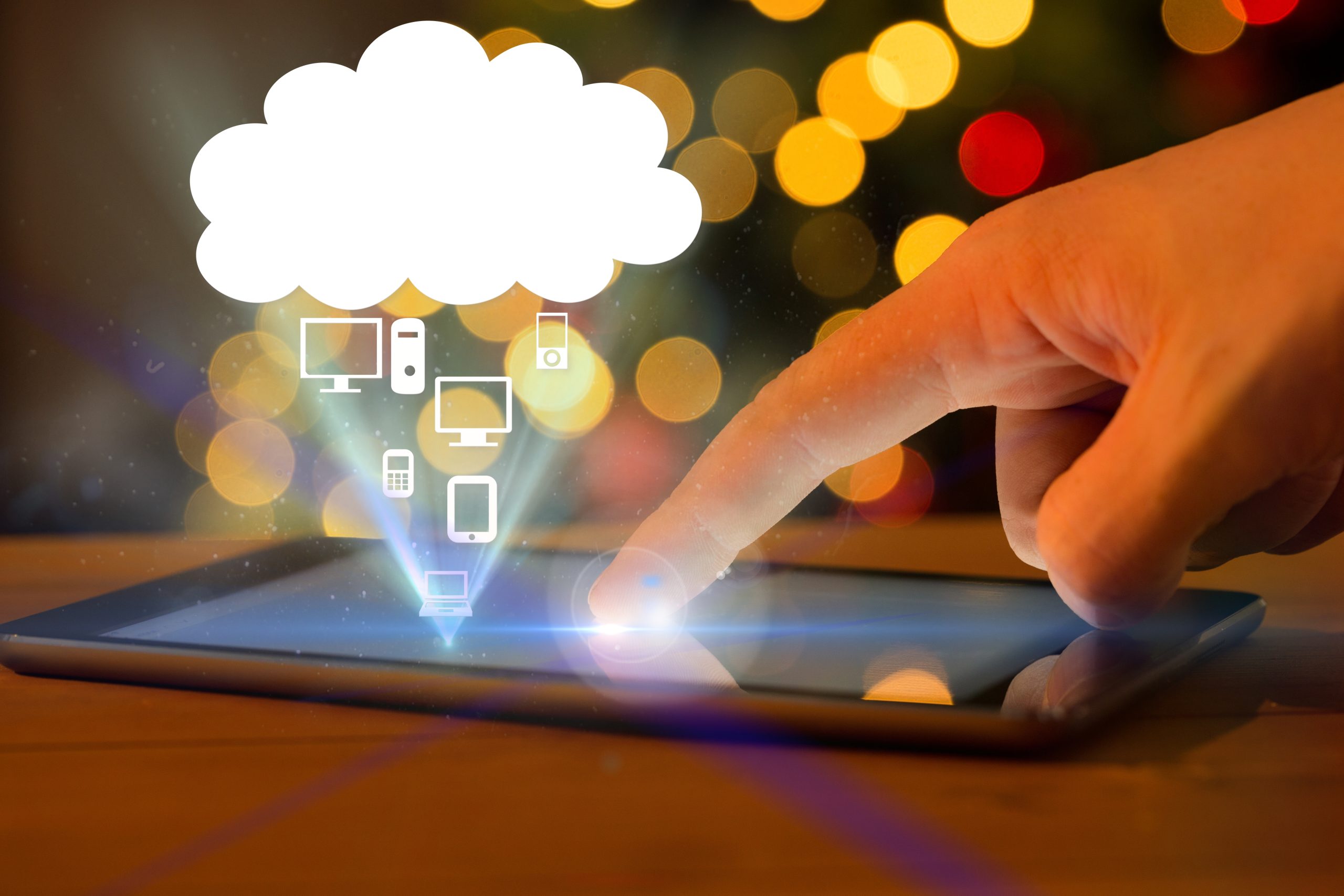
The Challenges of Multi-Cloud MLOps and How to Solve Them
Machine Learning Operations (MLOps) is essential for managing machine learning (ML) workflows at scale. However, as businesses adopt multi-cloud MLOps, they face new challenges that can hinder performance, security, and scalability. In this article, we’ll explore these challenges and practical solutions to overcome them.
What is Multi-Cloud MLOps?
Multi-cloud MLOps refers to the practice of deploying and managing ML models across multiple cloud providers, such as AWS, Azure, and Google Cloud. This approach helps organizations avoid vendor lock-in, enhance redundancy, and optimize costs. However, it also introduces complexity in integration, security, and compliance.
