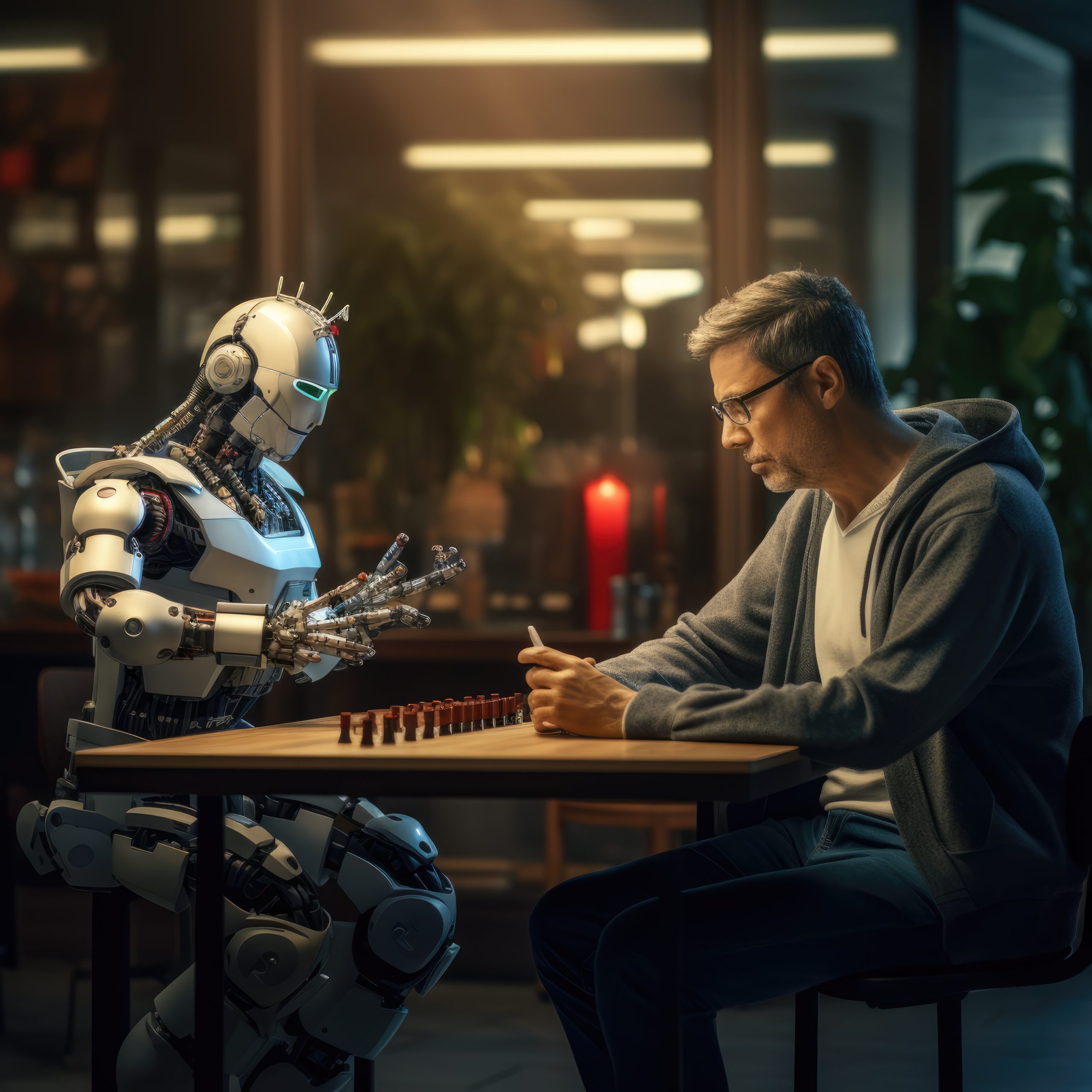
The Role of Quantum Computing in AI: Accelerating Machine Learning
Artificial intelligence (AI) moves fast. Yet, it often hits speed limits with traditional computing. That’s where quantum computing comes in. It holds the promise of accelerating machine learning and deep learning methods. In this blog post, you will learn what quantum computing is, how it can enhance AI algorithms, and where this technology is headed.
Purpose of this article:
- To drive traffic and generate leads for quantum computing and AI solutions
- To provide educational content on how quantum computing could accelerate AI
- To guide interested readers on the benefits and challenges of this new technology
Continue reading to discover the exciting role of quantum computing in artificial intelligence and how it could transform machine learning.
Understanding the Role of Quantum Computing in Artificial Intelligence
Quantum computing uses quantum bits (qubits) instead of classic bits. This allows qubits to be in multiple states at once, thanks to superposition. As a result, calculations can happen in ways classical computers cannot match. When applied to AI, quantum computing can reduce processing times for complex tasks.
Shorter processing times mean faster learning for AI. Machine learning algorithms often rely on huge data sets. By leveraging quantum properties, these algorithms can run complex calculations in parallel. This could lead to breakthroughs in tasks like natural language processing or image recognition.
