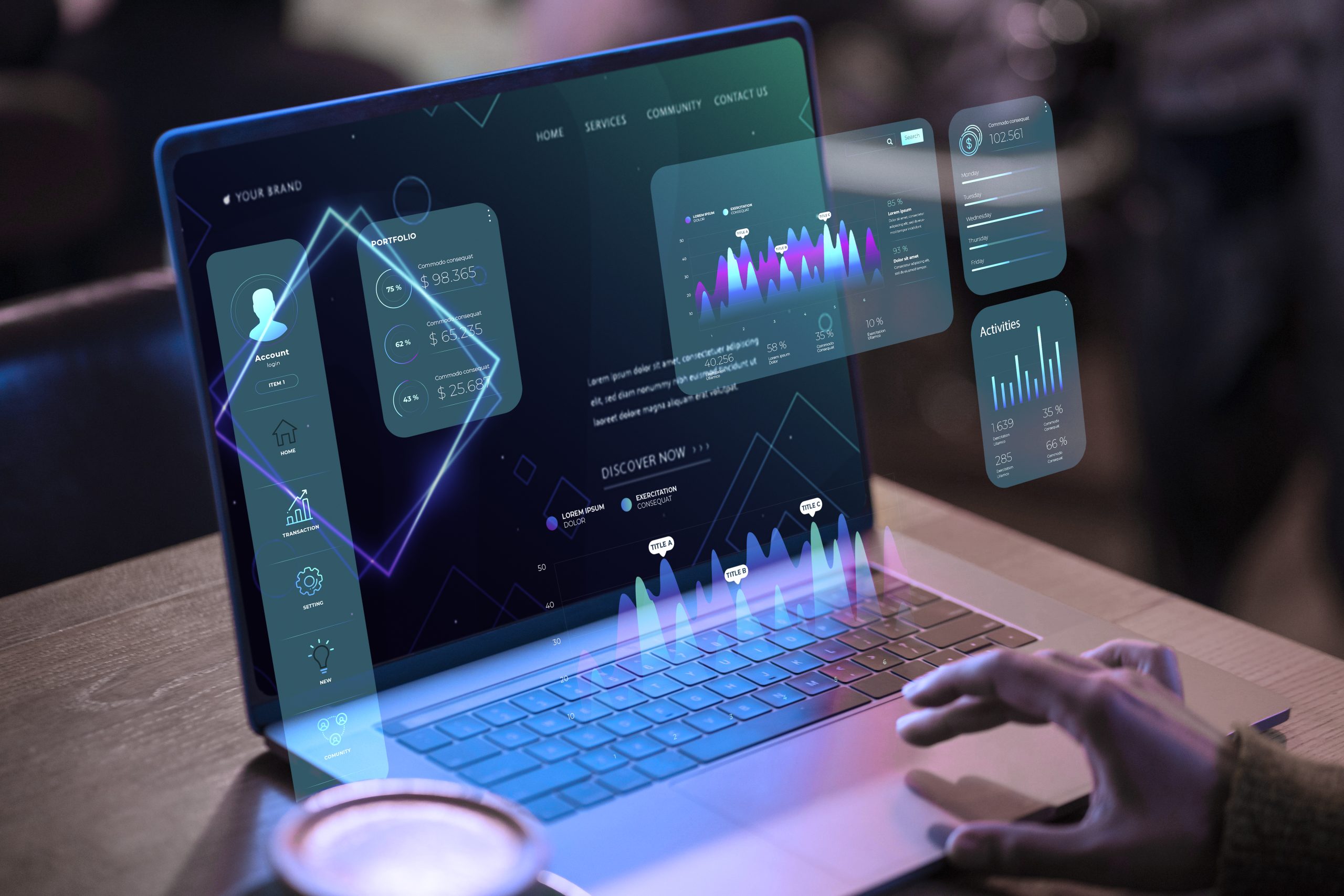
What Is MLOps? A Beginner’s Guide
Have you ever built a machine learning model that works well in a notebook but fails in production? That’s where MLOps comes in. MLOps helps teams manage the entire ML lifecycle. In this post, you will learn what MLOps is, why it differs from DevOps, and how it helps you scale machine learning efforts.
