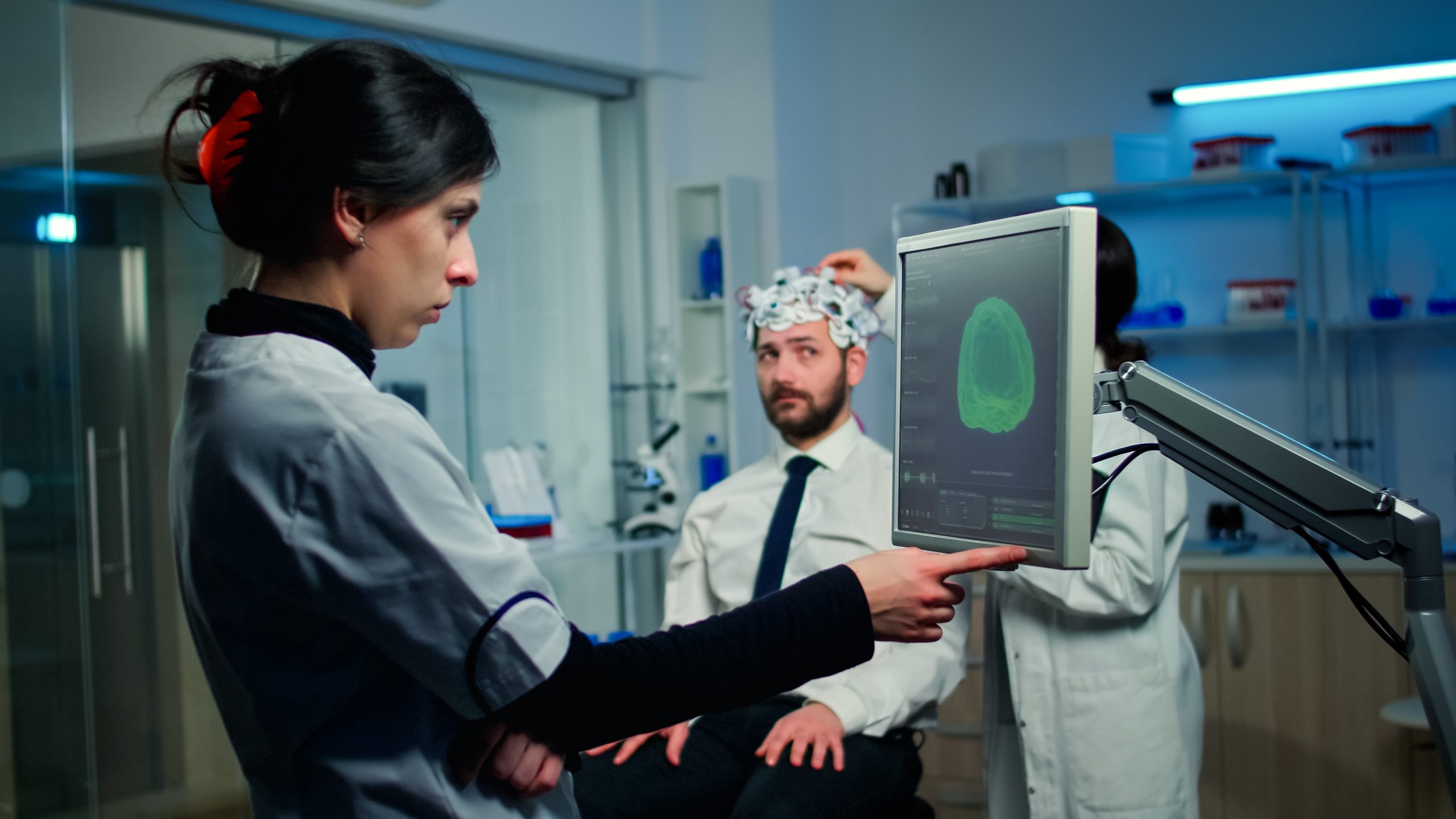
Machine Learning & AI for Automated Scientific Visualization
Machine Learning (ML) and Artificial Intelligence (AI) are transforming scientific visualization. They provide new ways to detect patterns, label complex data, and even generate visual assets automatically. These tools speed up research and help people see insights they might miss otherwise. In this post, you will learn how ML and AI techniques support automated scientific visualization, with real-world examples and best practices.
