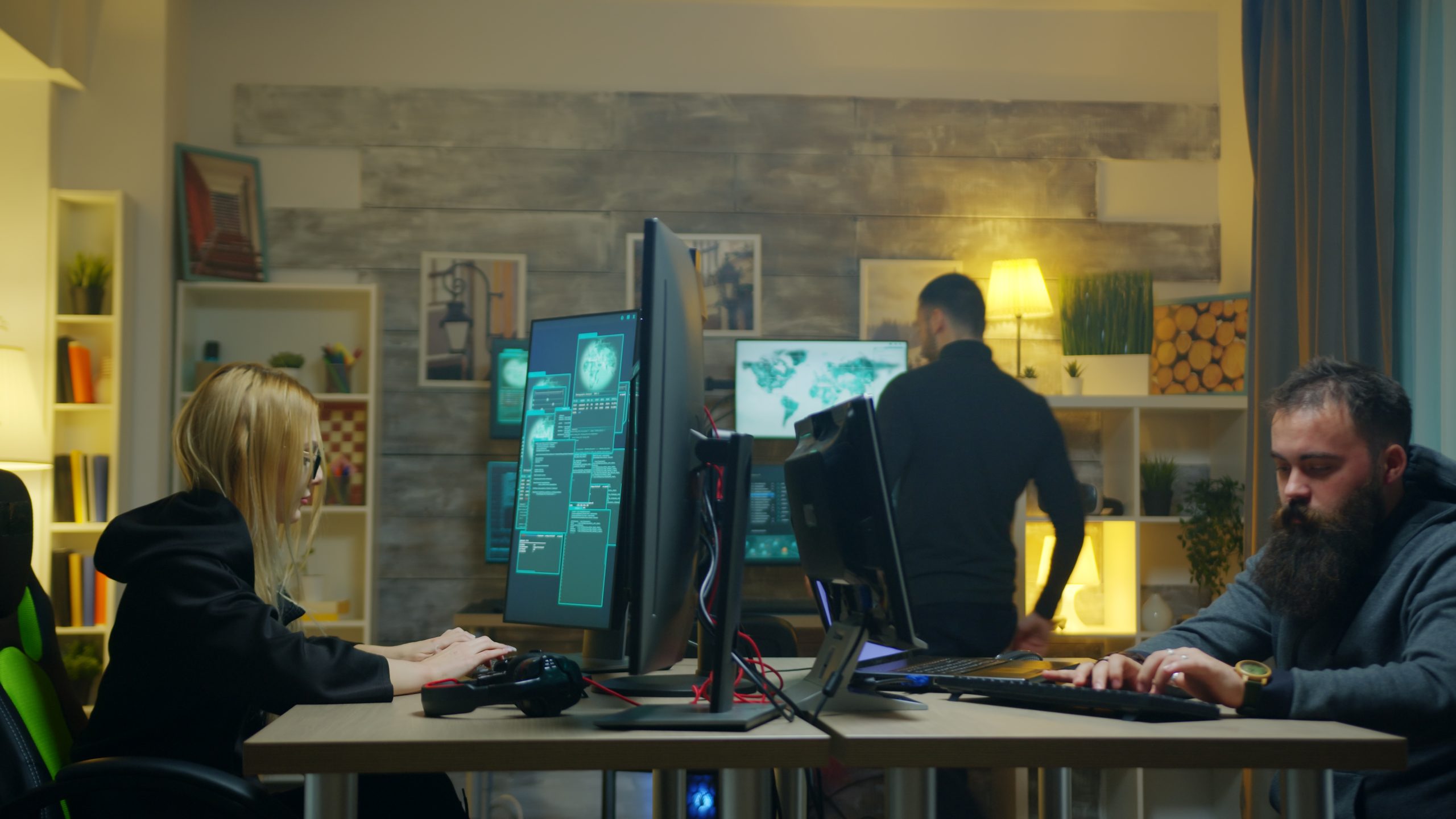
Scaling HPC Workloads: Strategies and Challenges
Learn practical tips for HPC scaling to handle bigger clusters, balance workloads, and optimize performance under heavy loads.
