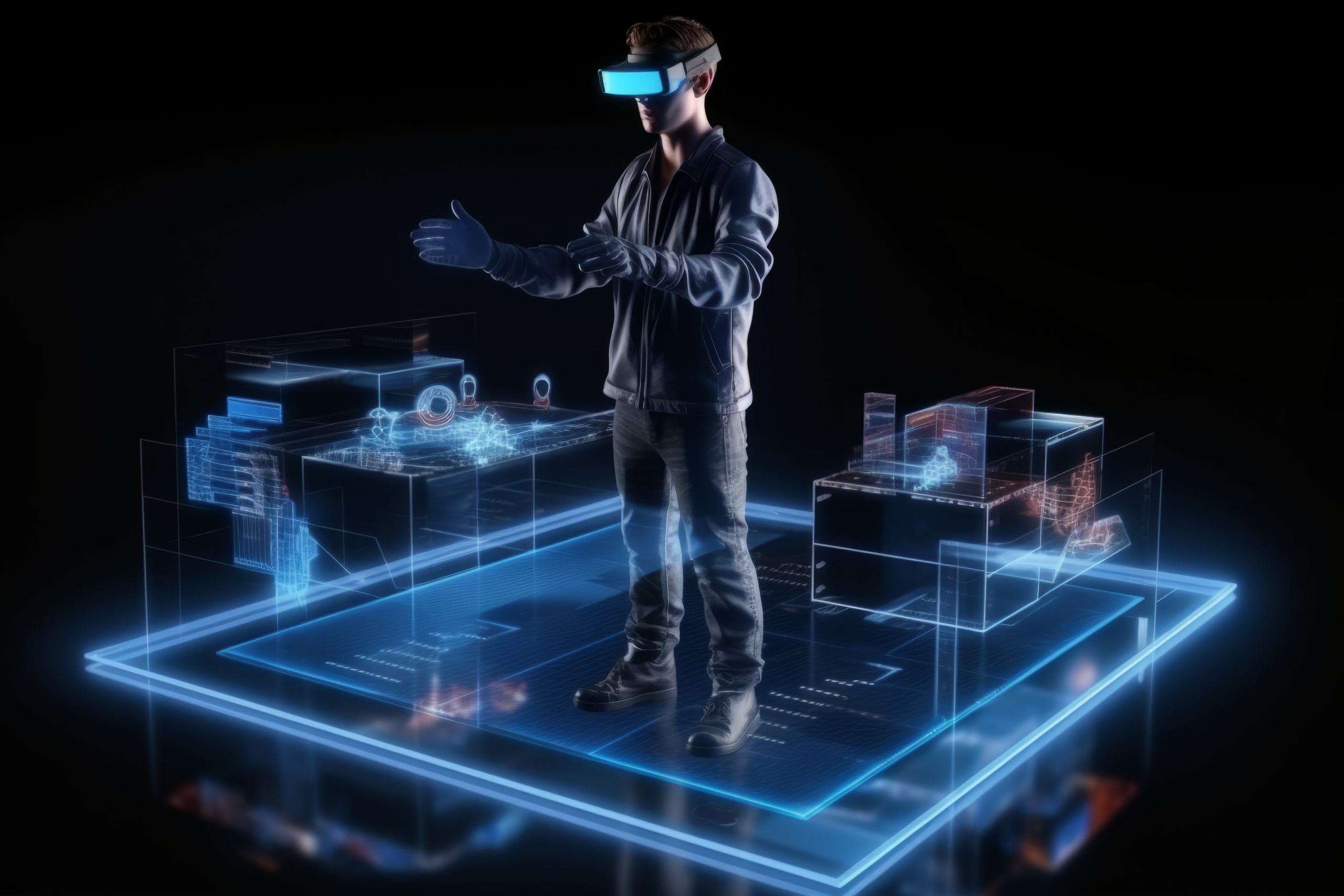
How Companies Move from Basic Mirrored Data to Full-Scale Predictive Analytics
Digital twin technology is changing how businesses manage operations. It offers new ways to analyze performance and forecast results. If you want to optimize cleaning processes, reduce costs, and improve safety, then this guide is for you. By the end, you’ll understand how to move from basic mirrored data to advanced predictive analytics.
What You’ll Learn
- The meaning of “digital twin maturity.”
- The difference between simple mirrored data and predictive analytics.
- Steps to implement predictive digital twins for cleaning and facility operations.
Understanding Basic Mirrored Data
Basic mirrored data is the first level of digital twin maturity. At this stage, the digital twin is a real-time representation of physical conditions. For a cleaning site, this could mean tracking foot traffic or cleaning supply levels. The goal is to collect accurate data that reflects current conditions in your building.
However, mirrored data alone has limits. It only tells you what is happening right now. You do not get insights into what might happen next. You simply see a mirror image of reality, which is still valuable but not predictive.
In many cleaning operations, basic mirrored data comes from sensors. These might track temperature, humidity, or how often certain areas get used. Even with this information, you must still make manual decisions. Without predictive analytics, you can’t forecast and optimize future cleaning schedules.
Why Digital Twin Maturity Matters
Maturity in digital twin technology means going beyond simple monitoring. As you advance, your digital twin can suggest actions based on patterns in the data. This includes predicting when a floor will need more frequent cleaning. It can also help you optimize resource use and staffing.
Gaining maturity isn’t just about technology. It’s about shifting your operations to rely on data-driven decisions. When you align your cleaning strategies with what your digital twin shows, you become more efficient. This leads to better quality control, lower costs, and improved worker safety.
