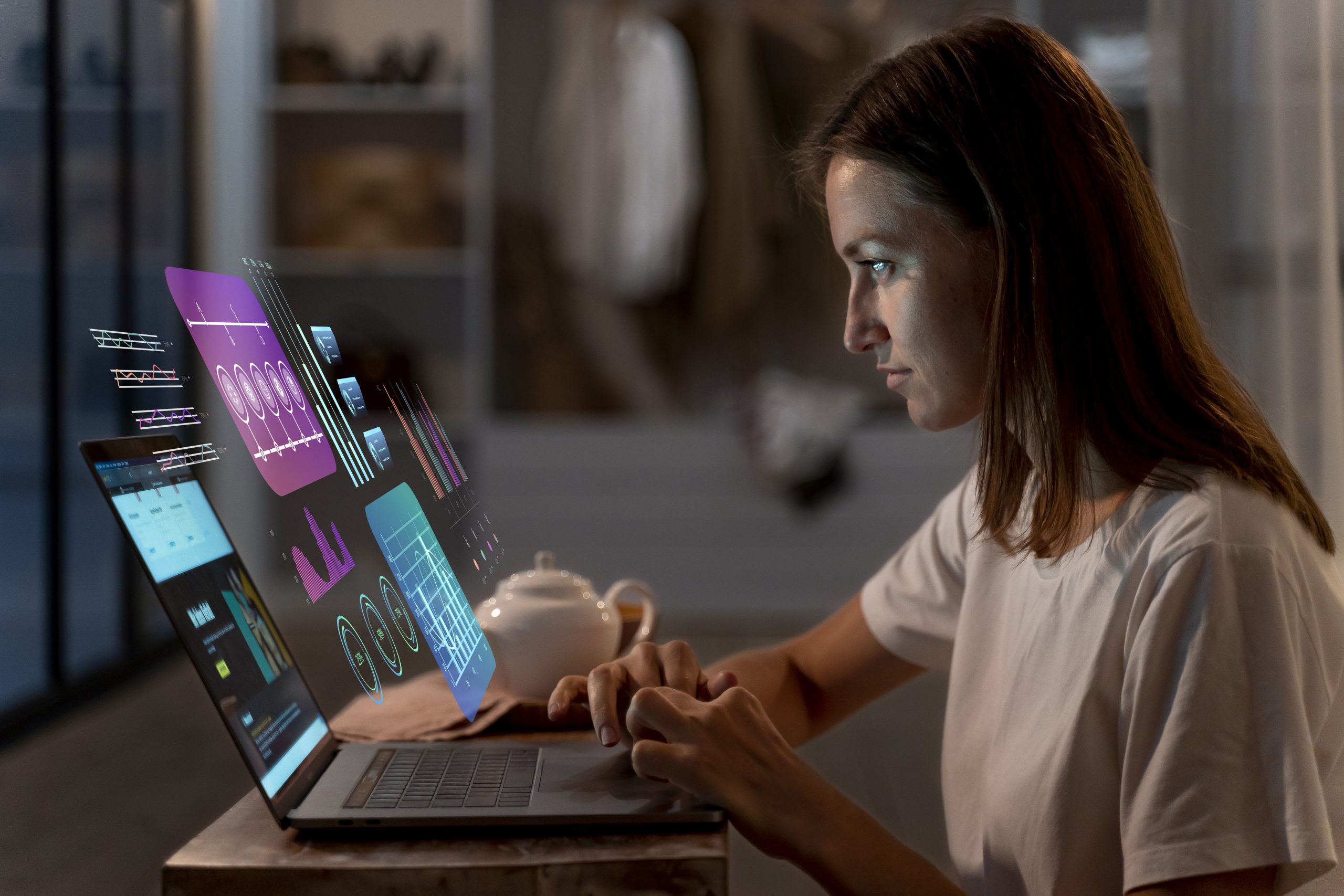
Best Practices for Versioning Data and Models in MLOps
MLOps (Machine Learning Operations) is essential for managing machine learning projects efficiently. One of the biggest challenges in MLOps is versioning data and models to ensure reproducibility, traceability, and smooth collaboration. Without proper version control, teams struggle to track changes, leading to inconsistencies in model performance and deployment issues.
