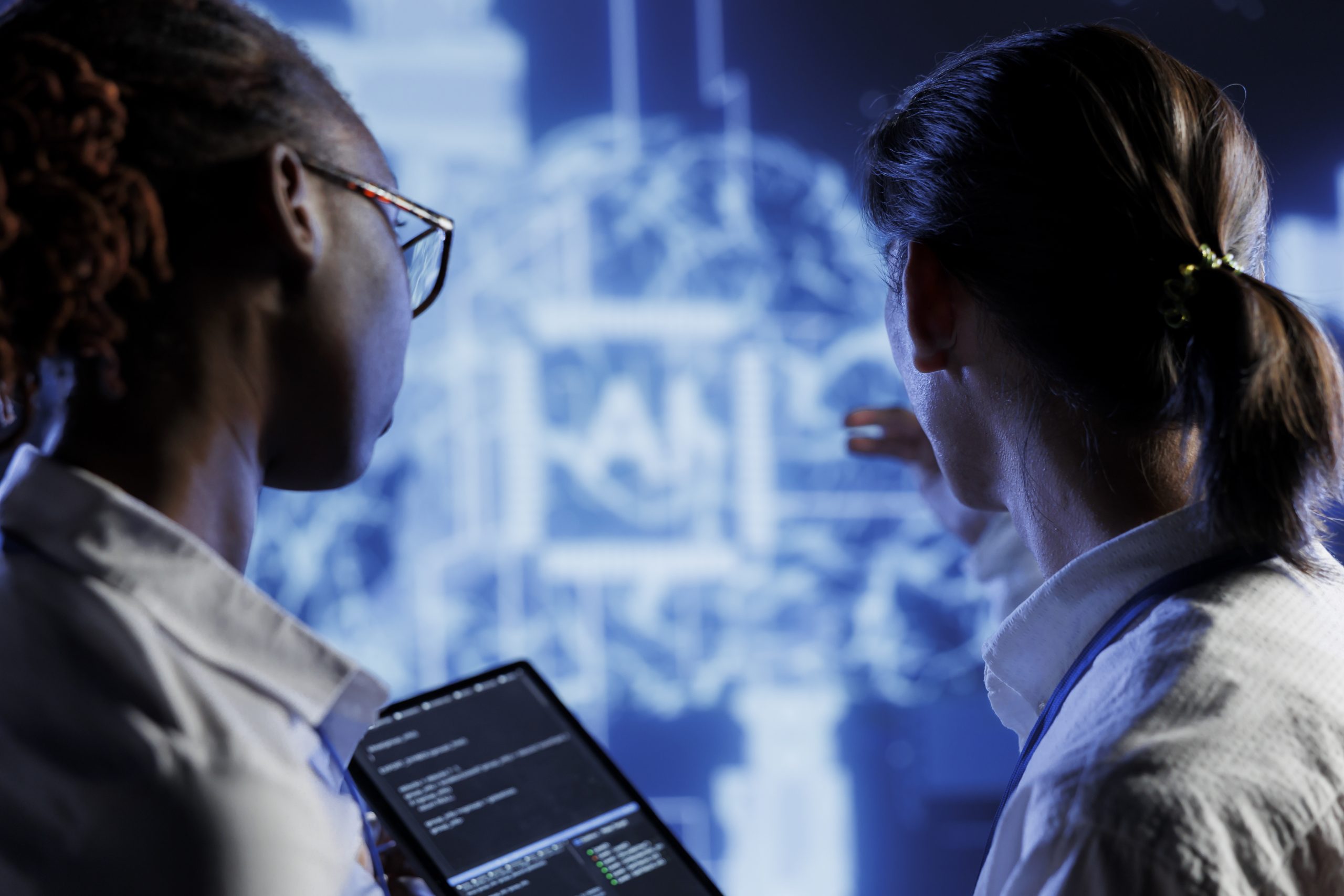
Scaling MLOps with Kubernetes and Kubeflow Pipelines
Machine learning models are growing in complexity. Teams must move faster to stay competitive. That’s where MLOps comes in. It blends development and operations to streamline machine learning workflows.
