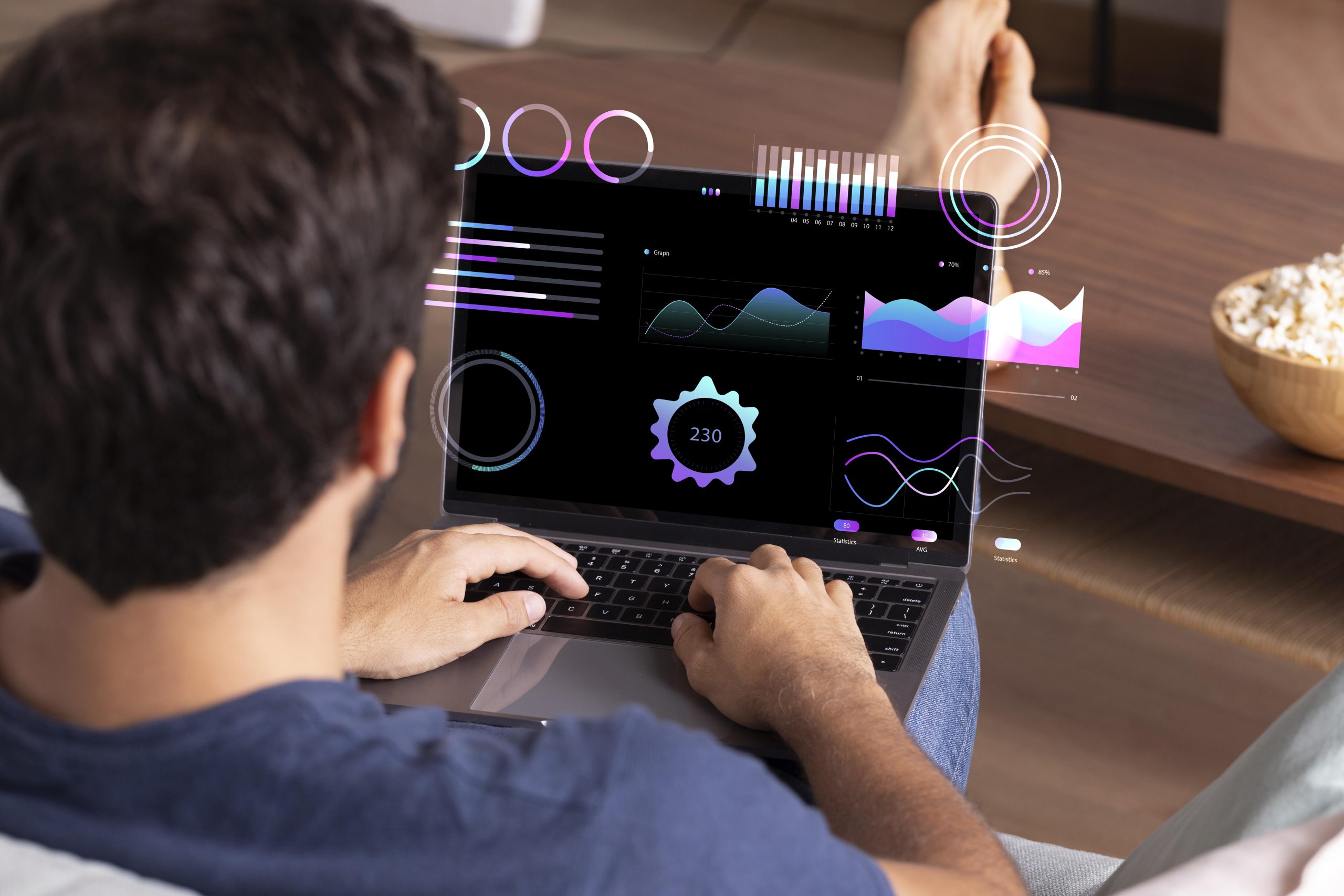
Cost Optimization Strategies for MLOps
Machine learning operations (MLOps) can be expensive. Many teams face escalating costs. This blog post shows how to cut these expenses without hurting performance. You’ll learn about compute optimization, data storage tips, and ways to measure ROI and TCO. Let’s get started.
Why MLOps Cost Optimization Matters
MLOps covers every stage of building and deploying machine learning solutions. This includes data collection, model development, and continuous monitoring. Each of these steps can drain your budget if not managed well.
First, large datasets can drive up storage costs. Next, computationally heavy training jobs can increase cloud bills. Finally, scaling your ML models in production can cause further expenses. Cost optimization reduces these problems while keeping model performance intact.
Budget-friendly MLOps helps you invest more in innovation. You can spend savings on research, talent, or advanced tools. It also makes your organization more competitive. A well-designed MLOps plan focuses on long-term gains and sustainability.
