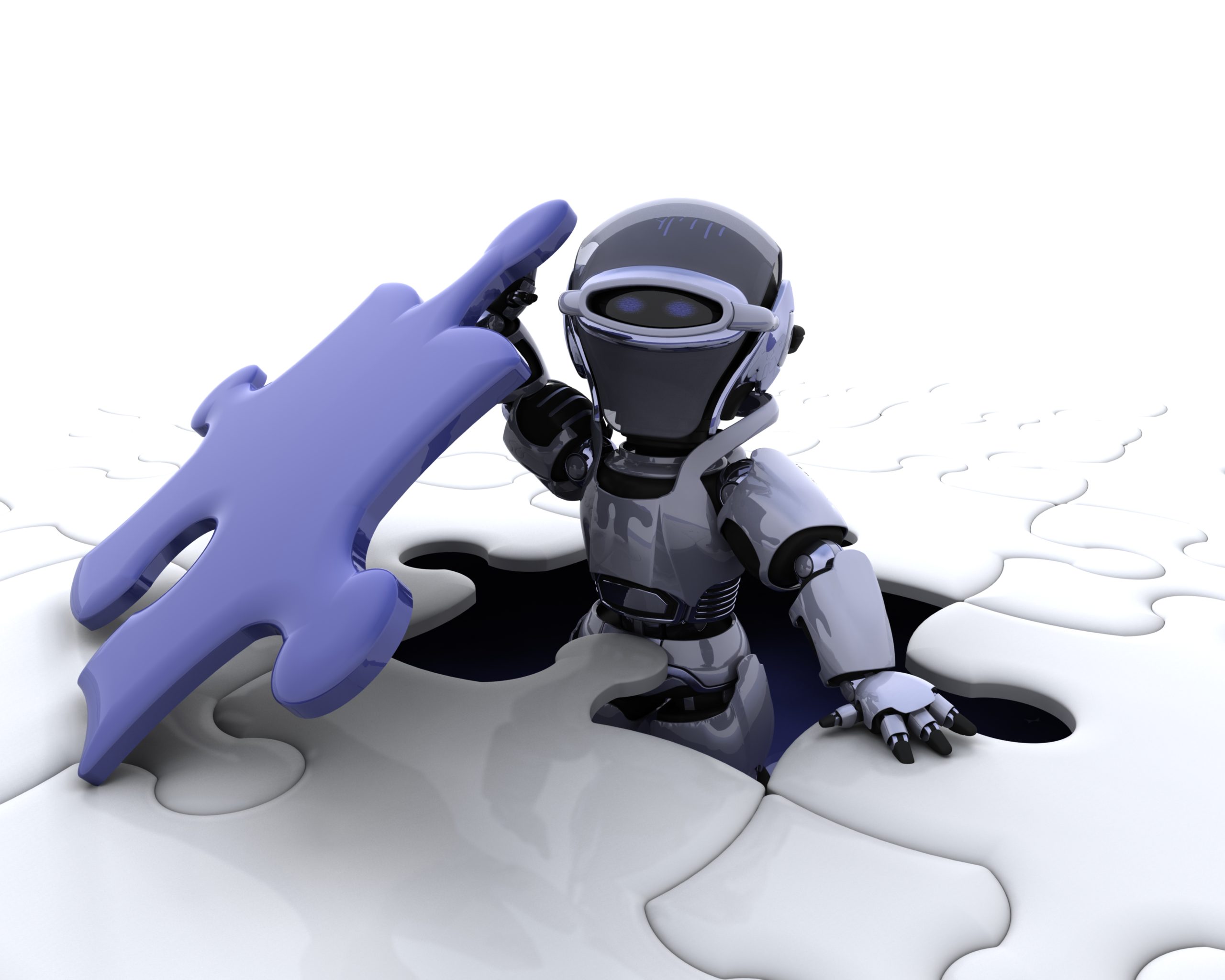
Open-Source Tools in AI & HPC: Boost Innovation and Efficiency
Written by Adithya Salgadu
Table of Contents
ToggleOpen-source software is changing the way we develop technology. It powers countless applications in the fields of AI and HPC (High-Performance Computing). In this post, you will learn about the benefits of using open-source tools, the top frameworks available today, and how to pick the right ones for your projects.
Why Open-Source Matters
Open-source code is publicly available. Anyone can inspect it, modify it, or share it. This transparency fosters innovation. Developers from around the world collaborate to improve open-source tools, making them more reliable, flexible, and cost-effective.
When it comes to AI and HPC, open-source solutions provide:
- Faster innovation through community-driven updates
- Lower costs by removing licensing fees
- Greater control over customization and scaling
Because of these advantages, open-source platforms are popular in both research and industry. Next, we’ll explore the top options that can help you jumpstart your AI and HPC projects.
The Role of Open-Source in AI
Artificial Intelligence (AI) depends on large datasets and powerful algorithms. Open-source libraries and frameworks simplify model building and deployment, whether you’re a newcomer or a seasoned expert. Below are some of the most widely used AI tools:
1. TensorFlow
- Developed by Google’s Brain team
- Supports deep learning across multiple platforms
- Offers pre-built models for computer vision and natural language processing
2. PyTorch
- Backed by Meta (Facebook)
- Famous for its dynamic computation graph, which eases model debugging
- Boasts a strong community with rapid updates
3. Scikit-learn
- A go-to library for machine learning tasks (regression, classification, clustering)
- Simple API, perfect for beginners and researchers alike
- Integrates smoothly with other Python tools like NumPy and Pandas
4. Hugging Face Transformers
- Specializes in natural language processing (NLP)
- Provides easy-to-use, pre-trained language models
- Regular updates and user-friendly tutorials
These frameworks are powerful yet flexible. By using them, you can build AI models that address tasks like image recognition, speech synthesis, and text summarization.
