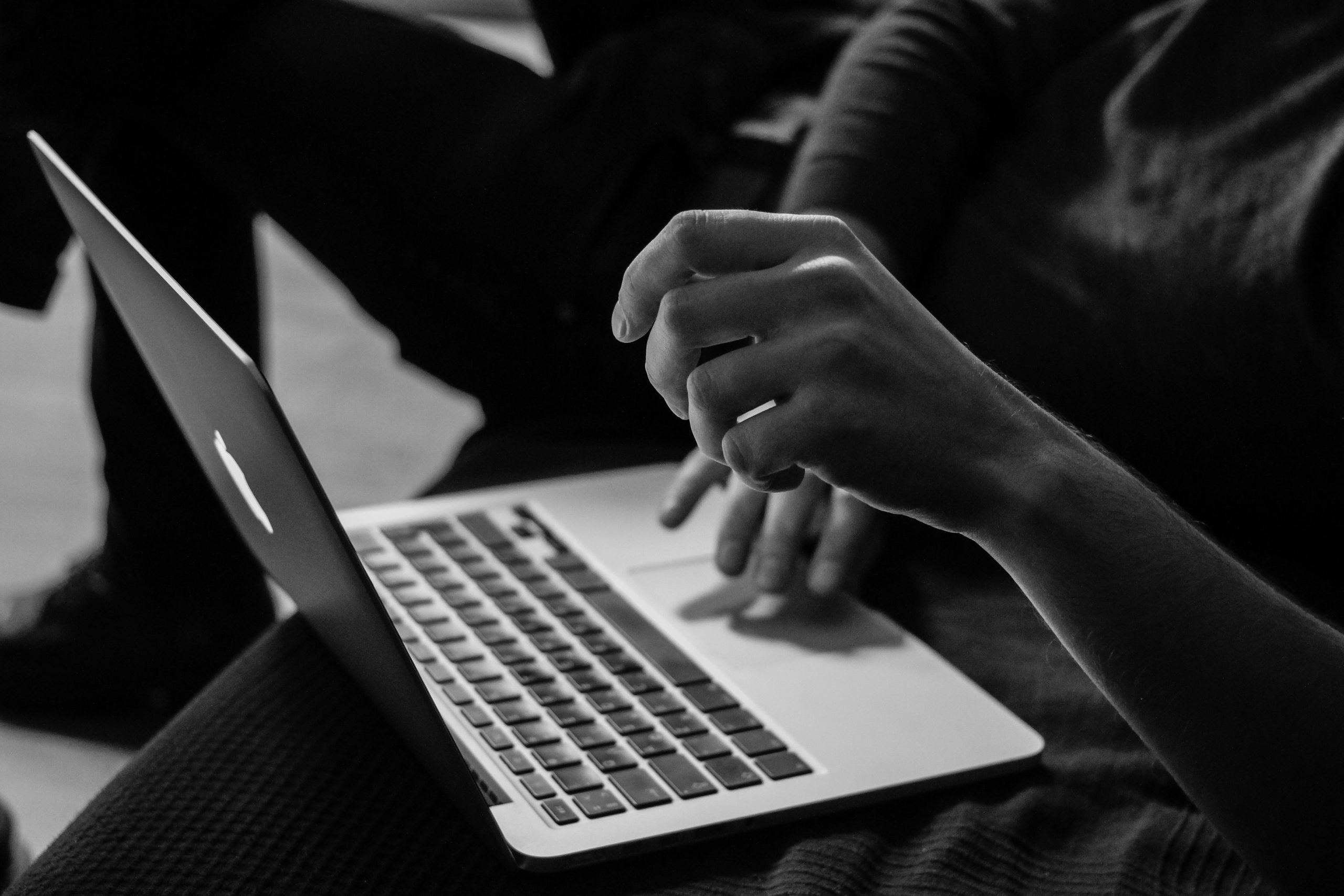
GPU Acceleration Transforms Rendering: Quantum Computing Impact
Introduction to GPU Acceleration in Rendering Workflows
GPU acceleration uses specialized graphics processing units to handle heavy computing tasks. Traditional CPUs are general-purpose, while GPUs excel at parallel operations. This distinction makes GPUs perfect for rendering, which relies on repetitive calculations.
First, a GPU can process thousands of threads at once. That means it can render and iterate through complex pixel data faster than a CPU. Next, modern GPUs are optimized with dedicated cores for lighting, shading, and physics calculations. Finally, software developers have integrated GPU support into major 3D tools, making GPU acceleration more accessible.
Key Benefits of GPU Acceleration for Rendering
GPU acceleration offers clear advantages for artists, engineers, and studios. Let’s examine the main benefits that show how GPU acceleration is transforming rendering workflows.
Faster Rendering Times
Render tasks demand large amounts of computation. GPUs divide these tasks into parallel operations. This parallelization slashes rendering times significantly compared to CPU-only solutions.
- Real-Time Feedback: Artists can see changes almost instantly.
- Shortened Deadlines: Animation teams can complete projects faster.
- Iterative Creativity: More time to experiment with lighting and textures.
More Complex Visualizations
Detail-rich projects typically require more processing power. GPU acceleration supports higher polygon counts, deeper textures, and advanced visual effects. This capability gives creators the freedom to build photorealistic models and intricate environments.