Optimize Compute Resources
Machine learning workloads can be compute-heavy. Training large models often requires powerful hardware. If you over-allocate resources, you pay for unused capacity. If you under-allocate, performance suffers. Striking the right balance is key.
Auto-Scaling
Auto-scaling helps you match compute resources to current workloads. With auto-scaling, your system adds or removes server instances automatically. This ensures efficient resource use during peak and off-peak periods.
- Advantages:
- Cuts costs during low-demand periods.
- Improves model performance by adding servers when demand spikes.
- Reduces manual intervention.
- Disadvantages:
- Improper configurations can lead to sudden cost spikes.
- Delays in scaling up might slow real-time inference for a short time.
Tip: Use metrics like CPU usage, RAM usage, and request counts. These indicators help you decide when to scale up or down.
Consider Spot Instances
Spot instances let you rent unused cloud compute capacity at discounted rates. Cloud providers like AWS and Azure offer these as “spot” or “preemptible” instances. They can cost up to 90% less than standard on-demand instances. However, they can be reclaimed by the provider at short notice.
Spot instances are best for tasks that can handle interruptions. For example, large batch training jobs that can resume from checkpoints. If your workflow can pause mid-way without losing progress, spot instances can provide big savings.
- Implementation Tips:
- Set up frequent checkpoints during training.
- Use container orchestration (Kubernetes) for quick restarts.
- Keep a backup plan for sudden instance termination.
- Trade-offs:
- Risk of interruptions.
- Extra complexity in checkpointing and job management.
Embrace Serverless ML
Serverless computing abstracts away server management tasks. Functions run in response to events. You pay only for the time your code executes. This can be a good match for ML tasks that don’t run continuously.
Batch Inference With Serverless
Many ML tasks involve periodic processing. Examples include generating weekly recommendations or monthly reports. Serverless systems can spin up quickly for these batch tasks. Once they finish, costs return to zero.
- Pros:
- No ongoing charge when idle.
- Minimal operations overhead.
- Automatic scaling based on event triggers.
- Cons:
- Not ideal for large, long-running training jobs.
- Possible cold start delays for real-time workloads.
Event-Driven Microservices
You can build event-driven ML microservices using serverless functions. For instance, an image recognition function might run each time a new image arrives in a cloud storage bucket. This approach can lower costs by preventing the need for a constantly running server.
Right-Sizing Instances
Choosing the correct instance type can save you money. Different workload types need different CPU, GPU, and memory ratios. Running on a massive instance for a small ML task wastes money. A tiny instance will slow big training jobs.
CPU vs. GPU
- CPU Instances:
- Good for smaller models and low-latency inference.
- Cheaper than GPU instances for many use cases.
- GPU Instances:
- Faster for complex tasks like deep learning.
- More expensive on a per-hour basis.
Evaluating Instance Options
- Benchmark Model Training:
- Run a short benchmark on a few instance types.
- Compare cost-per-epoch or cost-per-iteration.
- Pick the best cost-performance balance.
- Monitor Resource Usage:
- Track CPU, GPU, and memory during training.
- Use tools like nvidia-smi for GPU metrics.
- Adjust instance sizes based on real data.
Efficient Data Storage and Retrieval
Data storage may account for a big part of your ML budget. Larger datasets cost more to store. Frequent retrieval adds to expenses. Optimizing data storage can free up funds for other MLOps tasks.
Tiered Storage
Cloud providers offer tiered storage solutions. For example, AWS has S3 Standard, S3 Infrequent Access, and Glacier. You pay more for quick retrieval, but less for rarely accessed data. Placing the right data in the right tier can cut costs.
- Best Practices:
- Keep frequently accessed training data in a hot tier.
- Archive older or unused data to a cold tier.
- Set lifecycle policies to move data after a certain time.
Data Compression and Deduplication
ML datasets can have redundant records or repeated files. Compressing or deduplicating data can reduce storage costs.
- Compression:
- Tools like gzip or Snappy can cut file sizes.
- Use a balanced compression level to avoid slow reads.
- Deduplication:
- Remove duplicate files or images from your dataset.
- Use hashing methods to detect duplicates.
Caching Strategies
Caching improves data retrieval times. It also lowers repeated storage reads. Tools like Redis or Memcached can store recently used data. This works well if your training or inference system reuses data blocks often.
Tools and Techniques to Measure ROI and TCO
Reducing costs starts with knowing where money goes. You need to measure return on investment (ROI) and total cost of ownership (TCO). This data will guide your decisions.
