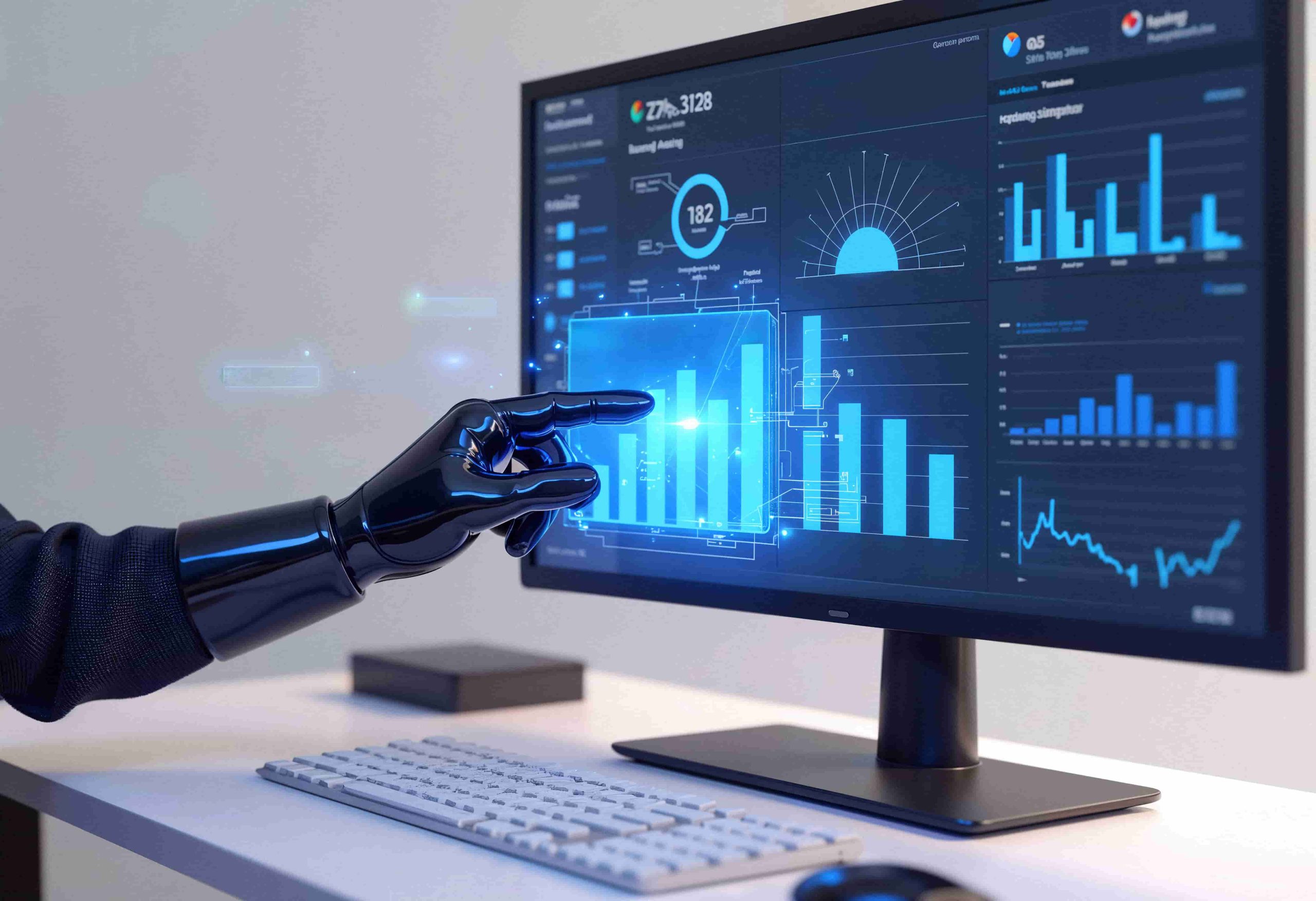
How to Reduce Bias in Data Analytics for Better Decisions
Bias in data can ruin your business decisions. It leads to unfair results, bad insights, and costly mistakes. In this article, you’ll learn what causes bias, its risks, and how to reduce it in analytics.
Whether you’re in IT, marketing, or finance, understanding this risk is key to making better choices.
What Is Bias in Analytics?
Bias happens when the information used doesn’t reflect reality correctly. This can happen at any stage—from data collection to how it’s processed and analyzed.
Main Types of Bias
-
Selection bias – Data doesn’t include everyone it should.
-
Measurement bias – Inaccurate tools or processes affect results.
-
Historical bias – Data reflects old values or systems.
-
Confirmation bias – Analysts favor data that supports their views.
Even if unintentional, these can severely affect the quality of insights.
Why Bias in Analytics Is a Serious Risk
Ignoring bias can have consequences. It affects everything from decision-making to customer trust.
The Risks:
-
Poor business decisions from inaccurate trends
-
Reputation damage from unfair practices
-
Legal issues in hiring, finance, or healthcare analytics
-
Missed market opportunities due to distorted insights
Sources of Bias in Data
Where does bias start? Here are a few common sources:
1. People
Those collecting or analyzing data may have hidden biases.
2. Incomplete Data
Overrepresenting or underrepresenting groups skews the results.
3. Legacy Systems
Old datasets may include outdated or discriminatory assumptions.
4. Algorithm Design
If biased data trains the model, it continues to produce biased results.
How to Reduce Bias in Analytics
Let’s look at practical ways to minimize this issue:
1. Use Diverse Data Sources
Balance the data with inputs from multiple audiences or demographics.
2. Conduct Data Audits
Regular checks help you identify missing info or unfair patterns.
3. Design Fair Algorithms
Use models that adjust for known biases.
4. Involve Diverse Teams
Different people spot different issues. Collaboration helps catch blind spots.
5. Test and Validate Results
Review findings using multiple methods to ensure fairness and accuracy.
Real-World Impacts of Bias in Data
-
Hiring Platforms: AI systems trained on biased resumes have excluded qualified candidates.
-
Healthcare AI: Tools sometimes give less care advice to minorities due to non-diverse training data.
This shows how vital it is to catch and fix bias in data early.
Benefits of Bias-Free Analytics
When you reduce bias, your data becomes stronger and more ethical.
Key Benefits:
-
More accurate decisions
-
Increased public trust
-
Lower legal risk
-
Greater market insight
It’s not just about fairness—it’s good business too.
FAQs
What causes bias in data?
Common causes include human error, bad sampling, and old or flawed datasets.
Can we remove all bias from data?
No, but we can reduce it using fair practices and regular reviews.
How do I find bias in my analytics?
Look for missing data patterns, check source diversity, and audit results.
Reduce Bias for Smarter Insights
Bias leads to errors, unfairness, and risks. But with careful planning and review, you can build fairer and smarter analytics systems.
Want more help? Explore our Data Integrity Best Practices Guide to learn how industry leaders manage clean, ethical data.
Author Profile
- Hey there! I am a Media and Public Relations Strategist at NeticSpace | passionate journalist, blogger, and SEO expert.
Latest entries
AI WorkflowsMay 23, 2025Redefining the Future of IT with Google Gemini Innovation
Robotics SimulationMay 23, 2025Robotics Simulation Enhances Software Testing in Automation
HPC and AIMay 23, 2025Open-Source Tools in AI & HPC: Boost Innovation and Efficiency
Quantum ComputingMay 23, 2025Quantum Computing AI and Machine Learning