In this article, you’ll learn best practices for versioning data and models in MLOps. We’ll cover why versioning is crucial, strategies for effective version control, and tools that simplify the process.
Why Versioning Matters in MLOps
Model development is an iterative process. Without proper versioning, teams face challenges such as:
- Lack of Reproducibility: Inconsistent results due to missing dataset versions.
- Difficult Collaboration: Team members struggle to sync changes.
- Deployment Issues: Outdated models may be deployed accidentally.
By implementing structured versioning, teams can streamline workflows and enhance model performance.
Best Practices for Versioning Data and Models
1. Use a Structured Naming Convention
A clear naming convention prevents confusion and ensures traceability. Follow these best practices:
- Data Versioning: Use dataset version numbers (e.g.,
dataset_v1.0
,dataset_v1.1
). - Model Versioning: Use semantic versioning (e.g.,
model_v1.0
,model_v1.2
). - Timestamps: Append dates for better tracking (e.g.,
dataset_2024-03-14
).
2. Leverage Data Version Control (DVC)
Data Version Control (DVC) is an essential tool for managing datasets and model files efficiently. It integrates with Git and enables:
- Tracking large datasets
- Efficient storage and retrieval
- Version control integration with code repositories
3. Store Metadata Alongside Data
Metadata provides context for datasets and models. Always store:
- Source of the dataset
- Preprocessing steps applied
- Feature engineering details
Tools like MLflow and DVC help in maintaining metadata efficiently.
4. Automate Versioning with CI/CD Pipelines
MLOps thrives on automation. Integrate versioning into CI/CD pipelines to:
- Track model improvements
- Ensure consistent deployments
- Reduce manual errors
5. Maintain Model Lineage
Understanding how a model evolved is crucial for debugging and audits. Maintain:
- Model training history
- Hyperparameter changes
- Evaluation metrics across versions
6. Use Cloud Storage for Scalable Versioning
Cloud-based storage solutions such as AWS S3, Google Cloud Storage, and Azure Blob Storage help in versioning large datasets and models effectively.
7. Implement Role-Based Access Control (RBAC)
Access control ensures only authorized users can modify datasets and models, preventing unintended changes.
Tools for Versioning Data and Models
1. Git & GitHub/GitLab
- Ideal for tracking code and small datasets.
- Use Git LFS for large files.
2. DVC (Data Version Control)
- Manages large datasets with Git-like functionality.
- Supports cloud storage integration.
3. MLflow
- Tracks model experiments, parameters, and versions.
- Supports deployment tracking.
4. Pachyderm
- Provides data lineage and pipeline versioning.
- Automates data transformation tracking.
5. Weights & Biases
- Tracks experiment logs and model versions.
- Provides visualization tools for better analysis.
FAQs
1. Why is versioning important in MLOps?
Versioning ensures reproducibility, consistency, and collaboration by tracking changes in datasets and models.
2. What is the best tool for versioning datasets?
DVC and Pachyderm are popular choices for versioning large datasets effectively.
3. How do I ensure version consistency across teams?
Use a structured naming convention, automate versioning with CI/CD, and enforce RBAC policies.
4. Can I use Git for model versioning?
Git works for small models, but for larger ones, tools like DVC or MLflow are better suited.
Conclusion
Versioning data and models in MLOps is critical for maintaining reproducibility and collaboration. By using structured naming conventions, leveraging tools like DVC and MLflow, and automating versioning through CI/CD, teams can efficiently manage ML projects.
Adopting these best practices will streamline workflows and prevent costly deployment mistakes. Start implementing version control today to scale your MLOps processes effectively.
Author Profile
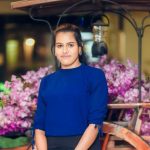
- Online Media & PR Strategist
- Hello there! I'm Online Media & PR Strategist at NeticSpace | Passionate Journalist, Blogger, and SEO Specialist
Latest entries
VirtualizationApril 30, 2025Future-Proof Virtualization Strategy for Emerging Tech
Simulation and ModelingApril 30, 2025Chaos Engineering: Build Resilient Systems with Chaos Monkey
Digital Twin DevelopmentApril 30, 2025How to Ensure Data Synchronization Twins Effectively
Scientific VisualizationApril 30, 2025Deepfake Scientific Data: AI-Generated Fraud in Research