Cost Monitoring Dashboards
Cloud providers offer cost monitoring dashboards. Examples include AWS Cost Explorer and Azure Cost Management. These tools show real-time cost breakdowns. You can also set budgets and alerts for overspending.
- Tips:
- Check these dashboards weekly or daily.
- Identify cost spikes quickly.
- Tag resources by project or department to track spending sources.
Third-Party Monitoring Tools
Some third-party tools provide advanced analytics for cloud usage. Examples include CloudHealth, Datadog, or New Relic. They can help you track CPU usage, memory usage, network traffic, and more.
- Pros:
- Deeper insights and custom reports.
- Multi-cloud support.
- Alerts and recommendations.
- Cons:
- Extra subscription costs.
- Learning curve for advanced features.
Cost-Benefit Analysis
A cost-benefit analysis compares the expected gains of an ML project to the expenses. This might include:
- Projected revenue increase from better predictions.
- Cost savings from automation.
- Development and maintenance costs of your ML pipeline.
If the financial gains outweigh the costs, your project is in good shape. If not, you may need to refine your strategies.
Real-World Tips for Cutting Costs Without Sacrificing Performance
Theory is one thing, but practice is what counts. Here are proven methods teams use to reduce MLOps expenses while keeping results strong.
Adopt a Model Registry
A model registry helps you track different model versions. This is vital when you have many models in play. Without a registry, you might retrain models unnecessarily. A registry ensures you reuse trained models when possible.
- Suggested Tools:
- MLflow’s model registry.
- Neptune.ai’s model tracking.
- Comet’s versioning system.
Use Pretrained Models and Transfer Learning
Some tasks don’t need training from scratch. Transfer learning allows you to start from a model that already learned useful features. This cuts compute time significantly.
- Example:
- Use a pretrained BERT model for NLP tasks.
- Fine-tune it on your specific dataset.
Intelligent Model Scheduling
Scheduling training jobs during off-peak hours can lower costs. Cloud providers may have lower spot instance prices at night. You could also stagger training jobs to avoid resource conflict.
- Strategies:
- Run less urgent jobs on weekends.
- Consolidate tasks to reduce overhead.
Containerize Your Workloads
Containers standardize your environment. This avoids version conflicts and improves resource usage. Kubernetes can pack containers tightly on nodes, ensuring minimal waste.
- Advice:
- Monitor cluster resource usage.
- Scale clusters based on real demand.
- Use reserved capacity if you expect long-term consistent usage.
Implement Proper Monitoring
Monitoring helps you detect issues before they become costly. A sudden increase in inference latency might signal a scaling problem. Unusual usage patterns might suggest a data drift that leads to wasted retraining.
- Key Metrics to Track:
- Model performance (accuracy, F1-score).
- System metrics (CPU, GPU, memory, disk I/O).
- Financial metrics (cost per hour, cost per user).
Collaborate with Finance Teams
Finance teams can offer insights into budgeting and cost planning. They can help you forecast spending patterns. Working together ensures a balanced approach to MLOps cost management.
Putting It All Together
MLOps cost optimization isn’t about going cheap. It’s about smart allocation of your resources. By right-sizing compute, adopting serverless where possible, and storing data efficiently, you can save money without losing performance. Tracking ROI and TCO gives clarity on where each dollar goes.
When you build your MLOps pipeline with these strategies, you create a sustainable environment for machine learning projects. You maintain agility and keep stakeholders happy. Best of all, you direct more funds toward innovation and value-driven activities.
Conclusion
Cost optimization in MLOps matters to any organization running machine learning at scale. By auto-scaling compute resources, using spot instances, and leveraging serverless ML, you can reduce your spending. Efficient data storage also plays a big part, so tier your data, compress large files, and use caching for frequently accessed datasets.
Next, keep an eye on your ROI and TCO with built-in cloud dashboards or third-party monitoring tools. Real-world tips like adopting a model registry and using transfer learning can further trim costs. Finally, collaborate across teams to manage finances in a structured way. Through these steps, you’ll master MLOps cost optimization and drive better outcomes without sacrificing performance.
Author Profile
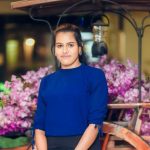
- Online Media & PR Strategist
- Hello there! I'm Online Media & PR Strategist at NeticSpace | Passionate Journalist, Blogger, and SEO Specialist
Latest entries
Simulation and ModelingApril 30, 2025Chaos Engineering: Build Resilient Systems with Chaos Monkey
Digital Twin DevelopmentApril 30, 2025How to Ensure Data Synchronization Twins Effectively
Scientific VisualizationApril 30, 2025Deepfake Scientific Data: AI-Generated Fraud in Research
Data AnalyticsApril 30, 2025What Is Data Mesh Architecture and Why It’s Trending