High-Performance Computing (HPC) systems handle some of the world’s most demanding tasks. They power weather simulations, scientific research, and data-intensive analytics. Yet, as needs grow, scaling HPC workloads can be daunting. In this post, you will learn practical strategies to scale HPC clusters, balance heavy workloads, and optimize performance.
Why Scaling HPC Matters
Scaling HPC is about meeting bigger demands without losing efficiency. It involves growing your clusters and managing more compute nodes. These systems must share data fast, handle massive workloads, and run complex software. With the right approach, you can meet new challenges and keep performance high.
First, you need clear goals. Do you want faster job completion or more parallel tasks? Next, you should assess your infrastructure. How many nodes can you add before your network slows down? Finally, you have to plan your budget. Each extra node has costs in hardware and power consumption.
Planning for Cluster Growth
Effective scaling begins with smart planning. You must ensure your system’s hardware can expand. This starts with selecting the right compute nodes, storage, and network components. Consider how each piece fits together.
Next, decide on a cluster architecture. Many organizations choose a hierarchical design with dedicated head nodes. Others select a more uniform setup where each node has the same role. Each approach has pros and cons. Plan for redundancy so you can survive node failures without halting your entire operation.
Finally, think about future upgrades. Today’s needs may not match tomorrow’s demands. HPC workloads often grow at a rapid pace. Designing with extra capacity in mind ensures a smoother scaling path.
Balancing Heavy Workloads
Load balancing is key when scaling HPC clusters. It ensures each node works at optimal levels. When tasks are uneven, some nodes sit idle while others face heavy strain.
First, explore different workload scheduling systems. Tools like Slurm or PBS Pro manage job queues. They distribute tasks across available nodes. Next, set priority rules. Some jobs may be time-sensitive while others can wait. A robust policy ensures urgent tasks run first but does not stall long processes.
Finally, monitor your cluster’s workload in real-time. Performance metrics help you spot imbalances. Adjust your approach if certain nodes get overloaded. Load balancing is a continuous process, not a one-time setup.
Optimizing HPC Performance
Optimal performance depends on careful tuning. Even small changes can yield big speedups. Focus on improving data transfer, node communication, and software efficiency.
- Network Tuning: Use high-bandwidth interconnects like InfiniBand. Reduce latency by fine-tuning your network protocols.
- Parallel I/O: Store data in parallel file systems. This ensures reading and writing large data sets won’t bottleneck your system.
- Code Profiling: Use tools like Intel VTune or NVIDIA Nsight to spot slow code sections. Optimize them for better performance.
Next, verify that your cluster’s operating system is up to date. System updates often include new drivers and kernel improvements. Sometimes, a small patch can solve performance issues. Finally, think about specialized accelerators. GPUs or FPGAs can speed up specific tasks.
Overcoming Common Challenges
Scaling HPC isn’t just about adding more nodes. With growth comes new challenges. One major issue is communication overhead. More nodes mean more network traffic. This can slow down large-scale computations.
Another challenge is fault tolerance. Bigger clusters have more potential points of failure. You need robust strategies to handle hardware faults. Automated recovery can reroute tasks to healthy nodes. This avoids long downtimes.
Finally, power and cooling can be costly. Extra nodes consume more electricity. They also produce more heat. Plan for an efficient cooling system. This can save money in the long run. Some data centers use liquid cooling to keep hardware at safe temperatures.
Tools and Technologies for Scaling
When it comes to scaling HPC workloads, technology matters. Several tools can help manage clusters and keep them running smoothly. Below are a few must-haves:
- Resource Managers: Slurm, PBS Pro, and Grid Engine track and schedule jobs. They split tasks across nodes and monitor resource usage.
- MPI Libraries: OpenMPI and MPICH enable message passing between nodes. Proper use of MPI is crucial for parallel programs.
- Containers: Singularity and Docker can simplify software deployment. They let you package dependencies and ensure consistent environments across nodes.
