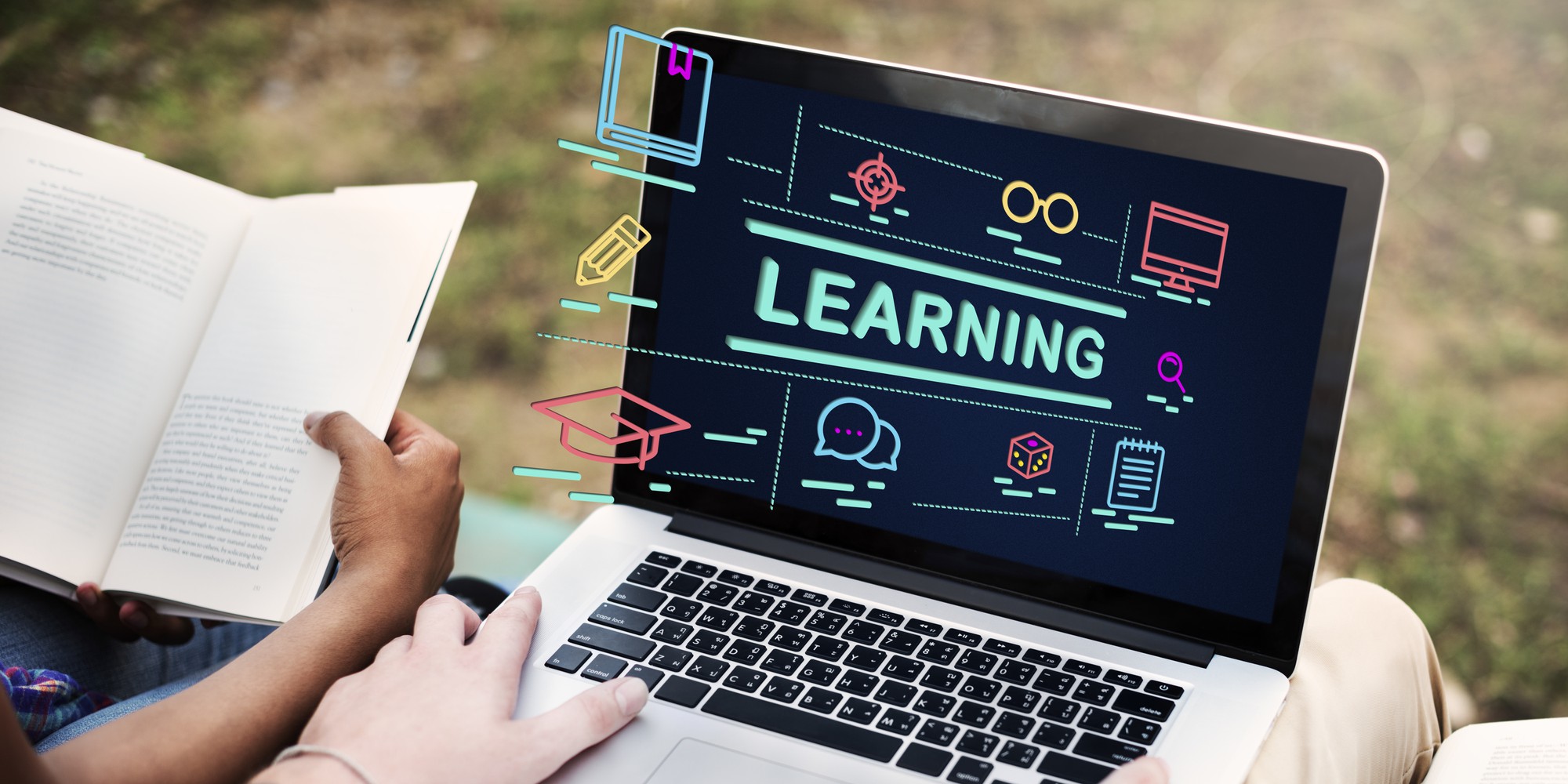
How Federated Learning is Changing the MLOps Landscape
Federated learning in MLOps is gaining traction as teams seek better ways to train models without sacrificing data privacy. MLOps workflows have long depended on centralized datasets, but this method poses risks and compliance issues. Federated learning solves these problems by allowing decentralized training—making it a game-changer for modern machine learning systems.