Why AI Matters for Scientific Visualization
AI-powered methods allow scientists to analyze huge datasets much faster than manual methods. Traditional visualization often relies on human interpretation of complex charts or scans. But high-resolution or multi-dimensional data can overwhelm even the best researchers. ML can sift through this data and point out hidden structures.
First, AI can detect patterns that are not obvious to the naked eye. Next, it can predict new outcomes based on past data trends. Finally, it can generate visual models in real-time. This helps labs, companies, and educational institutions make decisions more quickly.
Basics of Machine Learning and AI
Machine Learning is about teaching computers to learn from examples. Large sets of labeled data guide these computer models. Through training, they recognize patterns or make predictions on new data. AI is a broader term for software that mimics human intelligence, often using ML as a core component.
Many ML tasks are supervised. That means they rely on labeled datasets during training. Others are unsupervised, meaning they discover patterns without labels. Scientists might prefer supervised learning for tasks like image classification. Unsupervised learning might help reveal unknown groupings in massive data logs or sensor readings.
How ML and AI Enhance Automated Visualization
Automated visualization saves time. It also reduces the risk of human error. Below are some ways AI can boost scientific visualization:
- Pattern Detection: ML models can spot trends or anomalies in complex datasets.
- Segmentation: AI can segment regions of interest in images, like tumors in medical scans.
- Automated Asset Generation: ML can create custom 3D models or highlight key parts of large datasets.
- Dynamic Updates: Real-time ML systems can update visualizations as new data arrives.
Next, we will look at each of these in more detail.
Pattern Detection in Complex Data
Scientific datasets often contain millions of data points. Identifying patterns by eye is time-consuming. A typical example is climate data, where temperature or rainfall readings can vary across locations. AI can scan these readings and highlight unusual spikes or dips.
Key Benefits of Pattern Detection:
- Faster Insights: ML tools can process large volumes of data much quicker than human analysts.
- Anomaly Spotting: Outliers or unexpected events are flagged early. This can reveal interesting research leads.
- Predictive Power: Pattern detection can also feed into forecasting, improving the accuracy of models over time.
When the ML model detects a pattern, it can feed that result into visualization software. Scientists then see highlighted zones, clusters, or other cues. This reduces manual sorting and helps experts focus on valuable details.
Segmentation and Labeling with AI
Segmentation is about dividing a visual dataset into meaningful parts. In medical imaging, AI might isolate organs or highlight diseased tissue. In astronomy, it might separate galaxies, stars, and cosmic debris in large sky surveys. The same concept applies to other fields that use images or spatial data.
ML-based segmentation often relies on convolutional neural networks (CNNs). These networks excel at identifying structures in images. They apply filters that detect edges, shapes, and textures. Once trained, they can label new data quickly.
Benefits of ML-Based Segmentation:
- Consistency: AI applies the same rules every time, removing human bias.
- Speed: Large image sets can be segmented in a fraction of the time needed by human experts.
- Detail: Neural networks can detect subtle edges or boundaries that might be hard to see manually.
Next, we will explore how deep learning helps with complex tasks like image reconstruction or classification.
Deep Learning for Image Reconstruction
Deep learning techniques, especially generative models, can rebuild images from partial or noisy data. This process is called image reconstruction. It’s crucial for fields like medical imaging, remote sensing, and microscopy.
For example, MRI scans can be sped up by taking fewer measurements. A deep learning model then reconstructs the missing parts. This saves time for patients and also improves throughput in busy hospitals. Similarly, in astronomy, telescopes can gather partial data. AI fills in the gaps, producing clearer images of distant objects.
Why This Matters:
- Better image quality leads to more accurate analysis.
