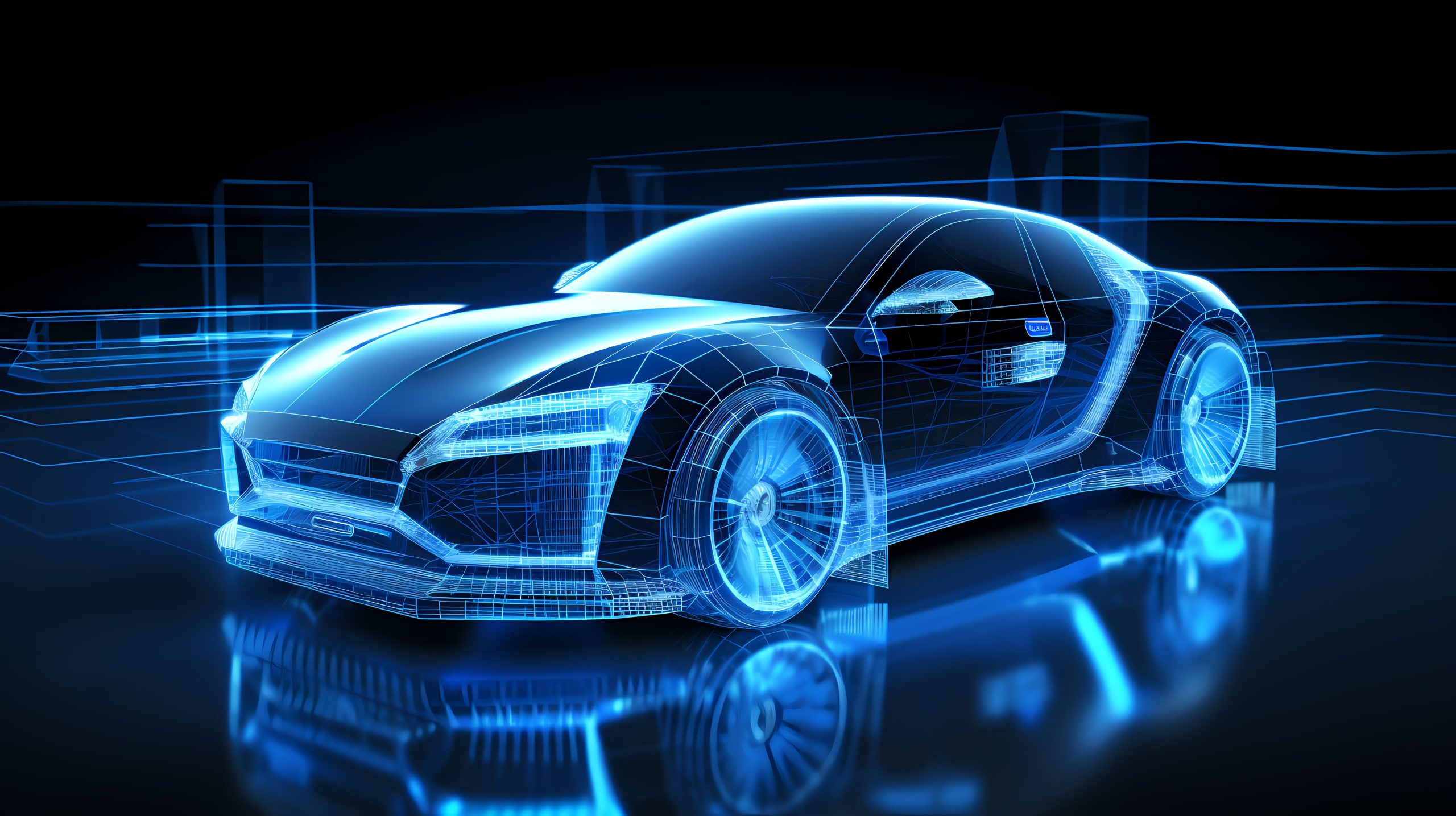
AI Simulation & Training | HPC for Autonomous Vehicles
The role of HPC in autonomous vehicle development is crucial for training AI systems to safely navigate roads. Before self-driving cars hit the streets, their AI models must be rigorously tested and optimized. High-Performance Computing (HPC) enables this process by running large-scale simulations and deep-learning models at rapid speeds.