MLOps stands for Machine Learning Operations. It includes methods to automate and manage ML models from development to deployment. You’ll discover the key parts of MLOps, common tools, and best practices. By the end, you’ll see why MLOps is essential for modern data science teams.
Understanding MLOps
MLOps brings structure to how models are built, tested, and maintained. It extends the principles of DevOps to the machine learning world. Data scientists, ML engineers, and IT teams all contribute.
Core Elements of MLOps
- Data Management
Collecting, cleaning, and tracking data versions. - Model Development
Experimenting with architectures, hyperparameters, and feature sets. - Model Deployment
Pushing models into production environments, such as APIs or batch processes. - Monitoring and Maintenance
Tracking performance, detecting drift, and updating models when needed.
MLOps ensures each step is repeatable and transparent. It keeps your process organized, so you can focus on delivering insights faster.
How MLOps Differs From DevOps
At first, MLOps sounds a lot like DevOps. Both focus on continuous integration, continuous delivery, and collaboration. But there are key differences.
DevOps mostly deals with software code. It tracks changes in code, runs automated tests, and deploys apps. MLOps covers more territory. It includes data pipelines, model training, and performance monitoring. Data evolves over time, and models can degrade if the data changes. MLOps must handle these shifts.
DevOps can reuse code modules, but ML models depend on unique datasets. Changes in data can break a model’s performance. That’s why version control for data and models is central to MLOps. You need extra layers of tracking and validation to ensure accuracy.
Why Is MLOps Important?
First, MLOps helps you scale your machine learning efforts. A single model might work fine with ad-hoc processes. But as you add more models or bigger datasets, problems grow. MLOps organizes these tasks so you can deploy dozens of models without chaos.
Next, it reduces risks in production. ML models can drift or become stale if not monitored. MLOps sets up checks and alerts that spot issues early. This approach cuts downtime and preserves customer trust.
Finally, MLOps boosts collaboration. Data scientists want to experiment fast. IT teams need stable deployments. MLOps aligns these goals, saving time and preventing friction.
Key Steps in the MLOps Lifecycle
Below is a typical MLOps workflow. Each step ensures reliable and repeatable processes.
1. Data Collection and Preprocessing
- Gather datasets from internal or external sources.
- Clean, transform, and label them.
- Store data in a version-controlled repository.
2. Model Development
- Experiment with various model designs.
- Track hyperparameters, metrics, and code changes.
- Save each model version for later review.
3. Continuous Integration (CI)
- Automate model testing with unit tests or integration tests.
- Validate data schema and distribution.
- Check code quality and style with linting tools.
4. Continuous Deployment (CD)
- Package the model into a container or microservice.
- Deploy to staging or production automatically.
- Use infrastructure as code (IaC) for consistent environments.
5. Monitoring and Feedback
- Collect real-time metrics, such as inference speed and accuracy.
- Compare predictions against ground truth if available.
- Create alerts for performance drops or data drift.
6. Model Retraining
- Trigger model refreshes when data changes or performance declines.
- Validate the new model against baseline metrics.
- Roll out the updated model gradually, using strategies like canary releases.
This loop repeats as your product evolves. Each cycle refines models, data, and processes.
Best Practices for Effective MLOps
MLOps can be tricky. Follow these best practices to increase your success rate.
- Version Everything
Track code, data, and model binaries in separate repositories. Use tags or commit hashes for clarity. - Automate as Much as Possible
Use continuous integration pipelines to test and package models. Save time and reduce human error. - Use Feature Stores
Store key features in a central place. This ensures consistent data usage across training and inference. - Adopt a Clear Governance Policy
Define roles, responsibilities, and guidelines for each step. This includes data privacy and model explainability. - Test for Bias
ML models can inherit bias from data. Run fairness tests to detect and correct it. - Implement Monitoring Dashboards
Real-time metrics help you catch anomalies early. Tools like Prometheus or Grafana can track custom ML metrics.