In this article, you’ll learn:
- What federated learning is
- How federated learning supports MLOps workflows
- Real-world applications of federated learning in MLOps
- Tools and frameworks enabling this shift
- Key challenges and the future of federated learning in MLOps
Let’s dive in.
What Is Federated Learning?
Federated learning is a machine learning technique where model training happens across multiple devices or servers holding local data. Instead of sending data to a central location, each device trains the model locally and only shares updates.
Key Features of Federated Learning in MLOps:
- Data stays on the device
- Only model updates are shared
- Helps meet privacy rules like GDPR and HIPAA
Example:
Google’s Gboard improves its text prediction by training models on your phone using federated learning—without collecting your keystrokes.
Why Federated Learning Matters for MLOps
MLOps deals with managing machine learning models from development to deployment. Federated learning fits well by solving several modern challenges:
1. Data Privacy at the Edge in MLOps
Centralized data pipelines carry security risks. Keeping data on local devices helps reduce exposure.
2. Meeting Compliance Standards in Federated MLOps
Privacy regulations are tightening. Decentralized model training simplifies compliance with data protection laws.
3. Efficient Training Pipelines in MLOps
No need to transfer large datasets. Local training speeds up development and deployment.
Real-World Uses of Federated Learning in MLOps
Federated Learning for Healthcare MLOps
Hospitals can train shared models for diagnostics while keeping patient data private.
Federated Learning in Finance
Banks collaborate on fraud detection models using local transaction data without sharing it.
Smartphone MLOps with Federated Learning
Phones update voice and text models using on-device training, improving services without sending data to the cloud.
Tools That Support Federated Learning in MLOps
Several open-source tools help teams bring federated learning into MLOps workflows.
TensorFlow Federated for MLOps
From Google, this supports decentralized training on distributed data using TensorFlow.
PySyft Integration in MLOps
From OpenMined, it supports secure and private machine learning.
Flower for Federated Learning
Flexible and framework-agnostic, ideal for production-scale federated systems in MLOps environments.
Common Challenges in Federated MLOps
This approach has some drawbacks that teams need to solve:
1. Uneven Data Distribution
Devices may have biased or incomplete datasets, affecting federated learning outcomes.
2. Limited Device Power in MLOps Edge Devices
Edge devices may lack the resources for full model training.
3. Slow Communication in Federated Systems
Sharing updates across many devices can introduce lag.
Solutions include federated averaging and techniques like differential privacy.
The Road Ahead for Federated Learning in MLOps
Adoption is growing, especially among tech giants like Google and Apple. We’ll likely see:
- More plug-and-play MLOps tools with federated learning built-in
- Improved performance on edge devices
- Enhanced privacy protections for federated pipelines
This technique will be essential for any team that values security and speed in their machine learning workflows.
FAQs
Who benefits most from federated learning in MLOps?
Industries like healthcare, banking, and mobile tech benefit the most due to data sensitivity.
Is it more secure than traditional training?
Yes. It keeps raw data off the cloud, reducing breach risk.
Can it be added to existing MLOps workflows?
Yes. Tools like Kubeflow and MLflow support integration.
Is federated learning real-time?
It’s near real-time today, and performance is improving.
Author Profile
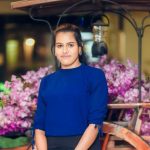
- Online Media & PR Strategist
- Hello there! I'm Online Media & PR Strategist at NeticSpace | Passionate Journalist, Blogger, and SEO Specialist
Latest entries
HPC and AIApril 30, 2025AI and HPC in Gaming: Realistic Virtual Worlds Today
Robotics SimulationApril 30, 2025How Robotics Simulation Agriculture Is Changing Farming
VirtualizationApril 30, 2025Future-Proof Virtualization Strategy for Emerging Tech
Simulation and ModelingApril 30, 2025Chaos Engineering: Build Resilient Systems with Chaos Monkey