- Faster scanning lowers costs and wait times.
- Enhanced visuals allow experts to spot smaller details.
Reconstructed images can be fed into real-time dashboards or shared with collaborators worldwide. That level of automation frees researchers to focus on interpreting the findings.
Classification in Scientific Visualization
Another way AI aids visualization is through classification. The model learns from labeled examples, such as “this region is healthy tissue, that region is cancerous.” Then it classifies new, unseen data. This is vital in scenarios like diagnosing diseases or identifying specific chemical compounds in a sample.
Classification models can include random forests, support vector machines, or deep neural networks. The choice depends on the complexity of the data. For large, high-dimensional images, deep neural networks often perform best. Once trained, these models can rapidly classify new images, sometimes with near-human accuracy.
Practical Examples:
- Categorizing types of stars in astrophysics.
- Sorting images of microorganisms in biology.
- Labeling satellite photos of forests vs. urban zones.
Classification steps can be integrated into visualization pipelines. A dashboard may highlight each class in a different color. This lets viewers grasp patterns at a glance.
Automated Generation of Visualization Assets
AI can do more than just label existing data. It can create new assets automatically. For instance, a model can generate 3D renderings of molecular structures based on raw numeric data. Another might create time-lapse animations of an evolving weather system.
Common AI-Driven Asset Generation Approaches:
- Generative Adversarial Networks (GANs): These can create realistic images from random noise or partial inputs.
- Neural Style Transfer: This can apply styles or textures to data-based images.
- 3D Reconstruction: Combining multiple 2D images to generate a 3D model.
Automated asset generation saves significant manual effort. It also allows scientists to experiment with visual representations they might not have considered before. That leads to fresh insights and more engaging presentations.
Integrating AI with Dynamic, Real-Time Visualizations
Modern research often needs up-to-the-second data displays. For example, sensor networks in industrial plants stream data constantly. AI can learn from this flow and adjust the visualization on the fly. If a system detects a sudden change in temperature, it can highlight that zone in bright red.
In astronomy, telescopes might continuously scan the night sky. AI can spot interesting cosmic events and generate instant alerts or visual highlights. In environmental science, sensors in rivers or oceans can track pollution levels in real time. The system can then visualize these changes on interactive maps.
Key Steps to Implement Dynamic AI Visualizations:
- Set Up a Real-Time Data Pipeline: Use frameworks like Apache Kafka or MQTT.
- Deploy ML Models in Production: Host models on servers or in the cloud, ready to handle incoming data.
- Choose a Visualization Library: Tools like D3.js, Plotly, or Unity can display results interactively.
By merging AI with real-time data, scientific dashboards become more insightful. Users can react faster to changing conditions.
Common Tools and Frameworks
Developers have access to a range of frameworks to build AI-driven visualization solutions. Below are some popular options:
- TensorFlow: A widely used open-source library for building and deploying ML models.
- PyTorch: Another popular deep learning library, known for its flexible and dynamic computation graphs.
- OpenCV: Useful for image processing tasks and real-time computer vision.
- Unity or Unreal Engine: For immersive 3D visualizations and VR experiences.
- ParaView or VTK: Focused on scientific visualization with support for Python scripting and ML integration.
Choosing the right tools depends on your dataset, team expertise, and the final application. Always test your workflow with a small sample of data before scaling up.
Challenges and Best Practices
While AI offers many benefits, it also brings its own set of challenges. Below are some best practices:
- Data Quality
- Garbage in, garbage out.
- Clean and validate data before training.
- Model Overfitting
- Evaluate on separate test sets.
- Use cross-validation and regularization.
- Explainability
- Many deep learning models act like black boxes.
- Consider interpretable AI methods when trust is vital.
- Computational Costs
- Training deep models can be expensive.
- Use GPU-accelerated computing or cloud services.
- Ethical Concerns
- Biomedical or sensitive data must be protected.
- Follow privacy regulations and consent protocols.
