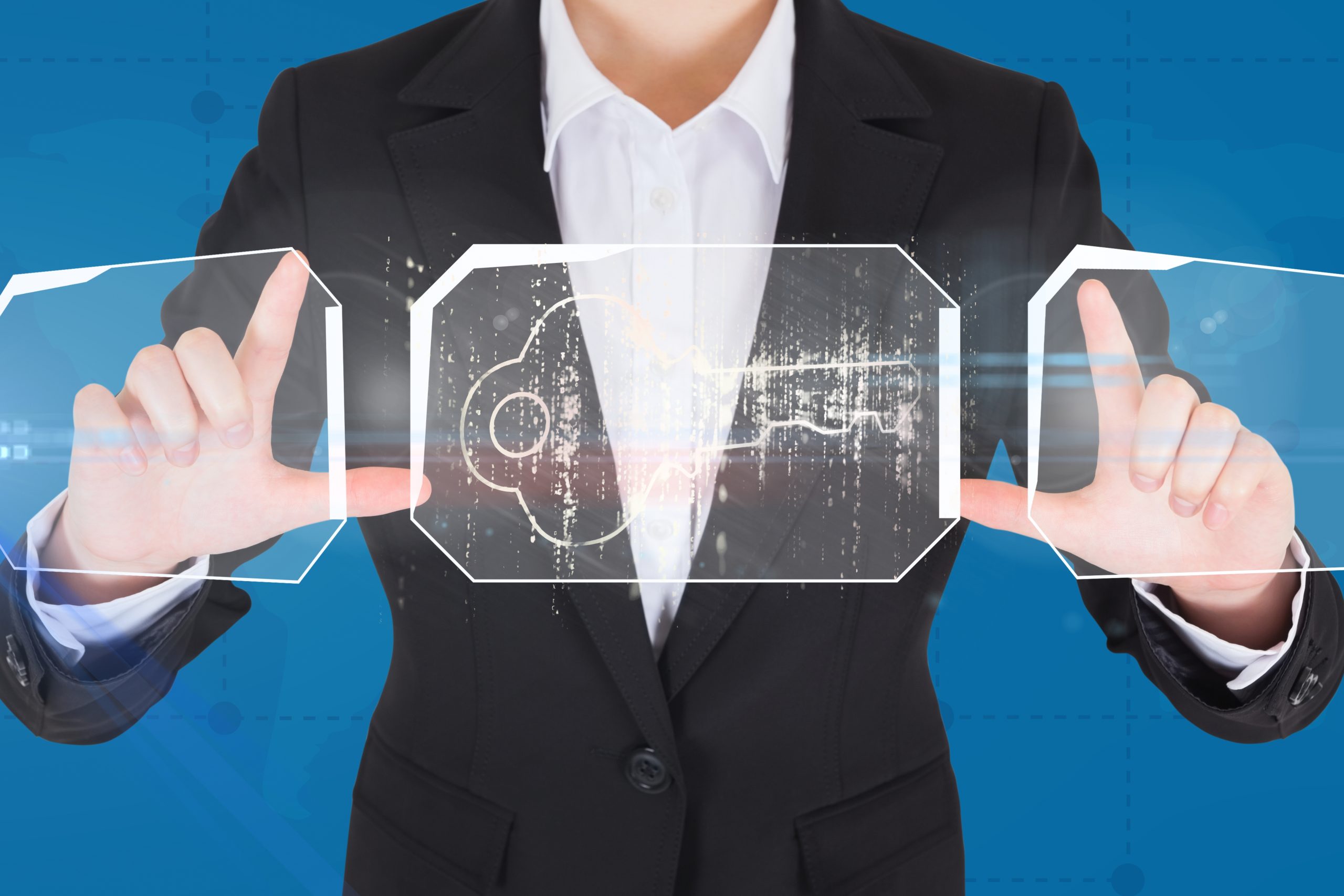
Ethics and Responsible AI in MLOps
Artificial Intelligence (AI) is transforming industries, but with great power comes great responsibility. As machine learning (ML) models become more integrated into decision-making processes, ensuring ethical practices in MLOps (Machine Learning Operations) is critical. This blog explores how to address bias, ensure fairness, and comply with regulations while deploying AI solutions responsibly.
By the end of this article, you’ll understand:
- The importance of fairness, privacy, and bias mitigation in ML models.
- Techniques like LIME and SHAP for explainability.
- How regulatory frameworks like GDPR and CCPA impact AI deployment.
- Best practices in MLOps to promote responsible AI.
Why Ethics and Responsible AI Matter in MLOps
AI systems are only as good as the data they’re trained on. If the data contains biases, the model will reflect those biases, leading to unfair outcomes. For example, biased hiring algorithms can discriminate against certain groups. Responsible AI in MLOps ensures that models are fair, transparent, and compliant with legal standards.
