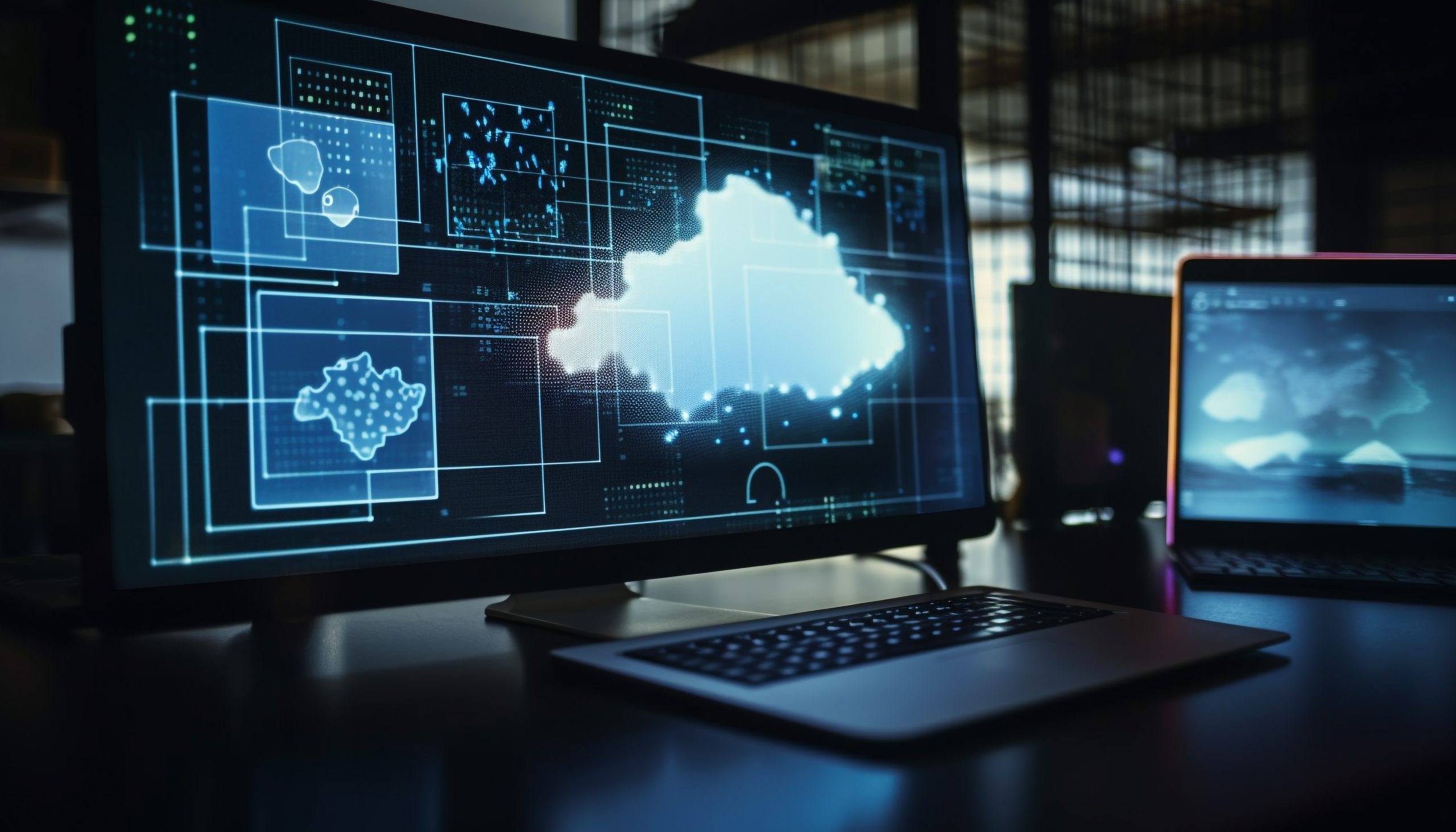
The Challenges of Multi-Cloud MLOps and How to Solve Them
Multi-cloud MLOps is becoming a top priority for many organizations. Leaders want to leverage the best cloud platforms for machine learning (ML). But juggling different infrastructures can lead to complications. In this blog, you’ll learn the core challenges of multi-cloud MLOps and how to solve them.
Why Multi-Cloud MLOps Matters
Multi-cloud MLOps is about using multiple cloud providers to host and manage ML pipelines. Each platform offers unique services. Some are better for data storage, while others excel at compute power.
First, multi-cloud strategies help avoid vendor lock-in. Businesses can pick the most cost-effective solutions. Next, you get flexibility. If one cloud faces an outage, you can switch to another provider. Finally, multi-cloud MLOps fosters innovation. It opens up a range of specialized ML services across clouds.
Common Challenges in Multi-Cloud MLOps
Despite its benefits, multi-cloud MLOps presents real obstacles. These challenges can stall projects and increase costs. Below are the main issues you should consider.
1. Data Security and Governance
Data often lives in different locations. This makes it harder to ensure privacy and compliance. Each cloud follows its own security model and governance rules. You must confirm that data transfer between clouds is secure. Failing to do so could violate regulations such as GDPR or HIPAA.
2. Network Latency and Connectivity
Moving large ML datasets across multiple clouds is no small task. Network latency can slow training and inference. This delay can make real-time analytics much less effective. Additionally, connectivity issues may result in inconsistent data synchronization. Planning for efficient network usage is key to keeping ML systems responsive.