In this blog post, you’ll learn how Kubernetes and Kubeflow Pipelines help you scale MLOps. We’ll explore container orchestration, training, inference, and best practices. By the end, you’ll have a solid grasp of how to deploy and manage ML systems at scale.
What Is MLOps and Why Does It Matter?
MLOps stands for Machine Learning Operations. It merges machine learning development with production operations. This approach ensures smooth collaboration between data scientists, ML engineers, and DevOps teams.
Speed is crucial in ML projects. Models must be deployed and updated quickly. MLOps gives you standardized processes to automate these steps. This saves time, reduces errors, and boosts reliability.
The Power of Kubernetes in MLOps
Kubernetes simplifies container orchestration. It automates deployment, scaling, and management of containerized services. This is vital for machine learning workloads that often require different environments.
First, Kubernetes offers self-healing features. If a container fails, Kubernetes restarts it. This feature ensures your ML services remain up and running.
Next, Kubernetes supports horizontal scaling. You can add more container replicas to handle more load. This is especially important for heavy training jobs and real-time inference.
Finally, Kubernetes helps standardize your infrastructure. It provides consistent environments for data processing, model training, and serving. This consistency is the core of MLOps success.
Getting Started with Kubeflow Pipelines
Kubeflow Pipelines is a platform for building and deploying ML workflows on Kubernetes. It ties together steps like data preprocessing, model training, and model evaluation. Each step is a pipeline component that you can reuse.
First, you define a pipeline using Python code or a graphical UI. This pipeline describes the sequence of components. Kubeflow Pipelines automatically handles container orchestration for each part of the workflow.
Next, you can track metrics and artifacts within the Kubeflow Pipelines dashboard. This is helpful for debugging or comparing model versions. It creates a central place to watch your entire ML process.
Building Blocks of Container Orchestration
Container orchestration is the practice of managing multiple containers. It handles scheduling, scaling, and networking. Kubernetes is the most popular tool for container orchestration in ML.
Below are key elements of container orchestration:
- Pods: The smallest unit in Kubernetes. Each pod holds one or more containers.
- Services: Define how pods are accessed. They load-balance requests across pod replicas.
- Deployments: Control the creation and scaling of pods. They help keep your application up to date.
- ConfigMaps and Secrets: Store configuration data and sensitive information. They keep your containers stateless.
Using these building blocks, you can design scalable ML systems. Each model training job and inference service can be defined as a series of pods, deployments, and services. Kubernetes ensures that resources are allocated efficiently.
Setting Up Kubernetes for Machine Learning
Before deploying ML services, you need a Kubernetes cluster. You can use cloud platforms like Google Kubernetes Engine (GKE), Amazon EKS, or Azure Kubernetes Service (AKS). You can also set up a local cluster using Minikube.
First, confirm that your cluster is large enough for your workloads. Training can be CPU- or GPU-intensive. You can enable GPU support by selecting instances that include GPU hardware.
Next, install Kubeflow or Kubeflow Pipelines on top of your cluster. The Kubeflow project provides scripts and documentation to guide you. This step configures the pipeline components for your environment.
Finally, secure your cluster. Use authentication and role-based access control (RBAC) to limit user permissions. This prevents unauthorized access to your ML pipelines and data.
Scaling Training with Kubernetes
Training ML models is resource-intensive. Kubernetes helps you manage resources effectively. You can define resource requests and limits for each training job. This ensures no single job can starve others of CPU or memory.
Distributing Training Jobs
Some ML frameworks, like TensorFlow or PyTorch, enable distributed training. You can spread training across multiple pods. Each pod runs a part of the training process. This speeds up model development.
- Data Parallelism: Split your dataset into batches across multiple workers.
- Model Parallelism: Split different parts of the model architecture across multiple workers.
