforms often include built-in MLOps features like monitoring, retraining, and version control.
Challenges and Limitations to Consider
While promising, low-code and no-code AI platforms aren’t perfect. There are still concerns.
1. Limited Customization
Advanced models may require full coding for fine-tuning, which low-code tools can’t fully support.
2. Vendor Lock-In
You may become too dependent on one platform’s ecosystem.
3. Security and Compliance
Some platforms may not meet strict enterprise or regulatory needs.
4. Data Handling Limitations
Uploading and processing large datasets may still need engineering effort.
Top Platforms Shaping the Future of MLOps with Low-Code and No-Code
Here are some tools leading the movement:
-
Google Cloud AutoML – Best for beginners and fast prototyping
-
Microsoft Azure ML Studio – Offers MLOps capabilities with drag-and-drop UI
-
DataRobot – Enterprise-level no-code AI platform with automated MLOps
-
H2O.ai – Open-source, low-code ML platform with enterprise solutions
-
Amazon SageMaker Canvas – A no-code extension of SageMaker for ML
Each of these tools helps integrate MLOps with low-code and no-code AI platforms, bringing automation and collaboration to new heights.
The Future of MLOps: Trends to Watch
Looking ahead, the trend is clear. Businesses want AI tools that are easy to use, fast to deploy, and require less technical skill.
Emerging Trends:
-
AutoMLOps: Fully automated MLOps pipelines from data to deployment
-
AI Model Marketplaces: Plug-and-play models with minimal setup
-
Citizen Data Scientists: Non-experts driving ML innovation with no-code tools
-
Edge MLOps: Deploying models on devices using no-code interfaces
According to Gartner, by 2026, 80% of new AI projects will use low-code or no-code tools in at least one part of the pipeline.
FAQs on the Future of MLOps with Low-Code and No-Code AI Platforms
What is MLOps in simple terms?
MLOps means managing machine learning models so they work well in real life—like DevOps, but for AI.
Can non-coders really use AI platforms?
Yes. Many low-code and no-code AI platforms let users build models using visual tools.
Are no-code AI platforms secure?
Most are built with security in mind, but it depends on the vendor. Always review data policies.
Will low-code tools replace data scientists?
Not completely. They will assist, not replace. Complex projects still need experts.
Making AI More Accessible for Everyone
The future of MLOps with low-code and no-code AI platforms is all about inclusion and speed. These tools are breaking down walls between business and tech. They allow more people to join the AI conversation and build real solutions.
As tools improve, expect to see AI development become part of everyday workflows across industries. Whether you’re a startup or an enterprise, now is the time to explore these platforms and bring AI to your teams.
Author Profile
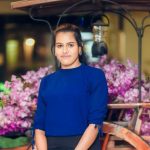
- Online Media & PR Strategist
- Hello there! I'm Online Media & PR Strategist at NeticSpace | Passionate Journalist, Blogger, and SEO Specialist
Latest entries
Data AnalyticsJune 13, 2025Future of Data Warehousing in Big Data
AI InterfaceJune 13, 2025Aligning AI Developments with Corporate Goals in the AI Era
HPC and AIJune 13, 2025HPC Architecture Taking to the Next Level
Quantum ComputingJune 13, 2025Ethical Issues in Quantum Tech: Privacy, Jobs, and Policy