Following these guidelines results in smoother deployments and higher model reliability.
Common MLOps Tools and Frameworks
A variety of platforms simplify MLOps tasks. Below are some popular options:
- MLflow
Tracks experiments, stores models, and manages deployments. - Kubeflow
Runs ML pipelines on Kubernetes, with a focus on scalability. - DVC (Data Version Control)
Manages large datasets and model files with Git-like commands. - TensorFlow Extended (TFX)
Offers pipeline components for training and serving TF models. - Airflow
Orchestrates complex workflows for data ingestion and model training.
Each tool meets different needs. Evaluate your project size, budget, and team skillset before choosing a platform.
Common Pitfalls in MLOps
Despite best efforts, mistakes happen. Here are a few common pitfalls to avoid:
- Ignoring Data Quality
Poor data leads to bad models. Always inspect data for errors or bias. - Lack of Governance
Without clear rules on model usage, teams might push untested models into production. - Overcomplicating Pipelines
Keep your initial MLOps setup simple. Add advanced features only when needed. - Inconsistent Environments
If local and cloud setups differ, you’ll face surprises in production. Use containers or environment managers to keep them in sync. - Skipping Model Validation
Model performance can drop if data changes. Validate each release and monitor it constantly.
Learning from these pitfalls saves you time and prevents nasty surprises.
Real-World Applications of MLOps
Companies across industries rely on MLOps for smoother ML deployments:
- E-commerce
Personalization and recommendation models need frequent updates. MLOps automates data pulls and model rollouts. - Finance
Fraud detection models must retrain often due to changing fraud patterns. Continuous monitoring is essential for quick reaction. - Healthcare
Predictive models help with diagnostics. They must be tested thoroughly and meet strict regulations. MLOps ensures compliance and traceability. - Manufacturing
ML-driven quality checks identify defects on assembly lines. MLOps reduces downtime by updating detection models quickly.
In each case, MLOps offers a structured approach to building, deploying, and improving models over time.
The Future of MLOps
Machine learning will keep growing. So will MLOps. Expect more advanced automation, better integration with data pipelines, and stricter governance. Tools may also leverage AI to handle tasks like hyperparameter tuning or anomaly detection.
As more companies adopt ML, the need for reliable pipelines rises. MLOps will be at the center of this shift, guiding teams from small tests to enterprise-scale solutions. Staying aware of new features and best practices will help you stay competitive.
Conclusion
MLOps is the missing link between data science experiments and production-grade ML solutions. It provides structures and tools to handle data, models, and deployments at scale. Unlike DevOps, MLOps must address data versioning, model drift, and performance issues unique to machine learning.
First, define clear processes for data collection, model training, and testing. Next, automate as many steps as you can, from CI/CD pipelines to monitoring. Finally, pick the right tools, keep an eye on common pitfalls, and never stop optimizing.
By applying MLOps principles, you’ll deliver reliable, high-performing models that adapt to real-world changes. This approach saves time, reduces risks, and keeps your team focused on building smarter solutions. Embrace MLOps, and let your machine learning efforts thrive.
Author Profile
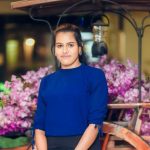
- Online Media & PR Strategist
- Hello there! I'm Online Media & PR Strategist at NeticSpace | Passionate Journalist, Blogger, and SEO Specialist
Latest entries
Simulation and ModelingApril 30, 2025Chaos Engineering: Build Resilient Systems with Chaos Monkey
Digital Twin DevelopmentApril 30, 2025How to Ensure Data Synchronization Twins Effectively
Scientific VisualizationApril 30, 2025Deepfake Scientific Data: AI-Generated Fraud in Research
Data AnalyticsApril 30, 2025What Is Data Mesh Architecture and Why It’s Trending