The 4 Steps Toward Full-Scale Predictive Analytics
Below is a clear roadmap to help you progress from basic mirrored data to robust predictive analytics. Each step builds on the previous one.
1. Data Collection
First, you need reliable data. Sensors capture real-world conditions such as:
- Room occupancy
- Foot traffic
- Air quality
- Cleaning fluid levels
Make sure the data is clean and accurate. Incomplete or incorrect data can create wrong assumptions. If you are tracking cleaning supply levels, validate that your sensor readings match physical inventories. Strong data quality at this stage sets the foundation for all next steps.
2. Real-Time Monitoring
Next, set up live dashboards or alerts. This is where the mirrored data becomes visible to your team. For a cleaning site, you might see which areas have the highest traffic at any given moment. You can also track how often trash bins fill up. By monitoring in real time, you can make immediate decisions. This is still reactive, but it’s a leap from relying on manual checks.
3. Predictive Analysis
Then, layer machine learning or advanced analytics on top of your real-time data. This step is the core of digital twin maturity. Predictive models can:
- Estimate when a specific area will need extra cleaning
- Forecast supply shortages
- Identify unusual patterns that might indicate a spike in facility usage
A predictive system doesn’t just tell you “what is.” It tells you “what could be.” This lets you plan proactively. For example, if your digital twin shows that a certain restroom will reach maximum traffic at 2 PM, you can schedule staff to clean it right after the rush. That saves time, reduces complaints, and ensures better hygiene.
4. Prescriptive Decisions
Finally, move to full-scale predictive analytics with prescriptive capabilities. This is where your system suggests the best actions and can even automate certain tasks. For instance:
- Auto-scheduling: The system can create cleaning schedules based on predicted usage.
- Smart replenishment: Orders for cleaning supplies can trigger automatically when stock runs low.
- Resource optimization: Staff timesheets are synced with high-traffic areas, saving labor costs.
This level of maturity is the ultimate goal. When your digital twin can learn from data and recommend the best course of action, your cleaning operations become more efficient.
Key Tools and Technologies
Certain tools help you progress through these stages more quickly. Here are some examples:
- IoT Sensors
- Monitor real-time conditions like temperature, moisture, or foot traffic.
- Low-power sensors can send data wirelessly for remote areas.
- Cloud Platforms
- Store and process large sets of data at scale.
- Offer features like data lakes, analytics services, and machine learning tools.
- Edge Computing
- Process data close to where it is generated.
- Helps reduce latency and save bandwidth.
- Machine Learning Frameworks
- Tools like TensorFlow or PyTorch can build predictive models.
- Simple drag-and-drop platforms also exist for non-technical users.
- Data Visualization Dashboards
- Show real-time metrics and alerts.
- Make it easy to spot trends and outliers.
You don’t have to adopt every tool at once. Focus on the technologies that fit your budget and goals.
Overcoming Common Challenges
Implementing a digital twin can be exciting but also challenging. Below are some common issues and tips on how to solve them:
- Data Overload
- Issue: Collecting too much data without a plan leads to confusion.
- Solution: Start small. Identify critical metrics that matter most to your cleaning operations.
- High Costs
- Issue: Sensors, cloud services, and skilled staff can be expensive.
- Solution: Try pilot projects to prove value. Scale your solutions gradually.
- Security Concerns
- Issue: Data breaches can expose sensitive information.
- Solution: Use strong encryption, robust access controls, and compliance with data privacy laws.
- Team Resistance
- Issue: Employees may worry about job changes or new technology.
- Solution: Offer training, explain how digital twins enhance their roles, and show them the benefits.
- Lack of Clear ROI
- Issue: Decision-makers need proof of return on investment.
- Solution: Measure key performance indicators (KPIs). Show data-driven wins such as reduced cleaning times or fewer supply shortages.
Connecting Digital Twins with Cleaning Site Success
A digital twin for a cleaning site goes beyond simple task scheduling. It can track supply inventories and staff productivity. It can predict how many visitors will enter a building on a specific day. This knowledge helps you schedule staff when and where they are needed most.
When combined with predictive analytics, you can also spot trends in maintenance tasks. For example, if certain floors require more frequent attention, you can adjust cleaning routines proactively. This level of maturity reduces waste, cuts costs, and improves the overall environment for customers and employees.
Future Outlook and Final Thoughts
Digital twin maturity has a clear roadmap: start with mirrored data, scale up to predictive analytics, and then adopt prescriptive actions. Each step can bring immediate benefits to your cleaning operations. You will see cost savings, better labor allocation, and improved customer satisfaction.
If you are new to digital twins, begin with small projects. As you collect real-time data and apply simple analytics, you’ll build momentum. Over time, your cleaning site can adopt advanced tools and machine learning. The future holds endless ways to optimize with digital twins. The only limit is how far you’re willing to take your technology.
Are you ready to move beyond basic mirrored data? Then it’s time to invest in predictive analytics for your cleaning site. You’ll not only keep your facility spotless but also stay ahead of the curve.
Author Profile
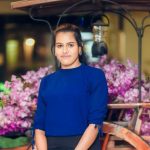
- Online Media & PR Strategist
- Hello there! I'm Online Media & PR Strategist at NeticSpace | Passionate Journalist, Blogger, and SEO Specialist
Latest entries
Simulation and ModelingApril 30, 2025Chaos Engineering: Build Resilient Systems with Chaos Monkey
Digital Twin DevelopmentApril 30, 2025How to Ensure Data Synchronization Twins Effectively
Scientific VisualizationApril 30, 2025Deepfake Scientific Data: AI-Generated Fraud in Research
Data AnalyticsApril 30, 2025What Is Data Mesh Architecture and Why It’s Trending