Key Challenges of Multi-Cloud MLOps (And How to Solve Them)
1. Integration Complexity
The Challenge:
Each cloud provider has its own set of tools, APIs, and ML services. Integrating these platforms can lead to inconsistencies in data pipelines and model deployment.
The Solution:
- Use containerization with Docker and Kubernetes to create portable ML environments.
- Implement multi-cloud orchestration tools like Apache Airflow or Kubeflow.
- Standardize on open-source ML frameworks like TensorFlow, PyTorch, and ONNX to ensure cross-cloud compatibility.
2. Data Governance and Security
The Challenge:
Handling data security, access control, and compliance across multiple clouds can be challenging, especially with regulations like GDPR and HIPAA.
The Solution:
- Adopt data encryption for both in-transit and at-rest data.
- Utilize identity and access management (IAM) solutions like AWS IAM, Azure AD, and Google IAM.
- Implement federated security models to maintain consistent security policies across platforms.
3. Cost Management
The Challenge:
Multi-cloud environments often lead to unpredictable costs due to differences in pricing models, data transfer fees, and resource utilization.
The Solution:
- Use cost monitoring tools like AWS Cost Explorer, Google Cloud Pricing Calculator, and Azure Cost Management.
- Set up auto-scaling and resource allocation policies to avoid over-provisioning.
- Optimize ML workloads using spot instances and reserved pricing plans where applicable.
4. Latency and Performance Optimization
The Challenge:
Running ML workloads across different clouds can introduce latency issues, affecting real-time inference and training efficiency.
The Solution:
- Deploy models closer to data sources using edge computing solutions.
- Use CDNs and hybrid cloud setups to reduce inter-cloud latency.
- Optimize model architectures with quantization and pruning techniques to enhance inference speed.
5. Monitoring and Logging Across Clouds
The Challenge:
Tracking ML models across multiple cloud providers requires a centralized monitoring and logging system.
The Solution:
- Implement unified logging frameworks like ELK Stack (Elasticsearch, Logstash, Kibana) or Prometheus.
- Use ML model monitoring tools such as MLflow, Weights & Biases, or TensorBoard.
- Enable automated anomaly detection using AI-driven observability platforms.
Best Practices for Multi-Cloud MLOps
To streamline MLOps across multiple cloud providers, follow these best practices:
- Adopt Infrastructure-as-Code (IaC): Use Terraform or CloudFormation for consistent cloud provisioning.
- Leverage API Gateways: Standardize API endpoints to manage model deployment seamlessly.
- Prioritize Interoperability: Choose ML tools that support cross-cloud deployments.
- Implement CI/CD Pipelines: Automate model training, testing, and deployment with Jenkins, GitHub Actions, or GitLab CI/CD.
Frequently Asked Questions (FAQs)
1. Why use multiple cloud providers for MLOps?
Using multiple cloud providers helps organizations reduce dependency on a single vendor, improve uptime, and take advantage of cost savings and best-in-class services from different providers.
2. How do you ensure data consistency in multi-cloud MLOps?
Data consistency can be maintained using distributed databases, data lakes, and cloud-agnostic storage solutions like Apache Kafka or Delta Lake.
3. What are the best tools for managing MLOps across multiple clouds?
Some of the best tools include Kubeflow, MLflow, TensorFlow Extended (TFX), and Apache Airflow.
4. How do you secure machine learning workloads in multi-cloud environments?
Security best practices include role-based access control (RBAC), encryption, and federated identity management.
5. How do you monitor machine learning models across different cloud platforms?
Use centralized logging, monitoring dashboards, and AI-driven observability tools like Datadog or Prometheus.
Author Profile
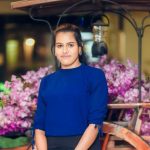
- Online Media & PR Strategist
- Hello there! I'm Online Media & PR Strategist at NeticSpace | Passionate Journalist, Blogger, and SEO Specialist
Latest entries
Scientific VisualizationApril 30, 2025Deepfake Scientific Data: AI-Generated Fraud in Research
Data AnalyticsApril 30, 2025What Is Data Mesh Architecture and Why It’s Trending
Rendering and VisualizationApril 30, 2025Metaverse Rendering Challenges and Opportunities
MLOpsApril 30, 2025MLOps 2.0: The Future of Machine Learning Operations