The Power of Open-Source in HPC
High-Performance Computing (HPC) helps you solve massive computational tasks. It’s used in scientific research, data analytics, and even weather forecasting. Below are some open-source solutions that excel in HPC environments:
1. OpenMPI
- A library that enables parallel processing across multiple nodes
- Helps accelerate computations for simulations and data analysis
- Works well with various hardware configurations
2. SLURM
- A job scheduler that handles resource allocation in clusters
- Used by many supercomputers for reliability and efficiency
- Includes tools for monitoring and managing large-scale jobs
3. Warewulf
- Simplifies the deployment of HPC clusters
- Offers bare-metal provisioning to set up systems quickly
- Active community focused on improving cluster management
4. OpenFOAM
- Focuses on computational fluid dynamics (CFD)
- Widely adopted for simulations in engineering and scientific domains
- Easily extensible through additional modules
When merged with robust hardware, these open-source tools can handle extreme workloads. They reduce the time needed to crunch complex data and enable more in-depth research.
Reasons to Choose Open-Source for AI and HPC
- Transparency
With open-source, you can review the code yourself. This ability to inspect every detail enhances security and trust in the software. - Community Support
Open-source tools typically have large user communities. This means you’ll find tutorials, forums, and add-ons created by others who faced similar challenges. - Scalability
Many open-source frameworks are designed with scalability in mind. You can start small and then expand to multi-GPU or multi-node setups as your needs grow. - Cost Savings
Avoiding expensive licenses frees up your budget for hardware improvements or hiring specialized talent.
How to Pick the Right Open-Source Tools
Choosing an open-source tool for AI or HPC doesn’t have to be daunting. Focus on these factors to make a smart decision:
- Project Goals
Clarify what you want to achieve. For instance, if you’re building a deep learning application, libraries like TensorFlow or PyTorch may be ideal. - Community Activity
Look at the project’s GitHub repository or forums. Frequent commits and large communities suggest the tool is actively maintained. - Documentation Quality
Good documentation speeds up learning and helps you troubleshoot issues. - Licensing
Review the license to ensure it fits your organization’s requirements. Most open-source AI and HPC tools use permissive licenses like Apache 2.0 or MIT.
Best Practices for Implementation
Even the best open-source tools need the right approach to deliver maximum value:
- Start Small
Pilot projects help you evaluate how the tool integrates with your workflows. - Engage with the Community
Ask questions in forums or contribute to the project. This two-way interaction enriches your understanding and helps others too. - Invest in Training
Provide learning resources for your team. Skilled users will unlock more value from the tools. - Monitor Performance
Track resource usage, execution times, and costs. Use this data to optimize your setups.
Conclusion: Harness the Power of Collaboration
Open-source tools offer unmatched flexibility and community support. They’re the cornerstone of AI and HPC development for many organizations, from small startups to global research institutions. Whether you need advanced machine learning features or robust cluster management solutions, open-source frameworks deliver on performance, scalability, and cost.
By choosing the right tools and best practices, you can accelerate innovation and solve complex problems more efficiently. Open-source isn’t just about saving money; it’s about nurturing a culture of collaboration. Embrace these solutions to stay competitive and drive meaningful breakthroughs in your projects.
Frequently Asked Questions (FAQ)
1. Is open-source software reliable enough for critical AI and HPC projects?
Yes. Many large companies and research labs depend on open-source frameworks. Popular tools often have dedicated teams and large communities that help ensure quality and stability.
2. Can I combine multiple open-source tools for one project?
Absolutely. It’s common to use more than one framework or library in AI and HPC. For example, you might use PyTorch for deep learning and SLURM for cluster management.
3. What if I need professional support?
Many open-source projects have commercial backing. You can purchase support or training from providers if you need professional assistance.
4. Do open-source tools fit small and large teams?
Yes. Startups can quickly adopt them without high licensing costs. Large enterprises also benefit from scalable solutions and broad community input.
5. Where can I learn more about these tools?
Explore their official documentation, GitHub repositories, and user forums. You’ll find tutorials, best practices, and sample code to guide your learning journey.
Author Profile
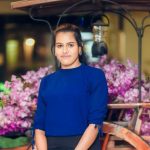
- Adithya SalgaduOnline Media & PR Strategist
- Hello there! I'm Online Media & PR Strategist at NeticSpace | Passionate Journalist, Blogger, and SEO Specialist
Latest entries
Digital Twin DevelopmentApril 30, 2025How to Ensure Data Synchronization Twins Effectively
Scientific VisualizationApril 30, 2025Deepfake Scientific Data: AI-Generated Fraud in Research
Data AnalyticsApril 30, 2025What Is Data Mesh Architecture and Why It’s Trending
Rendering and VisualizationApril 30, 2025Metaverse Rendering Challenges and Opportunities