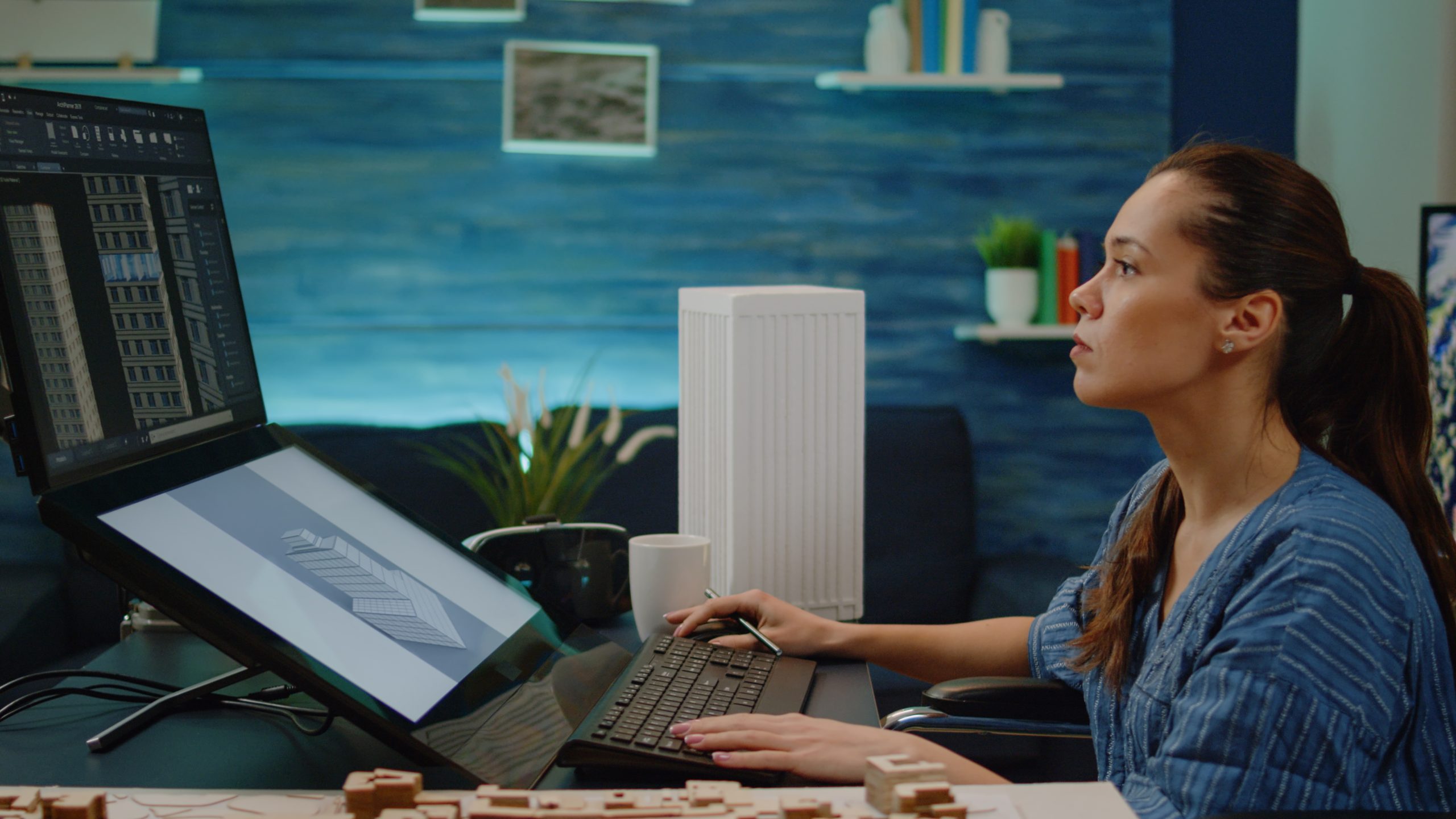
Climate Modeling on Supercomputers
Climate change affects every corner of our planet. Yet, it’s hard to predict exactly how weather, oceans, ice, and land will behave in the future. That’s where climate modeling on supercomputers comes in. Today, you will learn how these powerful tools help scientists create better climate projections and face big data challenges.
What Are Climate Models?
Climate models are computer programs that simulate Earth’s weather and environmental processes. They combine data from the atmosphere, oceans, ice sheets, and land surfaces. Each part interacts with the others and shapes how our climate behaves. Without these models, we would struggle to predict future climate patterns or plan for changes ahead.
Key Components of Climate Models
- Atmospheric processes: Air movement, cloud formation, and gas emissions.
- Ocean dynamics: Currents, temperature shifts, and salinity levels.
- Ice coverage: Glaciers, ice sheets, and sea ice.
- Land interactions: Soil moisture, vegetation, and snow cover.
Each part needs careful study. Combining them provides a complete view of Earth’s climate system.
Why Use Supercomputers?
Climate models require huge amounts of calculation. A single simulation can involve billions of data points. This level of detail is too big for regular computers. Supercomputers, also known as High-Performance Computing (HPC) systems, have the processing power and memory to handle these tasks.
Benefits of HPC in Climate Science
- Faster results: Complex climate models can run in days instead of months.
- Higher resolution: HPC systems let scientists study smaller regions in greater detail.
- More accurate forecasts: By handling more data, HPC improves climate projections.
- Global collaboration: Large-scale HPC centers connect scientists worldwide.
