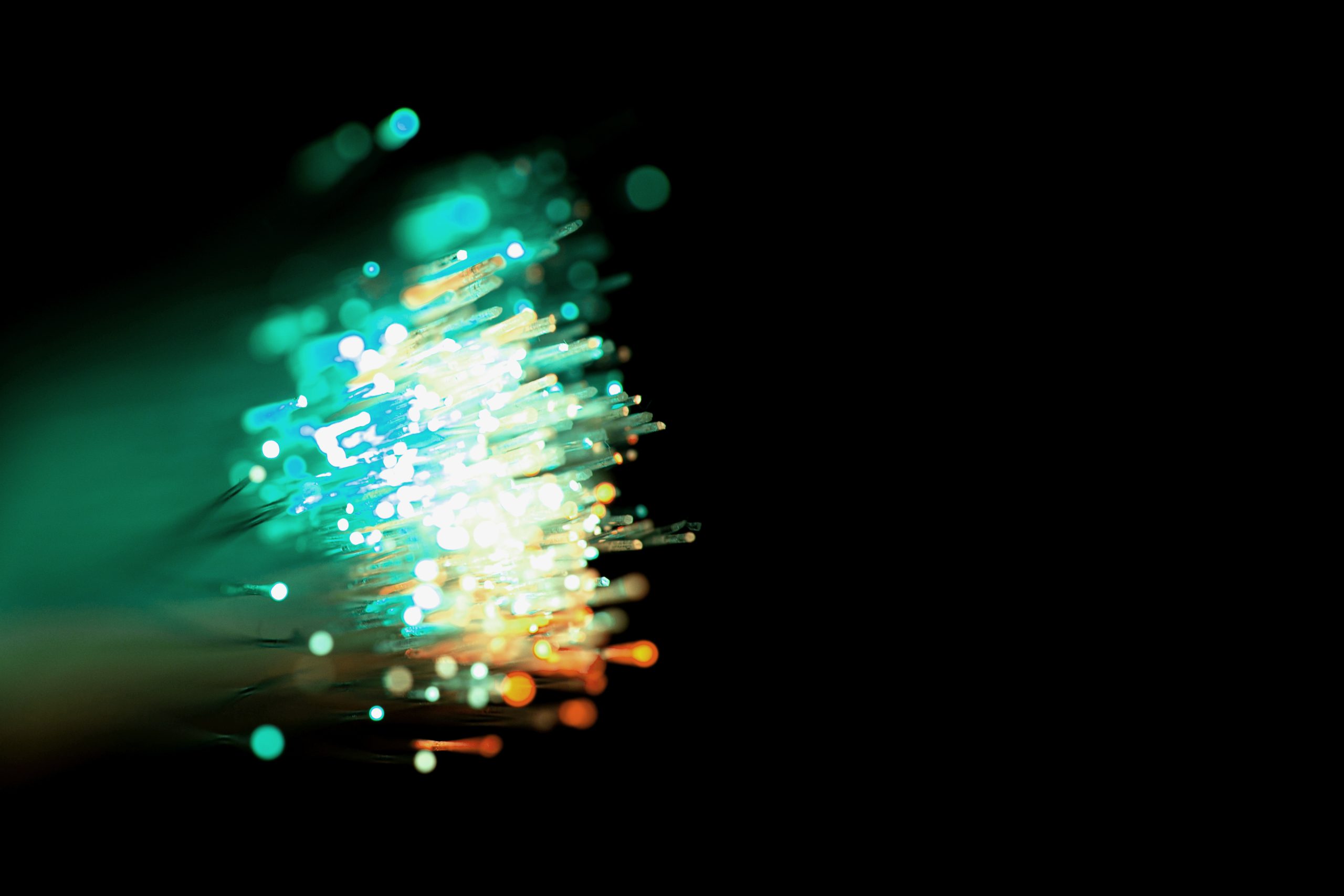
The Role of Apache Spark in Big Data Analytics
In today’s data-driven world, businesses rely on big data analytics to make smarter decisions. However, processing massive datasets quickly and efficiently remains a challenge. That’s where Apache Spark comes in—a powerful tool that is transforming how we manage big data.
In this article, you’ll learn:
-
What Apache Spark is and why it’s essential for big data analytics
-
The key benefits of using Spark for data processing
-
Real-world applications and how Spark drives innovation
Let’s dive in!
What Is Apache Spark?
Apache Spark is an open-source, distributed computing system designed for fast and flexible data processing. It handles large-scale analytics tasks with ease, making it a top choice for businesses and data scientists alike.