Key Features of Quantum Computing for AI
-
Superposition
- Enables simultaneous exploration of multiple solutions
- Gives AI models more flexibility in finding patterns
-
Entanglement
- Connects qubits in a way that measuring one affects the other
- Can improve the accuracy of certain AI operations
-
Quantum Gates
- Manipulate qubits to perform calculations
- Act as building blocks for quantum algorithms
How Quantum Computing Speeds Up Machine Learning Algorithms
Machine learning relies on patterns from large data sets. Classical computers break these tasks into smaller steps. Quantum computers, on the other hand, can handle many of these steps at the same time.
This parallelism accelerates how we train AI models. Training that once took weeks might be reduced to days or hours. For example, quantum computing can power faster search strategies in optimization tasks. This is especially important in fields like drug discovery or financial modeling, where speed matters.
Potential Improvements in Machine Learning
-
Accelerated Training
- Quantum computers can test multiple hypotheses quickly
- Deep learning could see major gains in speed
-
Enhanced Data Analysis
- Clustering and classification might be more efficient
- Can handle complex data sets with fewer resources
-
Breakthrough Research Opportunities
- Exploratory machine learning algorithms become more feasible
- Opens doors to solving problems once deemed too large
Real-World Applications of Quantum Computing for AI
Quantum computing is not just theory. Several organizations are exploring ways to merge it with artificial intelligence. Companies like IBM, Google, and Microsoft invest in quantum research. Their goal is to build hardware and software that can handle complex AI tasks.
These projects often focus on chemistry simulations, financial modeling, and secure communication. For instance, quantum-driven AI could predict chemical reactions faster. It could also model financial markets more accurately. In cybersecurity, quantum algorithms might help detect unusual network behavior with greater speed.
Examples of Quantum-AI Projects
- Quantum Machine Learning Libraries
Companies are creating libraries to integrate quantum algorithms into popular AI frameworks. - Quantum Optimization Tools
Quantum-inspired methods help solve scheduling, routing, and other complex optimization tasks. - Quantum Communication
Research teams use entanglement to build secure communication channels, boosting AI-driven encryption.
Overcoming Challenges in Quantum Computing for Artificial Intelligence
Quantum computing is powerful, but it’s not perfect. Current quantum computers have limited qubits. They also suffer from noise and instability issues. These challenges make it hard to run algorithms at large scales.
Researchers are exploring error-correcting codes and more stable qubit materials. They aim to keep quantum systems coherent for longer. This will reduce errors and improve reliability. Despite these hurdles, progress is steady. As technology advances, we can expect better quantum hardware and more refined algorithms.
Ethical and Security Concerns
-
Data Privacy
- Quantum-based hacking threats may appear
- AI systems must ensure data protection
-
Resource Allocation
- Developing quantum computers requires rare materials
- Ethical sourcing of those materials is important
-
Skill Gaps
- Experts in quantum physics, AI, and computer science are rare
- Training more specialists is essential
The Future of Quantum AI
Quantum computing’s role in artificial intelligence grows each day. As we refine hardware, AI and machine learning will benefit. Complex tasks may become solvable in fractions of the time. This leap in computing speed could change industries, from healthcare to finance.
Still, many challenges remain, such as noise and limited qubits. Yet, the research community and industry leaders show promise. As quantum computers evolve, AI will harness their speed and power. Keeping an eye on this developing field can give you a competitive edge.
FAQ
1. What is the main benefit of quantum computing in artificial intelligence?
The primary benefit is faster processing speeds. Quantum computing can handle multiple states at once, speeding up tasks like training machine learning models.
2. Do quantum computers replace classical computers in AI?
Not right now. Quantum computers are still in early development. They often work alongside classical systems to handle specialized tasks.
3. How will quantum computing affect machine learning algorithms?
It can accelerate search and optimization processes. This can reduce the time needed for tasks like data clustering, pattern recognition, and model training.
4. Is quantum computing safe for data privacy?
Quantum technology has the potential to create more secure encryption methods. However, it also poses risks if used to crack existing encryption. Ongoing research aims to balance these concerns.
5. Why is quantum computing still not widely used in AI?
Current quantum hardware is expensive and limited by noise. Researchers are working on error-correction solutions and better hardware designs. Wider adoption will come as systems become more stable.
Author Profile
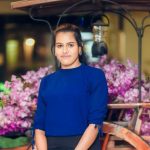
- Online Media & PR Strategist
- Hello there! I'm Online Media & PR Strategist at NeticSpace | Passionate Journalist, Blogger, and SEO Specialist
Latest entries
Scientific VisualizationApril 30, 2025Deepfake Scientific Data: AI-Generated Fraud in Research
Data AnalyticsApril 30, 2025What Is Data Mesh Architecture and Why It’s Trending
Rendering and VisualizationApril 30, 2025Metaverse Rendering Challenges and Opportunities
MLOpsApril 30, 2025MLOps 2.0: The Future of Machine Learning Operations