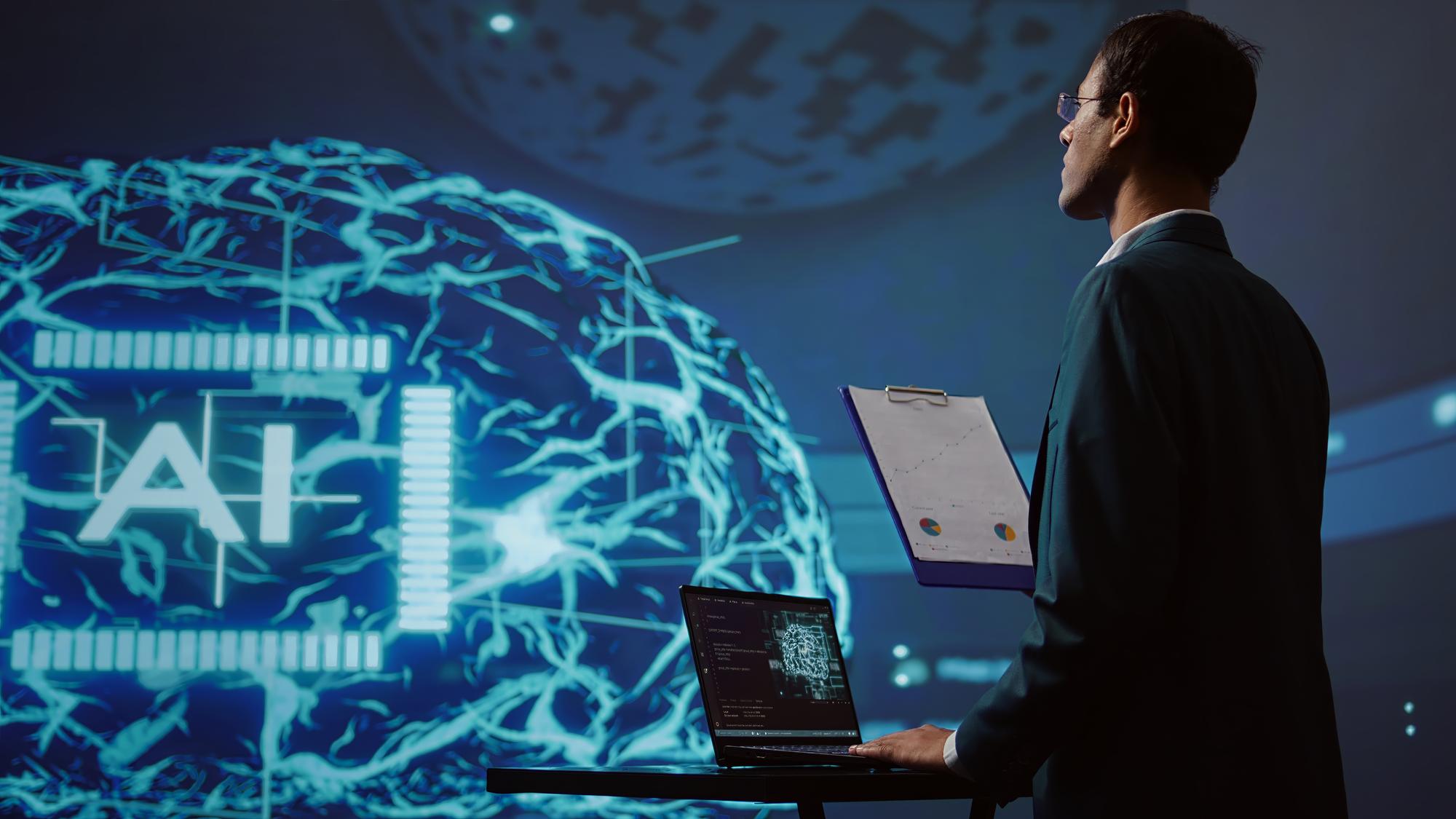
Predictive Analytics with Machine Learning
Predicting the future may sound impossible. But with machine learning, we can make smart guesses based on data. In this guide, you’ll learn how to perform predictive analytics using machine learning in a simple and practical way.
Whether you’re a business owner or a beginner in data science, this article will help you understand the steps involved and how to get started.
What is Predictive Analytics?
Predictive analytics is the use of data, statistics, and machine learning to forecast future outcomes. Companies use it to predict customer behavior, market trends, and more.
Why is it important?
- It helps businesses make better decisions
- Reduces risk by predicting future issues
- Improves customer experiences
How Machine Learning Powers Predictive Analytics
Machine learning helps computers learn from data and make predictions without being directly programmed.
Key benefits of using machine learning:
- Processes large amounts of data quickly
- Improves accuracy over time
- Adapts to new patterns or changes
Step-by-Step Guide to Perform Predictive Analytics Using Machine Learning
1. Define Your Goal
Before anything else, you need to know what you want to predict.
Examples:
- Will a customer cancel their subscription?
- What will next month’s sales be?
2. Collect and Prepare Data
You can’t predict anything without data. Gather data from reliable sources like:
- Customer records
- Website traffic logs
- Sales reports
Then, clean your data:
- Remove duplicates
- Fix missing values
- Format data for analysis
3. Choose the Right Machine Learning Model
There are many types of models. Pick one that fits your goal.
Common models used:
- Linear regression: For predicting numbers (like sales)
- Decision trees: For predicting choices (like churn yes/no)
- Random forests: A mix of decision trees for better accuracy
4. Split Your Data
Split your data into:
- Training set: To teach the model
- Test set: To check the model’s accuracy
5. Train the Model
Use your training data to teach the machine learning model how to spot patterns.
6. Test and Improve
After training, test the model with the test set. Check how accurate the predictions are.
If accuracy is low:
- Try a different model
- Add more data
- Improve data quality
7. Deploy and Monitor
Once your model is accurate:
- Use it in real-world systems
- Monitor predictions regularly
- Update the model as new data comes in
Real-Life Uses of Predictive Analytics with Machine Learning
In Retail
- Predict what products customers will buy
- Forecast inventory needs
In Healthcare
- Predict patient health risks
- Improve diagnosis speed and accuracy
In Finance
- Detect fraud
- Predict stock price movements
Tips for Success with Predictive Analytics
- Always use clean, quality data
- Start small and scale up
- Keep improving your model with new data
- Work with domain experts who understand your business
FAQ: Predictive Analytics and Machine Learning
What is the difference between predictive analytics and machine learning?
Predictive analytics is the process. Machine learning is the tool used to make predictions.
Can small businesses use predictive analytics?
Yes. Many tools make it easy and affordable for small teams.
Do I need to be a programmer?
Not necessarily. Some tools offer drag-and-drop interfaces.
How accurate are machine learning predictions?
Accuracy depends on data quality, model type, and the problem you’re solving.
Conclusion
Knowing how to perform predictive analytics using machine learning empowers you to make data-driven decisions. Follow the steps in this guide, start small, and continually refine your methods. With consistent effort, you’ll soon uncover powerful insights that drive your business forward.
Learn More About Machine Learning Basics
Author Profile
- Hey there! I am a Media and Public Relations Strategist at NeticSpace | passionate journalist, blogger, and SEO expert.
Latest entries
Scientific VisualizationJune 27, 2025Corporate ESG: Guide to Green IT and Smart Tech Buying
Scientific VisualizationJune 27, 2025Scientific Visualization in Medicine: MRI & Surgery Tech
Vehicle SimulationJune 24, 2025How Vehicle Simulation Training is Transforming Driver Programs
High Performance ComputingJune 20, 2025How Quantum Computing Power Is Transforming Technology