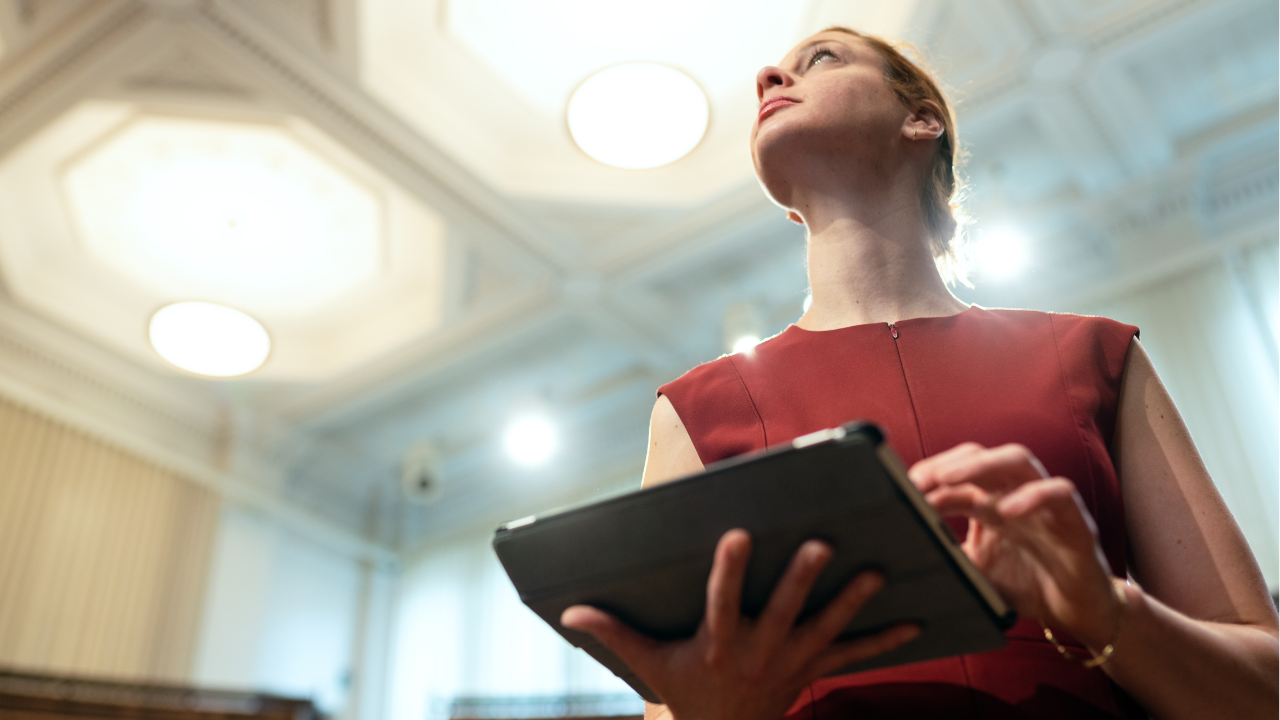
Beginner’s Guide to Google BigQuery Analysis Setup
Want to explore big data tools but don’t know where to start? With Google BigQuery analysis, you can process large datasets quickly and efficiently—using just SQL and a browser.
In this guide, you’ll learn the fundamentals of Google BigQuery from setting it up to writing your first queries. Whether you’re a beginner or an IT professional, you’ll walk away with the confidence to start analyzing cloud data right away.
What Is Google BigQuery Analysis and Why Use It?
Google BigQuery analysis refers to using Google’s fully managed data warehouse for querying massive datasets. Since it runs on the Google Cloud Platform (GCP), it scales automatically without any need for infrastructure setup.
Why Choose Google BigQuery?
-
Easy to use with standard SQL
-
Fast processing, even for terabytes of data
-
Scalable and secure
-
Seamlessly integrates with other Google tools
Most importantly, it lets you focus on analysis instead of infrastructure.
How to Set Up Google BigQuery Analysis
To begin using Google BigQuery, follow these steps:
Step 1: Create a Google Cloud Project
First, go to the Google Cloud Console, then create a project. Afterward, enable billing to unlock BigQuery features.
Step 2: Enable the BigQuery API
Next, open the APIs section in your dashboard. Search for BigQuery API, and enable it for your project.
Step 3: Create a Dataset
Then, head to BigQuery in the GCP menu. Click Create Dataset, name it, and choose the location.
Step 4: Import Your Data
You can load CSV files, connect Google Sheets, or link external sources like Cloud Storage.
By the end of this setup, you’re ready to run queries.
Running Queries in Google BigQuery Analysis
Now that your environment is ready, it’s time to execute your first Google BigQuery analysis query.
A Simple Query Example
Here’s a basic query that counts rows:
Helpful Query Tips
-
Use
LIMIT
to reduce data load -
Filter data using
WHERE
-
Group data with
GROUP BY
-
Preview tables to estimate costs
These best practices will help you analyze data efficiently and avoid surprises in billing.
Best Practices for Google BigQuery Analysis
To get the most from Google BigQuery analysis, follow these performance and cost tips.
Boost Performance
-
Use partitioned and clustered tables
-
Avoid
SELECT *
for production queries -
Preview data before running full queries
Control Costs
-
Set query limits or budgets
-
Use cost estimation tools
-
Schedule large jobs during off-peak hours
Taking these steps will help you stay both efficient and budget-friendly.
Top Use Cases for Google BigQuery Analysis
Many industries benefit from Google BigQuery , including:
Common Applications
-
Marketing: Track user behavior and campaign ROI
-
Finance: Perform fraud detection and real-time reports
-
Healthcare: Analyze patient trends and treatment outcomes
-
Retail: Forecast inventory and understand customer behavior
As a result, businesses gain faster and smarter insights from their data.
Frequently Asked Questions (FAQ)
What is Google BigQuery used for?
Google BigQuery is used to run fast SQL queries on large datasets without server maintenance.
Is there a free tier for BigQuery?
Yes, Google offers 10 GB storage and 1 TB of queries per month for free.
Do I need SQL knowledge to use BigQuery?
Absolutely. Basic SQL is required to write queries and analyze data in BigQuery.
Can I visualize BigQuery results?
Yes. Tools like Looker Studio, Tableau, and Power BI connect easily with BigQuery.
Conclusion: Start Your Google BigQuery Analysis Today
Learning Google BigQuery doesn’t have to be hard. Now that you know how to set up a project, run queries, and apply best practices, you’re ready to start analyzing data in the cloud.
BigQuery is fast, flexible, and beginner-friendly—making it a powerful tool for any data-driven organization.
Author Profile
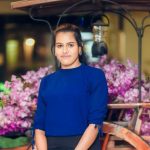
- Online Media & PR Strategist
- Hello there! I'm Online Media & PR Strategist at NeticSpace | Passionate Journalist, Blogger, and SEO Specialist
Latest entries
Computer Aided-EngineeringJuly 9, 2025Parametric Optimization Techniques for Best Design
Computer Aided-EngineeringJuly 9, 2025Modal Vibration Analysis: Natural Frequencies & Responses
NetworkingJuly 9, 2025Networking Challenges Healthcare: Key Issues & Fixes
NetworkingJuly 9, 2025Edge Computing Impact on Network Infrastructure