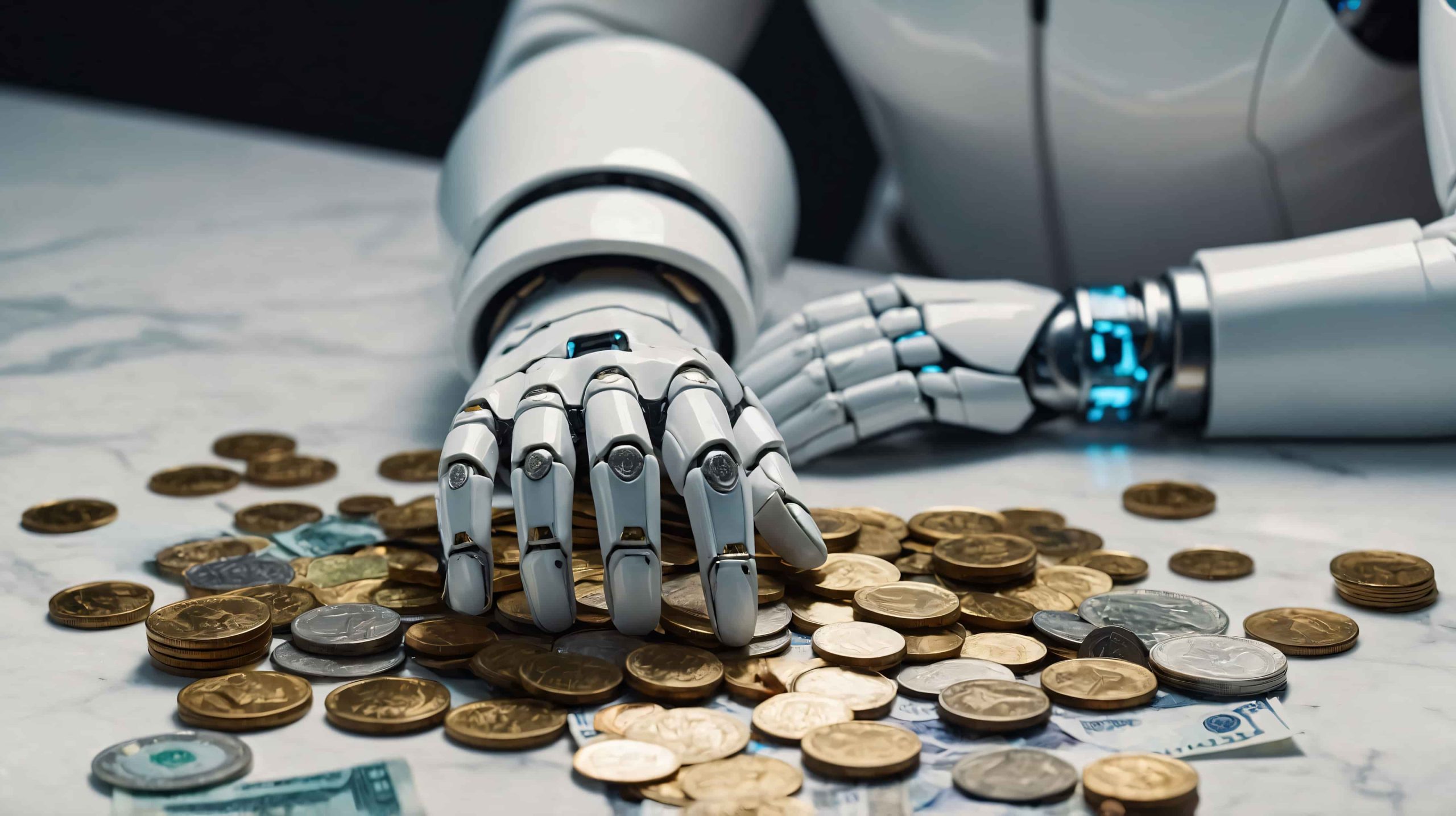
How to Manage Technical Debt in Machine Learning Projects
Machine learning (ML) is transforming how businesses operate. But behind the innovation lies a common challenge: technical debt. If left unmanaged, it can slow down development, introduce bugs, and inflate maintenance costs.
In this blog, you’ll learn how to manage technical debt in machine learning projects. We’ll cover the causes, consequences, and research-backed strategies to reduce and prevent technical debt. Whether you’re an ML engineer, data scientist, or IT manager, this guide is for you.