By following these guidelines, you can reduce risks and ensure your AI project remains reliable and ethical.
Use Cases Across Different Fields
AI-driven scientific visualization helps in many areas:
- Medicine
- Automate tumor detection or organ segmentation.
- Speed up diagnostic imaging.
- Climate Science
- Predict weather patterns and track storms.
- Visualize large-scale climate models.
- Biology
- Classify cell types in microscopy images.
- Build 3D models of protein structures.
- Manufacturing
- Monitor assembly lines with real-time sensors.
- Detect flaws in products using AI-based scans.
- Astronomy
- Sort galaxies and identify new cosmic bodies.
- Automate detection of rare events.
Each field has unique data types and visualization goals. Yet the core AI techniques remain similar, involving pattern detection, classification, and asset creation.
Practical Steps to Get Started
Interested in adding AI to your scientific visualization workflow? Below are some steps to consider:
- Collect and Clean Your Data
- Ensure high-quality inputs.
- Remove duplicates or incorrect labels.
- Choose the Right Algorithms
- Start simple with proven ML methods.
- Move to deep learning if needed.
- Define Your Visualization Goals
- Do you need real-time updates?
- Are 2D or 3D views more useful?
- Train and Validate Models
- Split data into training, validation, and test sets.
- Adjust hyperparameters carefully.
- Integrate with Visualization Software
- Use frameworks that can connect to your ML outputs.
- Plan for scaling if data volume increases.
You can also form small pilot projects to test AI’s impact. Once you see success in one area, expand to larger datasets or add real-time functionality.
Future Trends in Automated Visualization
AI-based tools for scientific visualization continue to evolve. Some trends we may see:
- Improved Explainable AI: Models that visualize their own decision processes.
- Edge AI: Running ML models on devices at the data source.
- Multi-Modal Visualization: Combining images, text, and sensor data in one integrated view.
- Augmented Reality (AR) and Virtual Reality (VR): Immersive environments for data exploration.
- AutoML: Automated machine learning pipelines that handle data preparation and model selection.
These developments will make scientific visualization more accessible. They will also help scientists draw new connections between data sources and real-world outcomes.
Key Takeaways
Machine Learning and AI are reshaping how scientists explore and present data. Here are the main insights:
- Automated Visualization: Faster, more accurate data insights with less manual effort.
- Segmentation & Classification: Highlight important details and label them automatically.
- Real-Time Updates: Respond to changes instantly for better monitoring and control.
- Deep Learning: High performance in tasks like image reconstruction and object detection.
- Future Growth: Expect new tools that offer even more user-friendly and powerful features.
Ultimately, AI opens the door to deeper understanding of complex datasets. With the right approach and tools, scientists can focus on discovery rather than tedious data wrangling.
Conclusion
Machine Learning and AI offer a powerful boost to scientific visualization. They enable pattern detection, segmentation, image reconstruction, and automated asset creation. These technologies also support real-time dashboards and dynamic visualization. By adopting AI, researchers can streamline their workflows and gain faster insights.
To succeed, start with clean data, pick suitable algorithms, and align the visual outputs with your research goals. Stay aware of challenges like model explainability and computational costs. With consistent practice and well-chosen tools, your projects can benefit from the latest AI methods in automated scientific visualization.
Embrace these techniques now to gain a competitive edge in science, industry, or any field that relies on data-driven insights.
Author Profile
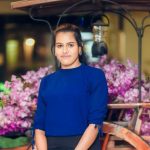
- Online Media & PR Strategist
- Hello there! I'm Online Media & PR Strategist at NeticSpace | Passionate Journalist, Blogger, and SEO Specialist
Latest entries
Data AnalyticsJune 13, 2025Future of Data Warehousing in Big Data
AI InterfaceJune 13, 2025Aligning AI Developments with Corporate Goals in the AI Era
HPC and AIJune 13, 2025HPC Architecture Taking to the Next Level
Quantum ComputingJune 13, 2025Ethical Issues in Quantum Tech: Privacy, Jobs, and Policy