- Higher Polygon Budgets: Scenes can include more objects with complex geometry.
- Advanced Shading: Ray tracing and global illumination run smoother.
- Dynamic Effects: Simulations like fog and water are rendered with more detail.
Cost and Resource Efficiency
Using a GPU for rendering can save you money in the long run. You can accomplish more with fewer machines. Each rendering node can handle more data, reducing the need for extra hardware.
- Reduced Infrastructure: Fewer render nodes mean lower energy costs.
- Scalable Solutions: Add more GPUs for extra power.
- Long-Term Savings: Quicker render times lead to lower operational expenses.
Accelerating Workflows with GPUs: Best Practices
Optimizing your workflow is just as important as hardware upgrades. Here are steps to get the most out of GPU acceleration for rendering workflows:
-
Update Your Software
- Ensure you have the latest GPU drivers.
- Use rendering software that supports GPU acceleration.
-
Choose the Right GPU
- Look for GPUs designed for 3D rendering.
- Check memory capacity and core count.
-
Leverage Hybrid Rendering
- Some pipelines use both CPU and GPU.
- This hybrid approach can maximize resource usage.
-
Optimize Scenes
- Reduce unnecessary geometry.
- Use efficient materials and shaders.
-
Benchmark Regularly
- Test render times under different settings.
- Identify the best balance of speed and quality.
Following these best practices ensures that GPU acceleration truly transforms your rendering workflows.
Examining Quantum Computing in AI and Machine Learning
Faster rendering might be just the start. Quantum computing has the potential to accelerate AI and machine learning algorithms in ways we have never seen. This technology deals with quantum bits (qubits) instead of standard binary bits, promising massive computational leaps.
What Is Quantum Computing?
Quantum computing takes advantage of quantum states like superposition and entanglement. These states let qubits represent multiple possibilities at once. This means a quantum computer can evaluate many outcomes in parallel.
- Superposition: A qubit can be 0, 1, or both at the same time.
- Entanglement: Linked qubits affect each other’s states instantly.
- Quantum Speedup: Potential to solve complex problems much faster.
Why Quantum Computing Matters for AI
AI models involve enormous datasets. Traditional computers can struggle with the sheer volume of calculations. Quantum computing could open new frontiers in data processing and model training.
First, quantum algorithms may handle optimization tasks faster. Next, large-scale machine learning may benefit from quantum-enhanced pattern recognition. Finally, as the technology matures, we may see breakthroughs in AI model complexity that were impossible before.
Bringing It All Together
GPU acceleration has already proven its worth for rendering workflows. It offers faster processing, more visual detail, and lower long-term costs. Meanwhile, quantum computing stands ready to push computational boundaries even further. As both technologies evolve, studios and research teams will discover new ways to combine their strengths.
Imagine a future where GPUs handle most 3D rendering tasks, and quantum computers tackle the toughest AI training. This combined approach might redefine what is possible, from hyper-realistic virtual worlds to advanced machine learning models that handle massive data instantly.
Conclusion
Rendering has come a long way from the days of waiting days for final outputs. GPU acceleration has transformed the industry by cutting render times and enabling complex, visually rich projects. Looking ahead, quantum computing may speed up AI and machine learning, creating an even faster world of innovation.
By using GPUs wisely and keeping an eye on quantum technology, you can stay ahead in a rapidly changing field. Embrace these advancements to produce quality work, meet tight deadlines, and keep costs under control. That is how GPU acceleration is transforming rendering workflows and preparing us for the next computing revolution.
FAQ
1. What types of projects benefit most from GPU acceleration?
Projects with complex visuals, such as films, gaming, and architectural visualization, benefit the most. Any task that involves heavy 3D rendering or simulation can see large performance gains.
2. Do all rendering engines support GPU acceleration?
Not all engines do, but many modern ones have added GPU support. Popular tools like Blender, Arnold (GPU version), and Redshift are optimized for GPUs.
3. Can I use multiple GPUs to speed up rendering?
Yes. Many systems allow multiple GPUs to divide the workload. This setup can greatly reduce render times for complex scenes.
4. How does quantum computing differ from GPU acceleration?
GPU acceleration relies on parallel processing of standard binary operations. Quantum computing uses qubits, which can represent multiple states simultaneously. The two technologies solve different types of problems but can be complementary.
5. When will quantum computing be widely available for AI?
Researchers are making progress, but quantum computing is still in its early stages. We may see more practical applications for AI within the next decade as hardware and algorithms improve.
Author Profile
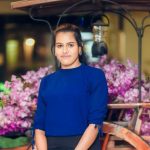
- Online Media & PR Strategist
- Hello there! I'm Online Media & PR Strategist at NeticSpace | Passionate Journalist, Blogger, and SEO Specialist
Latest entries
Digital Twin DevelopmentApril 30, 2025How to Ensure Data Synchronization Twins Effectively
Scientific VisualizationApril 30, 2025Deepfake Scientific Data: AI-Generated Fraud in Research
Data AnalyticsApril 30, 2025What Is Data Mesh Architecture and Why It’s Trending
Rendering and VisualizationApril 30, 2025Metaverse Rendering Challenges and Opportunities