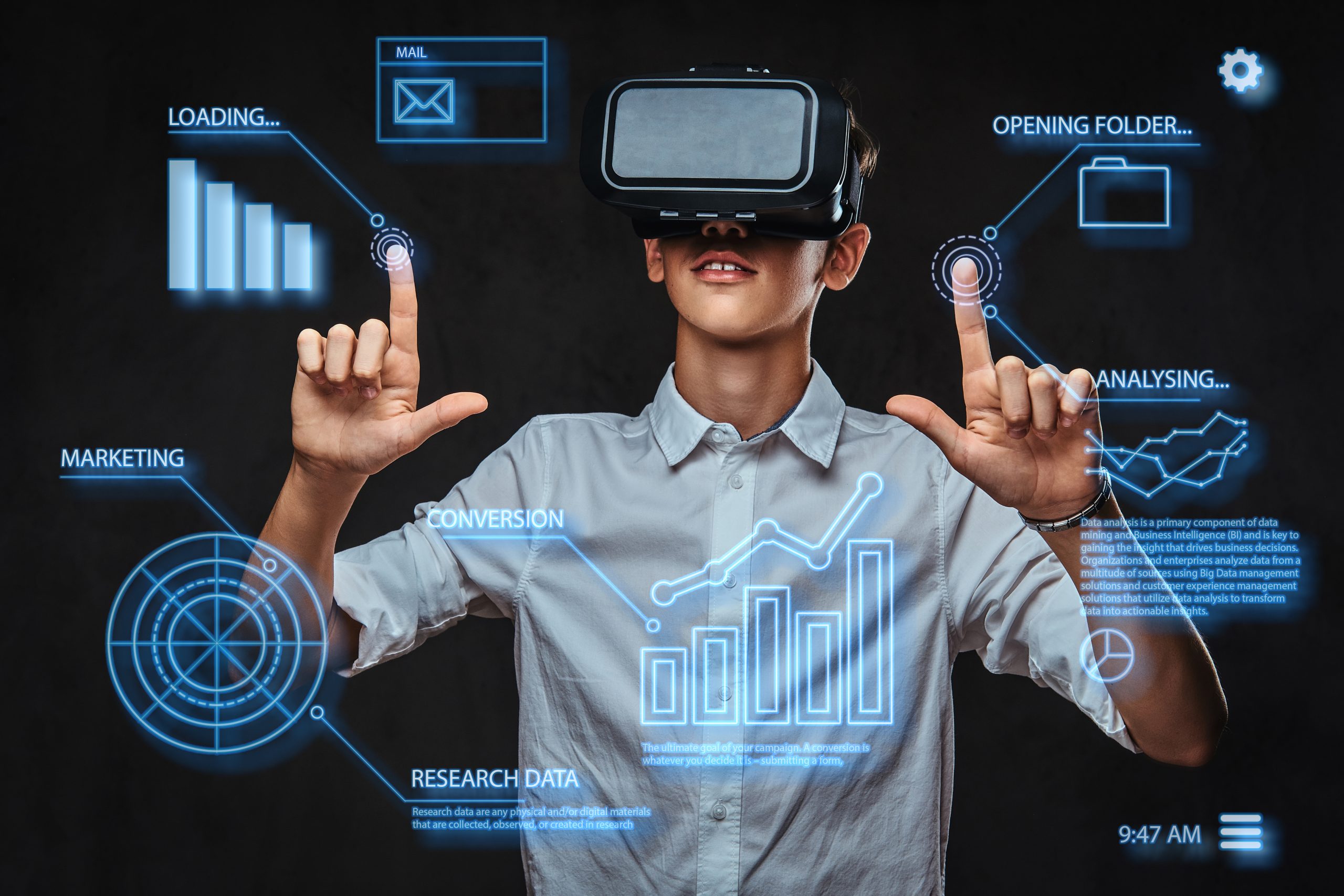
Managing Feature Stores in MLOps: A Guide to Data Consistency and Scalability
Learn how feature stores improve MLOps workflows, ensuring data consistency between training and inference. Explore centralized vs. distributed architectures and top tools like Feast, Tecton, and Databricks.
Why Feature Stores Matter in MLOps
Machine learning models are only as good as the data they use. One major challenge in MLOps is maintaining data consistency between training and inference. Without a structured system, feature engineering can become a bottleneck, leading to duplicated efforts, versioning issues, and unreliable predictions.
This is where feature stores come in. They provide a centralized repository for storing, managing, and serving machine learning features, making MLOps workflows more efficient and scalable.
In this guide, we’ll cover:
- What a feature store is and why it’s essential.
- Centralized vs. distributed feature store architectures.
- Popular feature store solutions (Feast, Tecton, Databricks).
- How feature stores ensure data consistency across training and inference.
What Is a Feature Store?
A feature store is a system for managing and serving machine learning features in a consistent and scalable way. It helps MLOps teams by:
- Standardizing feature engineering across teams.
- Storing precomputed features to reduce real-time computation.
- Ensuring consistency between training and inference data.
- Improving efficiency by enabling feature reuse across multiple models.
Why Are Feature Stores Important?