Unlike traditional tools, Spark processes data in memory, which drastically speeds up computations. Moreover, it supports multiple programming languages such as Python, Java, Scala, and R—enhancing accessibility across technical teams.
Learn more on the official Apache Spark website
Why Apache Spark Is Crucial for Big Data Analytics
1. Speed and Efficiency
Thanks to its in-memory processing capabilities, Spark performs tasks up to 100x faster than older systems like Hadoop MapReduce. This is crucial for real-time analytics and data-driven decision-making.
2. Versatility
Apache Spark supports multiple data processing models, including:
-
Batch processing (for large datasets)
-
Real-time data streaming
-
Machine learning
-
Graph processing
This versatility enables organizations to handle various data needs using a single platform.
3. Scalability
As your data grows, Spark can scale effortlessly—from a single server to thousands of machines. This flexibility ensures it can support businesses of any size.
Key Benefits of Using Apache Spark
Faster Data Processing
By processing data in memory and minimizing disk I/O operations, Spark delivers lightning-fast analytics—even on massive datasets.
Ease of Use
Spark offers APIs for Python (PySpark), Java, Scala, and R. As a result, data scientists and developers can work with the tools they already know—without a steep learning curve.
Advanced Analytics Capabilities
Spark comes with powerful libraries:
-
MLlib for machine learning
-
GraphX for graph processing
-
Spark Streaming for real-time data streams
These libraries empower users to perform complex analytics without needing separate platforms.
Real-World Applications of Apache Spark
1. Financial Services
Banks and financial institutions use Spark to detect fraud in real-time. By analyzing transaction patterns, they can flag suspicious activities instantly—helping prevent financial crimes.
2. Healthcare
In healthcare, Spark processes vast amounts of patient data to predict diseases and improve treatment plans. As a result, patient outcomes are significantly enhanced.
3. E-Commerce
Online retailers leverage Spark to personalize shopping experiences. By analyzing user behavior, they offer relevant product recommendations that improve conversion rates.
How Apache Spark Compares to Other Big Data Tools
Spark vs. Hadoop
While Hadoop is excellent for batch processing, Spark outshines it in speed and flexibility. Interestingly, Spark can even run on top of Hadoop, allowing organizations to get the best of both worlds.
Spark vs. Flink
Both tools support real-time processing, but Spark’s mature ecosystem and ease of integration make it more attractive for many businesses.
Getting Started
Step 1: Set Up Your Environment
You can install Spark locally or use managed platforms like AWS EMR, Azure HDInsight, or Databricks for a quicker setup.
Step 2: Learn the Basics
Start by writing simple programs to filter, sort, and aggregate data. The official Spark documentation offers excellent tutorials.
Step 3: Explore Advanced Features
As you become more comfortable, dive into Spark’s machine learning and streaming modules to unlock its full potential.
Conclusion
Apache Spark is a game-changer in big data analytics. Its speed, versatility, and scalability make it an essential tool for modern businesses.
Whether you’re analyzing financial transactions, improving healthcare services, or customizing shopping experiences, Spark helps you achieve better results faster.
Ready to explore Spark? Get started today and transform your data analytics strategy.
FAQs
1. What is Apache Spark used for?
Apache Spark is used for big data processing, real-time analytics, machine learning, and graph processing.
2. Is Apache Spark better than Hadoop?
Spark is faster and more versatile, especially for real-time data processing. However, both can work together effectively.
3. Can I use Apache Spark for small datasets?
Yes, though Spark is built for big data, it can efficiently process small datasets as well.
4. What programming languages does Spark support?
Spark supports Python, Java, Scala, and R.
5. Is Apache Spark difficult to learn?
Not really. If you’re familiar with programming, Spark’s user-friendly APIs and thorough documentation make it relatively easy to learn.
You can find more about Data analytics here.
Author Profile
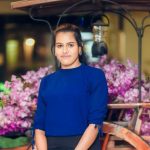
- Online Media & PR Strategist
- Hello there! I'm Online Media & PR Strategist at NeticSpace | Passionate Journalist, Blogger, and SEO Specialist
Latest entries
Data AnalyticsJune 13, 2025Future of Data Warehousing in Big Data
AI InterfaceJune 13, 2025Aligning AI Developments with Corporate Goals in the AI Era
HPC and AIJune 13, 2025HPC Architecture Taking to the Next Level
Quantum ComputingJune 13, 2025Ethical Issues in Quantum Tech: Privacy, Jobs, and Policy