3. Tooling and Integration
Each cloud provider often has its own set of tools. This can make it tricky to create unified processes for development, testing, and deployment. MLOps pipelines need to remain consistent across these platforms. Teams must learn multiple frameworks, which adds complexity. Without the right approach, you’ll end up with siloed projects that are hard to manage.
4. Cost Management
Multi-cloud MLOps can become expensive if costs aren’t monitored. Using multiple providers means varying pricing models. It’s easy to overspend on storage, computing, or data transfer. Without clear visibility, costs might spiral out of control. Proper budgeting strategies are critical to avoid unpleasant surprises.
Strategies to Overcome Multi-Cloud MLOps Obstacles
Next, let’s explore actionable solutions for multi-cloud MLOps. These strategies can help you streamline ML workflows, secure data, and keep costs in check.
1. Establish a Robust Data Governance Framework
- Define clear data policies: Outline who can access which data, under what conditions.
- Set up encryption in transit and at rest: This should apply across all cloud platforms.
- Audit regularly: Conduct security audits to ensure continuous compliance with industry standards.
Having a unified framework helps avoid confusion. It also simplifies compliance checks. You’ll minimize risk and maintain user trust.
2. Optimize Connectivity for Faster Workflows
- Use cloud regions wisely: Choose locations close to your user base or data sources.
- Leverage Content Delivery Networks (CDNs): CDNs reduce latency when serving ML models.
- Adopt a hybrid approach: Store data in the most cost-effective region but keep crucial analytics close to your team.
Maintaining solid connectivity reduces downtime. It also shortens training cycles. Faster workflows translate to quicker insights for your business.
3. Standardize Tooling with Containers and Microservices
Containers, like Docker, simplify multi-cloud MLOps. They provide a consistent environment across platforms. Microservices help you break down large ML applications into smaller units.
- Container orchestration: Use Kubernetes or another tool for scaling.
- Version control for ML models: Track changes to ensure reproducibility.
- Centralized logging and monitoring: Keep an eye on every microservice for quick issue resolution.
By standardizing your tools, you reduce the chance of errors. Plus, you enable faster iteration on models.
4. Implement Automated Testing and CI/CD
Automation ensures smooth deployments and updates. Continuous Integration (CI) and Continuous Deployment (CD) pipelines can detect issues early. This helps you maintain consistent ML environments across multiple clouds.
- Unit testing and integration testing: Catch bugs in ML code before deployment.
- Automated model validation: Confirm model performance hasn’t regressed.
- Rollbacks: If a deployment fails, roll back to a stable state automatically.
Testing and CI/CD keep multi-cloud MLOps pipelines efficient. They reduce manual workload and speed up releases.
5. Monitor Costs and Resource Usage
Cost monitoring is vital in a multi-cloud environment. Different providers may charge more for specific operations.
- Consolidate billing data: Use a single dashboard to track spending across providers.
- Set budgets and alerts: Avoid unexpected charges by setting automated alerts.
- Right-size resources: Scale compute and storage to fit current ML project needs.
A proactive approach to cost management helps you optimize ROI. You avoid overprovisioning and pay for only what you need.
Driving Growth with Multi-Cloud MLOps
Finally, multi-cloud MLOps offers immense possibilities. You can avoid vendor lock-in and leverage best-in-class services. Yet, you must address security, connectivity, tooling, and cost challenges.
By applying the strategies in this article, you can overcome many obstacles. You’ll streamline your ML operations and deliver valuable insights faster. When done right, multi-cloud MLOps drives innovation, keeps data protected, and optimizes spending.
Frequently Asked Questions
Q1: What is multi-cloud MLOps?
Multi-cloud MLOps involves developing and deploying machine learning models across multiple cloud providers. This approach lets organizations use the best services each provider offers while avoiding vendor lock-in.
Q2: How do I manage data security in a multi-cloud environment?
Use encryption, secure connections, and strong access controls. Implement a clear data governance framework. Conduct regular compliance audits to meet industry regulations.
Q3: How can I reduce network latency across clouds?
Choose cloud regions close to your data sources or users. Use CDNs to speed up content delivery. Also consider a hybrid approach, keeping critical analytics near your main operations.
Q4: Are containers helpful for multi-cloud MLOps?
Yes, containers standardize the deployment environment. They make it easier to manage machine learning services across various platforms. Tools like Kubernetes can automate scaling and resource usage.
Q5: How do I control costs in multi-cloud MLOps?
Monitor spending across providers. Set budgets and automated alerts. Right-size your resources to ensure you only pay for what you need.
Author Profile
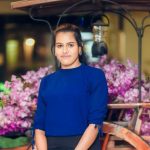
- Online Media & PR Strategist
- Hello there! I'm Online Media & PR Strategist at NeticSpace | Passionate Journalist, Blogger, and SEO Specialist
Latest entries
Scientific VisualizationApril 30, 2025Deepfake Scientific Data: AI-Generated Fraud in Research
Data AnalyticsApril 30, 2025What Is Data Mesh Architecture and Why It’s Trending
Rendering and VisualizationApril 30, 2025Metaverse Rendering Challenges and Opportunities
MLOpsApril 30, 2025MLOps 2.0: The Future of Machine Learning Operations