Without HPC resources, our climate models would be slower and less accurate. That would leave us less prepared for extreme weather events.
The Importance of Spatial Resolution
Spatial resolution is the size of each grid cell in a climate model. A coarse grid might treat a 100-kilometer region as a single cell. A finer grid can look at every 10 kilometers or even less. This higher resolution uncovers local weather patterns like storms or heat waves.
How Resolution Shapes Our View
- Fine resolution: Shows local floods, temperature spikes, or changing ocean currents.
- Coarse resolution: Gives a broad outline but may miss local details.
- Balancing act: Higher resolution requires more computing power.
Supercomputers solve this balancing act by offering enough speed and memory to handle fine details. This leads to more precise climate predictions that can guide local policies.
Challenges in Climate Modeling
Even with powerful HPC resources, climate modeling faces major hurdles. Researchers must handle immense datasets and couple different types of models. Energy consumption is also a growing concern as HPC centers expand. Let’s look at each challenge in detail.
1. Data Management
Climate models generate massive datasets. A single simulation can create terabytes or even petabytes of information. Storing and transferring these files is not simple.
Key data management steps include:
- Compression: To reduce file sizes without losing key information.
- Efficient storage: Using fast storage systems to manage large outputs.
- Data sharing protocols: Allowing teams in different locations to collaborate.
Better data management tools ensure quick access for researchers. This also helps maintain data quality, which is vital for solid climate projections.
2. Model Coupling
Model coupling means linking separate models—like atmosphere and ocean models—into one framework. Each model has its own math, timelines, and storage methods. Syncing them is not always easy.
Common model coupling issues:
- Different time scales: The ocean responds more slowly than the atmosphere.
- Varied data formats: Each model may save data differently.
- Communication lags: Models must pass data back and forth in real time.
Scientists use specialized software to unite these pieces. This creates a single, unified climate simulation. When it works well, the results are more accurate than treating each piece in isolation.
3. Energy Consumption of Large HPC Centers
Supercomputers run day and night to process climate models. This demands a lot of electricity. Many HPC facilities also need special cooling systems to avoid overheating.
Ways HPC centers reduce energy use:
- Efficient hardware: Low-power processors and power-saving designs.
- Renewable energy sources: Solar or wind power to run large data centers.
- Optimized software: Code that reduces wasted computing cycles.
Balancing performance with sustainability is key. HPC centers are adopting green strategies to cut their carbon footprint. This way, climate scientists don’t contribute heavily to the problems they’re trying to solve.
Advancements in HPC and Climate Science
New technologies are pushing climate modeling to new heights. GPUs (Graphics Processing Units) and specialized machine-learning methods are speeding up calculations. Researchers can now test many scenarios faster than before.
Emerging Trends
- Exascale computing: Supercomputers capable of a billion billion (10^18) calculations per second.
- Cloud-based HPC: Allows on-demand computing resources for smaller institutions.
- AI-driven analytics: Helps scientists quickly spot patterns in large climate datasets.
These advancements point to more accurate and timely climate forecasts. They also make climate modeling more accessible to a global network of researchers.
How Better Climate Models Help Communities
Communities rely on accurate forecasts to plan for droughts, floods, or heat waves. Farmers use climate data to guide planting seasons. City planners design infrastructure to handle extreme weather. Insurance companies update policies based on long-term risk. All these efforts depend on the quality of climate models.
Practical Applications
- Flood risk mapping: Identifies areas prone to heavy rainfall or rising rivers.
- Drought management: Provides early warnings for water shortages.
- Coastal planning: Prepares regions for sea-level rise.
- Wildfire prevention: Pinpoints dry areas where fires may spread.
Better climate models mean better decisions. This leads to safer, more resilient communities.
Getting Involved and Staying Informed
You don’t need to be a climate scientist to care about climate modeling. You can support organizations that fund research. You can also share accurate information about climate change in your community.
Simple Ways to Help
- Volunteer with local environmental groups.
- Stay updated by following reputable climate news sources.
- Encourage schools to teach climate science topics.
- Advocate for clean energy policies where you live.
Each action helps build a stronger network of people who understand and care about our planet. When more people know how climate modeling works, the push for solutions gains power.
Conclusion
Climate modeling on supercomputers is vital for understanding our changing climate. It helps scientists reveal how the atmosphere, oceans, ice, and land connect. High-performance computing expands the reach of these models, improving their accuracy and speed. Yet, challenges like data management, model coupling, and energy usage demand ongoing innovation.
We all benefit from clearer climate projections. Cities can plan for floods, farmers can prepare for droughts, and coastal areas can brace for rising seas. By supporting climate modeling and HPC, we take a step toward a safer future. The choices we make today about research funding and sustainable computing will shape the world tomorrow.
Author Profile
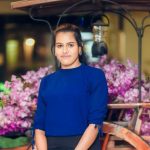
- Online Media & PR Strategist
- Hello there! I'm Online Media & PR Strategist at NeticSpace | Passionate Journalist, Blogger, and SEO Specialist
Latest entries
Scientific VisualizationApril 30, 2025Deepfake Scientific Data: AI-Generated Fraud in Research
Data AnalyticsApril 30, 2025What Is Data Mesh Architecture and Why It’s Trending
Rendering and VisualizationApril 30, 2025Metaverse Rendering Challenges and Opportunities
MLOpsApril 30, 2025MLOps 2.0: The Future of Machine Learning Operations