Ethical AI isn’t just about avoiding harm—it’s about building trust. Users need to understand how decisions are made, especially in high-stakes areas like healthcare or finance. This is where MLOps plays a key role in embedding ethical practices into the AI lifecycle.
Understanding Bias, Fairness, and Privacy in ML Models
What is Bias in Machine Learning?
Bias occurs when a model produces skewed results due to flawed assumptions or unrepresentative data. For example, a facial recognition system trained mostly on one ethnicity may perform poorly on others.
Ensuring Fairness
Fairness means ensuring that ML models treat all groups equally. Techniques like re-sampling data, adjusting model weights, or using fairness-aware algorithms can help reduce bias.
Protecting Privacy
Privacy is another critical concern. ML models often rely on sensitive data, such as medical records or financial information. Techniques like differential privacy and data anonymization can help protect user data while maintaining model accuracy.
Techniques for Explainability in AI Models
Explainability is the ability to understand and interpret how a model makes decisions. This is crucial for building trust and ensuring compliance with regulations.
LIME (Local Interpretable Model-agnostic Explanations)
LIME explains individual predictions by approximating the model locally. It helps users understand why a specific decision was made, even in complex models.
SHAP (SHapley Additive exPlanations)
SHAP assigns each feature an importance value for a particular prediction. This provides a clear picture of how different factors influence the model’s output.
Other Techniques
Feature Importance – dentifies which features contribute most to predictions.
Decision Trees -Visualizes decision paths in tree-based models.
Explainability not only builds trust but also helps identify and correct biases in the model.
Regulatory Frameworks and Compliance in AI Solutions
AI deployment must comply with legal standards to protect user rights and avoid penalties. Two key regulations are GDPR and CCPA.
GDPR (General Data Protection Regulation)
GDPR is a European law that mandates transparency, data protection, and user consent. AI systems must provide clear explanations for automated decisions and allow users to opt out.
CCPA (California Consumer Privacy Act)
CCPA gives California residents the right to know how their data is used and to request its deletion. AI solutions must ensure data privacy and provide opt-out mechanisms.
Compliance Best Practices
- Conduct regular audits to ensure data privacy and fairness.
- Implement robust data governance frameworks.
- Provide clear documentation and user-friendly explanations for AI decisions.
How MLOps Practices Promote Responsible AI
MLOps integrates ethical considerations into every stage of the ML lifecycle, from data collection to deployment.
Reducing Bias in MLOps
Data Audits – Regularly check datasets for biases and imbalances.
Diverse Teams – Involve diverse teams in model development to identify potential biases.
Continuous Monitoring – Track model performance across different groups to ensure fairness.
Encouraging Transparency
Model Documentation – Maintain detailed records of model development and decision-making processes.
User Communication – Provide clear, accessible explanations for AI-driven decisions.
Ensuring Compliance
Automated Compliance Checks – Use tools to monitor compliance with regulations like GDPR and CCPA.
Ethical Guidelines – Establish internal guidelines for responsible AI development.
FAQs on Ethics and Responsible AI in MLOps
1. What is the role of MLOps in ethical AI?
MLOps ensures that ethical practices, such as fairness, transparency, and compliance, are embedded throughout the ML lifecycle.
2. How can I detect bias in my ML model?
Use techniques like fairness metrics, data audits, and continuous monitoring to identify and mitigate bias.
3. What are LIME and SHAP?
LIME and SHAP are explainability techniques that help users understand how ML models make decisions.
4. How does GDPR affect AI deployment?
GDPR requires AI systems to be transparent, protect user data, and provide explanations for automated decisions.
5. Can MLOps help with regulatory compliance?
Yes, MLOps practices like automated compliance checks and robust data governance ensure adherence to regulations like GDPR and CCPA.
Building a Future of Responsible AI
Ethics and responsible AI in MLOps are not just buzzwords—they’re essential for building trustworthy, fair, and compliant AI systems. By addressing bias, ensuring explainability, and adhering to regulatory frameworks, organizations can deploy AI solutions that benefit everyone.
Author Profile
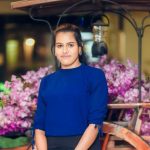
- Online Media & PR Strategist
- Hello there! I'm Online Media & PR Strategist at NeticSpace | Passionate Journalist, Blogger, and SEO Specialist
Latest entries
Conversational AIJune 20, 2025Conversational AI Security: Risks & Mitigation Strategies
Data AnalyticsJune 13, 2025Future of Data Warehousing in Big Data
AI InterfaceJune 13, 2025Aligning AI Developments with Corporate Goals in the AI Era
HPC and AIJune 13, 2025HPC Architecture Taking to the Next Level