In this article, we’ll explore how HPC powers AI training and testing for self-driving cars, improving safety, efficiency, and development time. Learn more about AI’s role in modern transportation.
What is HPC and Why Does It Matter for Autonomous Vehicles?
High-Performance Computing (HPC) refers to supercomputers that can process massive amounts of data at high speeds. Unlike regular computers, HPC systems can run complex simulations and AI training much faster and more efficiently.
For self-driving cars, HPC is essential because:
- It enables real-time data processing from multiple sensors.
- It helps train AI models with vast amounts of driving data.
- It allows for extensive simulation before real-world deployment.
Explore our guide on AI in automotive technology to dive deeper into this topic.
How HPC Powers AI Training for Self-Driving Cars
1. Handling Large Datasets
Self-driving cars generate petabytes of data from sensors like LiDAR, cameras, and radar. The role of HPC in autonomous vehicle development is to process and analyze this data efficiently, training AI models on realistic driving scenarios.
2. Running Advanced AI Algorithms
Deep learning models used in AVs require immense computational power. HPC accelerates the training process by running multiple AI models simultaneously, reducing the time needed to improve accuracy.
Discover more about deep learning in self-driving cars.
3. Optimizing Machine Learning Models
HPC helps fine-tune AI models, allowing them to:
- Recognize objects like pedestrians, vehicles, and road signs.
- Predict movement patterns and make safe driving decisions.
- Improve through continuous learning and retraining.
Simulation & Testing: How HPC Ensures AV Safety
1. Virtual Testing Environments
Before AVs are tested on real roads, they undergo millions of miles of virtual driving. HPC-powered simulations allow developers to:
- Test various driving conditions (e.g., rain, fog, traffic congestion).
- Identify and fix issues before deploying AVs in real-world scenarios.
Check out our latest article on self-driving simulations.
2. Running Complex Simulations
HPC enables:
- Monte Carlo simulations to test AV decision-making in uncertain environments.
- Real-time simulation of traffic flow and pedestrian behavior.
- Edge case testing to handle rare but critical situations.
3. Reducing Costs & Improving Efficiency
Real-world testing is expensive and time-consuming. HPC helps reduce costs by allowing extensive testing in a controlled, virtual setting before moving to on-road trials.
Challenges of Using HPC in Autonomous Vehicle Development
1. High Computational Costs
HPC systems require significant investment in hardware, software, and power consumption.
2. Data Management Complexity
Processing and storing massive datasets demand efficient data pipelines and high-speed storage solutions.
3. Continuous Model Updates
As AI models improve, they require constant updates, increasing computational demands and resource allocation.
Future of HPC in Autonomous Driving
As technology advances, HPC will continue to revolutionize autonomous vehicle development. Innovations such as:
- Quantum computing may further accelerate AI training.
- Edge computing could optimize real-time data processing in AVs.
- Cloud-based HPC solutions will make high-performance computing more accessible to automotive companies.
FAQs
1. Why is HPC important for self-driving cars?
The role of HPC in autonomous vehicle development is to enable fast AI training, real-time sensor data processing, and large-scale simulations, ensuring AVs are safe and reliable before deployment.
2. How does HPC help reduce self-driving car development time?
By running parallel computations, HPC speeds up AI training and simulations, cutting down the time required for model optimization and validation.
3. What are the biggest challenges of using HPC in AV development?
High costs, data management complexity, and the need for continuous AI model updates are major challenges.
4. Can cloud computing replace HPC for AV development?
Cloud-based HPC solutions can complement traditional HPC, but on-premise HPC remains crucial for ultra-fast, large-scale simulations.
Author Profile
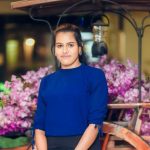
- Online Media & PR Strategist
- Hello there! I'm Online Media & PR Strategist at NeticSpace | Passionate Journalist, Blogger, and SEO Specialist
Latest entries
Scientific VisualizationApril 30, 2025Deepfake Scientific Data: AI-Generated Fraud in Research
Data AnalyticsApril 30, 2025What Is Data Mesh Architecture and Why It’s Trending
Rendering and VisualizationApril 30, 2025Metaverse Rendering Challenges and Opportunities
MLOpsApril 30, 2025MLOps 2.0: The Future of Machine Learning Operations