- Monitoring Suites: Prometheus or Grafana visualize cluster performance. These tools send alerts if your system nears critical load.
Next, explore specialized hardware. Some tasks benefit from GPU computing. Others need high-speed interconnects like Omni-Path or InfiniBand. Match your hardware to your workloads for the best results.
Best Practices for Large HPC Clusters
Large HPC clusters have unique needs. Follow these best practices:
- Regular Upgrades: Keep hardware and software up to date. This reduces security risks and improves performance.
- Detailed Documentation: Maintain clear records of cluster configurations. Document changes, node specs, and network settings.
- Proactive Maintenance: Replace aging components before they fail. This avoids downtime and costly repairs.
- User Training: Teach your team HPC best practices. Trained users submit more efficient jobs and report issues faster.
Finally, automate where possible. Automated workflows cut down on manual tasks. This allows administrators to focus on bigger goals.
Evaluating Scalability
Scalability is about how well performance grows when adding more resources. To measure it, run benchmarks and track performance metrics. Common HPC benchmarks include High-Performance Linpack (HPL) and HPCG.
First, test small cluster sizes. Record baseline numbers. Next, add nodes in increments. See how performance scales. If each new node yields proportional speed gains, your system scales well. If not, look for bottlenecks in network or I/O. Document these results to guide future improvements.
Budget and Resource Management
Scaling HPC systems involves costs. More nodes mean higher electricity bills and hardware expenses. You also need to manage space in your data center. If you run HPC in the cloud, plan for usage spikes. Cloud instances can be spun up fast, but they can also drive up monthly bills if not managed well.
Next, consider open-source vs. commercial software. Open-source tools often reduce licensing fees. Commercial tools, however, may provide advanced features or dedicated support. Weigh the cost and benefits before choosing a solution.
Finally, plan a resource allocation policy. Decide how to share resources among different departments or projects. This avoids conflict and ensures fair usage.
Monitoring and Troubleshooting
When HPC clusters grow, problems can occur at any time. Network errors, hardware failures, and software bugs all disrupt workloads. A proper monitoring system is your first line of defense. Tools like Nagios or Zabbix can keep tabs on node health.
If you spot issues, respond quickly. Inspect system logs to find patterns. Update configurations or replace faulty hardware. Keeping a root cause analysis (RCA) record helps prevent the same issue from recurring. A well-documented troubleshooting process saves time and resources.
Future Trends in HPC Scaling
The HPC world evolves constantly. New technologies like quantum computing and ARM-based architectures are on the horizon. Some organizations are exploring liquid cooling and carbon-neutral power to tackle energy costs. Others are embracing cloud bursting, which merges on-premises clusters with cloud resources for flexible scaling.
Keeping up with these trends ensures long-term success. HPC is not just about raw compute anymore. It’s about efficiency, adaptability, and sustainability.
Conclusion
Scaling HPC workloads is both an art and a science. You need to plan for cluster growth, balance heavy jobs, and fine-tune performance. You also face real challenges, from network congestion to rising power costs. Yet, with smart strategies, robust tools, and future-proof planning, you can meet your growing HPC demands.
As you scale, pay attention to metrics, maintain documentation, and update your systems. By staying proactive, you will overcome hurdles and unlock new achievements in High-Performance Computing.
Author Profile
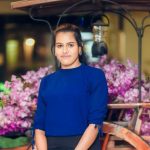
- Online Media & PR Strategist
- Hello there! I'm Online Media & PR Strategist at NeticSpace | Passionate Journalist, Blogger, and SEO Specialist
Latest entries
Digital Twin DevelopmentApril 30, 2025How to Ensure Data Synchronization Twins Effectively
Scientific VisualizationApril 30, 2025Deepfake Scientific Data: AI-Generated Fraud in Research
Data AnalyticsApril 30, 2025What Is Data Mesh Architecture and Why It’s Trending
Rendering and VisualizationApril 30, 2025Metaverse Rendering Challenges and Opportunities