Without a feature store, teams often write redundant code, leading to inconsistencies between training and inference. This can cause model drift and unreliable predictions.
A feature store solves this by acting as a single source of truth for machine learning features, ensuring consistency and improving model performance.
Centralized vs. Distributed Feature Store Architectures
Feature stores can be designed using two main architectures: centralized and distributed. Each has its own benefits and trade-offs.
1. Centralized Feature Store
A centralized feature store is a single system where all feature data is stored and served from a central repository.
Advantages
- Simplifies feature management by providing a single location for all features.
- Ensures consistency since all teams access the same feature definitions.
- Improves governance by enforcing data quality and compliance rules.
Challenges
- Scalability limits as the system grows and handles more models.
- Latency issues in real-time applications due to network dependencies.
2. Distributed Feature Store
A distributed feature store spreads feature storage and serving across multiple locations, often closer to where the models are deployed.
Advantages
- Scales better for large, complex systems.
- Reduces latency by storing features near inference points.
- Increases resilience since failures in one part of the system won’t affect the entire store.
Challenges
- Harder to manage due to decentralized control.
- Potential consistency issues if not synchronized properly.
Choosing between centralized and distributed architectures depends on factors like model complexity, scalability needs, and real-time processing requirements.
Popular Feature Store Solutions
Several feature store solutions exist, each designed to streamline feature management in MLOps. Here are three widely used options:
1. Feast
Feast (Feature Store for ML) is an open-source feature store designed for both batch and real-time machine learning.
Key Features:
- Supports both batch and real-time feature serving.
- Works with various data sources like BigQuery, Redis, and DynamoDB.
- Can be integrated with existing MLOps pipelines.
Best For: Teams looking for a flexible, open-source feature store with real-time capabilities.
2. Tecton
Tecton is a fully managed feature store designed for enterprise MLOps teams.
Key Features:
- Provides automated feature pipelines for real-time and batch processing.
- Ensures feature consistency across training and inference.
- Integrates with cloud-native MLOps tools.
Best For: Large teams that need a managed, production-grade feature store.
3. Databricks Feature Store
Databricks Feature Store is built into Databricks’ Lakehouse Platform, offering seamless integration with Spark-based workflows.
Key Features:
- Deep integration with Databricks and MLflow.
- Supports batch and real-time feature storage.
- Provides automated lineage tracking for better governance.
Best For: Organizations using Databricks for data processing and ML model development.
How Feature Stores Ensure Data Consistency Across Training and Inference
One of the biggest challenges in MLOps is ensuring that features used in training are identical to those used in inference. Without this, models can make inaccurate predictions.
Feature stores solve this problem in several ways:
1. Unified Feature Definitions
Feature stores provide a single source of truth for feature definitions. This ensures models always use the same calculations, regardless of whether they are in training or production.
2. Automated Data Versioning
By versioning features, a feature store ensures that models trained on older data can still access the same feature values during inference.
3. Consistent Feature Serving
Feature stores precompute and store features so they can be served in real time without discrepancies. This avoids situations where training data is outdated compared to inference data.
4. Monitoring and Governance
Most feature stores include monitoring tools to track feature drift, detect data quality issues, and enforce governance policies.
Why Feature Stores Are Essential for MLOps
Feature stores play a critical role in modern MLOps by ensuring data consistency, improving efficiency, and reducing redundant feature engineering.
To recap:
- Feature stores store and serve machine learning features for reuse.
- They come in centralized and distributed architectures, each with its own benefits.
- Popular solutions include Feast, Tecton, and Databricks Feature Store.
- Feature stores ensure consistency between training and inference, reducing model drift.
As machine learning continues to scale, adopting a feature store is a key step toward making MLOps workflows more reliable and efficient.
If your team struggles with managing features, consider implementing a feature store today to streamline your MLOps pipeline. 🚀
What’s Next?
Explore different feature store tools, experiment with open-source solutions like Feast, or evaluate managed platforms like Tecton to see which fits your workflow best.
FAQ
1. What is the difference between a feature store and a data warehouse?
A feature store is optimized for machine learning features, ensuring consistency and serving features in real time. A data warehouse stores large datasets for analytics but lacks ML-specific capabilities.
2. Can I build my own feature store?
Yes, but it requires significant engineering effort to ensure scalability, consistency, and low-latency serving. Most teams prefer using existing solutions.
3. How do feature stores handle real-time inference?
Feature stores like Feast and Tecton support real-time feature serving by precomputing and caching features for low-latency access.
Author Profile
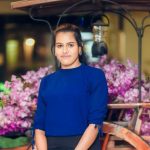
- Online Media & PR Strategist
- Hello there! I'm Online Media & PR Strategist at NeticSpace | Passionate Journalist, Blogger, and SEO Specialist
Latest entries
Robotics SimulationJune 27, 2025Why Accuracy in Robotics Simulation Is Critical Today
HPC and AIJune 27, 2025The Powerful IT Backbone Behind Urban Growth
AI PlatformJune 26, 2025AI Platforms Travel: Boost Hospitality Experience with AI
Data AnalyticsJune 23, 2025Navigating Global Asset Management Regulations Effectively