What is Technical Debt in Machine Learning Projects?
Technical debt refers to shortcuts or compromises made in code, architecture, or design that make future development harder. In ML, this debt can grow quickly due to:
-
Rapid prototyping
-
Lack of documentation
-
Model complexity
-
Data quality issues
Types of Technical Debt in ML
-
Code Debt: Poorly written, untested, or duplicated code.
-
Data Debt: Inconsistent or undocumented datasets.
-
Model Debt: Overfitted or opaque models that are hard to interpret or update.
-
Pipeline Debt: Hard-coded or fragile ML pipelines.
According to a Google Research paper titled “Machine Learning: The High-Interest Credit Card of Technical Debt” (source), ML systems introduce complex forms of debt due to entangled code and data dependencies.
Why Managing Technical Debt in ML is Critical
Reduced Agility
High technical debt slows down updates and innovation.
Increased Costs
Debugging and maintaining messy ML systems becomes expensive over time.
Higher Risk of Failure
Poor documentation or data inconsistency can lead to failed model predictions and system crashes.
How to Manage Technical Debt in Machine Learning Projects
Managing debt isn’t about eliminating it completely. It’s about keeping it under control while building sustainable ML systems.
Start with Strong Documentation
Good documentation reduces onboarding time and confusion.
Tips:
-
Document data sources and schema
-
Explain model choices and assumptions
-
Include comments in code
-
Version control everything using Git
Research by Microsoft (source) shows that lack of documentation is a major contributor to long-term technical debt.
Design for Reusability and Modularity
Best Practices:
-
Break code into small, reusable functions
-
Avoid hardcoding paths or parameters
-
Use configuration files for environment variables
This reduces model and pipeline debt and makes refactoring easier.
Automate Testing and Monitoring
Testing ML systems is tricky due to dynamic data.
What to Do:
-
Use unit tests for preprocessing and transformations
-
Validate model outputs regularly
-
Set up alerts for model drift or performance degradation
Tools like Great Expectations or MLflow help monitor data and model quality.
Keep Data Clean and Versioned
Dirty data is one of the biggest causes of data debt.
Strategies:
-
Set up data validation pipelines
-
Use tools like DVC (Data Version Control)
-
Track dataset lineage
Refactor and Review Regularly
Schedule Time for:
-
Code reviews
-
Technical debt assessment
-
Refactoring old scripts or pipelines
Small, frequent improvements reduce the build-up of hidden debt.
Encourage Cross-Team Collaboration
Miscommunication between data scientists, ML engineers, and DevOps teams leads to fragile systems.
Solutions:
-
Use shared platforms like MLflow or Kubeflow
-
Set common goals and metrics
-
Conduct joint reviews
Choose the Right Tools and Frameworks
Recommended Tools:
-
MLflow for experiment tracking
-
Kedro or TFX for pipeline management
-
Docker for environment consistency
The right tools reduce pipeline and environment debt.
FAQ: How to Manage Technical Debt in Machine Learning Projects
What causes technical debt in ML projects?
Rapid development, lack of documentation, and poor pipeline design are common causes.
How can I track technical debt?
Use project management tools with technical debt tracking features, like Jira. Conduct regular reviews and audits.
What tools help manage ML technical debt?
MLflow, Great Expectations, Kedro, and DVC are popular tools.
Is technical debt always bad?
Not always. Some debt is acceptable if it helps meet short-term goals. The key is to manage and reduce it over time.
Conclusion: Take Charge Before It’s Too Late
Managing technical debt in machine learning projects is essential for long-term success. You don’t have to fix everything overnight. Start small—clean your data, document your models, and use the right tools. Regularly review and refactor your systems to keep debt from spiraling.
With the right strategy, you’ll save time, cut costs, and build ML systems that scale.
Author Profile
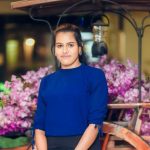
- Online Media & PR Strategist
- Hello there! I'm Online Media & PR Strategist at NeticSpace | Passionate Journalist, Blogger, and SEO Specialist
Latest entries
Data AnalyticsJune 13, 2025Future of Data Warehousing in Big Data
AI InterfaceJune 13, 2025Aligning AI Developments with Corporate Goals in the AI Era
HPC and AIJune 13, 2025HPC Architecture Taking to the Next Level
Quantum ComputingJune 13, 2025Ethical Issues in Quantum Tech: Privacy, Jobs, and Policy