- Parameter Servers: Maintain shared parameters for the model. Workers update these parameters in sync.
Auto-Scaling Training Jobs
Kubernetes supports custom auto-scaling. When CPU or GPU usage is high, more pod replicas are created. This is possible through the Horizontal Pod Autoscaler (HPA). You can also scale down pods during idle periods, saving money and resources.
Scaling Inference with Kubernetes
Inference is the process of using a trained model to make predictions. It often needs rapid response times. Kubernetes makes it easy to scale your inference services based on real-time demand.
Containerizing Inference Services
Wrap your model in a Docker container. This container includes the trained model, inference code, and dependencies. After packaging, you can deploy this container to your Kubernetes cluster.
Enabling Load Balancing
Use Kubernetes services to load-balance incoming requests. The service redirects traffic to the available pod replicas. If traffic surges, you can auto-scale the pods to handle the increased load.
Canary Releases and Rolling Updates
When updating a model, you want to test it in a low-risk way. With canary releases, you direct a small percentage of traffic to the new model. If results look good, you expand it to more users. Rolling updates help replace old pods with new ones without downtime.
Monitoring and Optimization
Monitoring is crucial for spotting issues. Tools like Prometheus and Grafana integrate with Kubernetes to track metrics. You can watch CPU, memory, GPU usage, and custom ML metrics.
First, set up alerts for abnormal resource usage or service downtime. This helps you respond quickly to problems. Next, examine logs from pods to debug training or inference issues. Logging tools like Elasticsearch or Fluentd can gather logs in a central place.
Finally, fine-tune your ML pipelines. Check how long each pipeline step takes. Optimize container images by removing unnecessary packages. Reduce overhead by using lightweight base images. Focus on continuous improvement to keep costs low and performance high.
Best Practices for Production Environments
Version Control and Reproducibility
Use Git for version control of pipeline definitions. Store model artifacts with a clear naming scheme. This makes it easy to roll back to a previous model if needed. Reproduce your results by keeping environment details in Docker images and pipeline components.
Security and Access Control
Employ role-based access control (RBAC) to limit who can deploy, modify, or view ML pipelines. Encrypt sensitive data using Secrets. Scan container images for vulnerabilities before deployment.
Infrastructure as Code
Manage your Kubernetes configurations with tools like Helm or Terraform. Keep these files in a repository for quick redeployments. This approach ensures consistency across development, testing, and production environments.
Continuous Integration and Deployment (CI/CD)
Automate model building, testing, and deployment with a CI/CD pipeline. Tools like Jenkins or GitLab CI can trigger pipeline runs after code changes. This reduces manual work and speeds up new feature releases.
Conclusion
Kubernetes and Kubeflow Pipelines make scaling MLOps more efficient. They unify the development, training, and production stages of machine learning. Their built-in orchestration, auto-scaling, and monitoring features improve model reliability.
By following best practices, you can optimize training and inference workflows. You can also tighten security and resource usage. Overall, integrating Kubernetes with Kubeflow Pipelines is a robust strategy for managing growing ML demands.
Now is the time to explore these tools in depth. Start with a small proof-of-concept. Then expand to a larger production environment. With Kubernetes and Kubeflow Pipelines, you’ll be ready for the next wave of machine learning challenges.
Author Profile
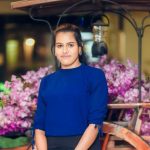
- Online Media & PR Strategist
- Hello there! I'm Online Media & PR Strategist at NeticSpace | Passionate Journalist, Blogger, and SEO Specialist
Latest entries
Digital Twin DevelopmentApril 30, 2025How to Ensure Data Synchronization Twins Effectively
Scientific VisualizationApril 30, 2025Deepfake Scientific Data: AI-Generated Fraud in Research
Data AnalyticsApril 30, 2025What Is Data Mesh Architecture and Why It’s Trending
Rendering and VisualizationApril 30, 2025Metaverse Rendering Challenges and Opportunities