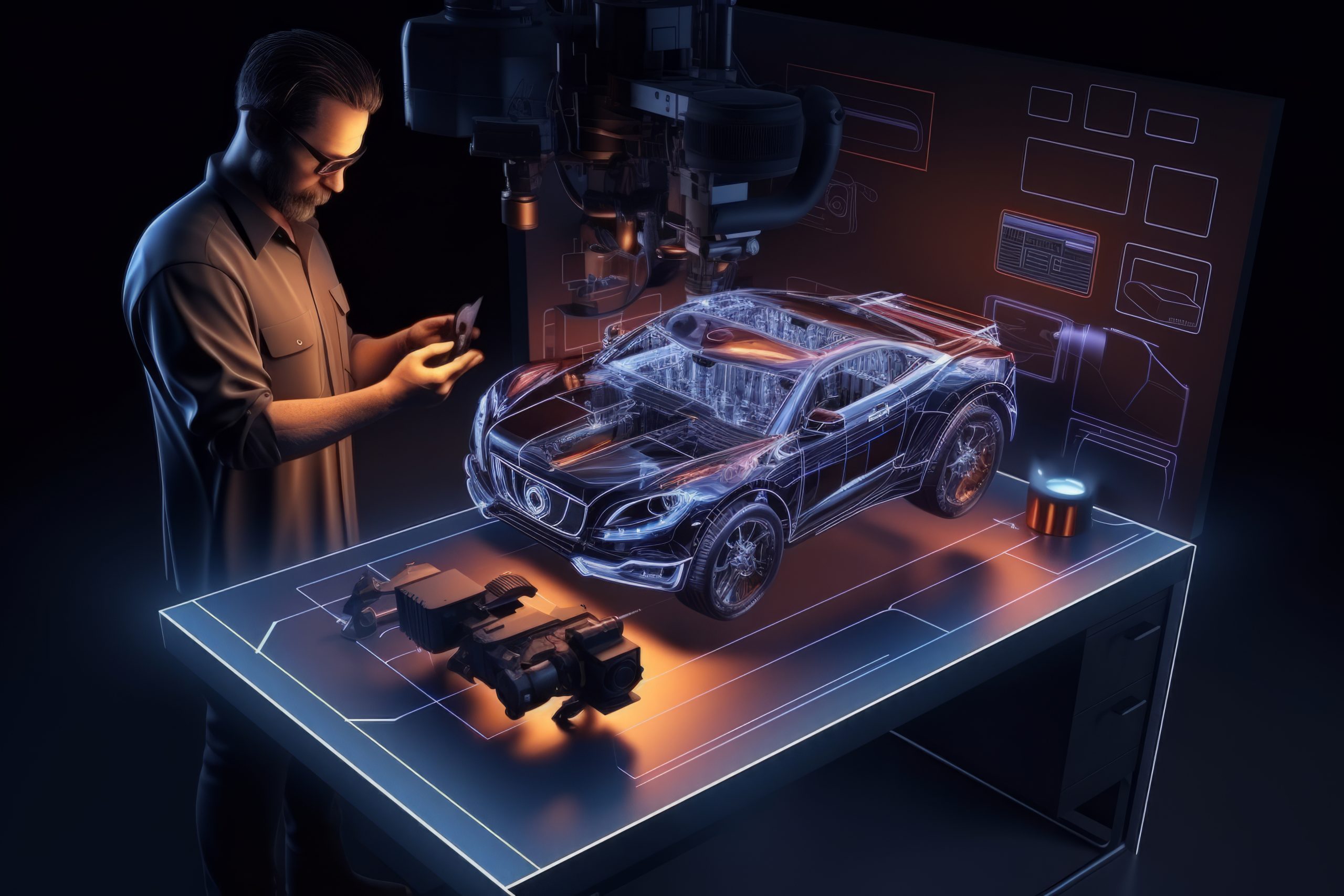
Can We Trust Digital Twin Accuracy in Simulations?
Simulations are everywhere. From designing aircraft to testing smart cities, companies use digital twin accuracy to make critical decisions. But what happens when these virtual models fail? In this article, you’ll learn when and why digital twin accuracy breaks down, the dangers of blind trust in models, and how to properly validate them.
Let’s dive into the limits of simulations—and what IT teams, engineers, and decision-makers should know before trusting the results.
Understanding Digital Twin Accuracy in IT and Engineering
Digital twin accuracy refers to how closely a virtual simulation matches real-world performance. A digital twin is a virtual replica of a physical system that helps organizations monitor, test, and predict behavior.
These models are widely used in:
-
Smart factories
-
Healthcare systems
-
Aerospace design
-
Energy grids
-
Transportation networks
But accuracy isn’t guaranteed.
When the data feeding the twin is flawed—or the model’s logic is off—the output can mislead. This can result in millions of dollars lost, system failures, or worse, safety risks.
Why Digital Twin Accuracy Can Fail in Real Applications
Model Assumptions Don’t Reflect Reality
All digital twins rely on assumptions. Whether it’s the environment, user behavior, or system stress, assumptions simplify reality to make models run faster. But if the real world changes, digital twin accuracy can collapse.
For example:
-
A smart grid simulation might assume consistent energy consumption.
-
But during extreme weather, consumption patterns shift dramatically.
Outdated or Incomplete Data
Digital twins require up-to-date data. If the data stream is delayed, missing, or biased, the model’s predictions won’t match reality.
-
Outdated sensor data = false alerts
-
Incomplete logs = missed risks
Overfitting or Overconfidence in the Model
Sometimes, simulations are tuned so closely to past data that they fail when conditions change. This “overfitting” limits adaptability. Teams may also place too much trust in high-accuracy scores without looking deeper into test coverage or bias.
How to Improve Digital Twin Accuracy in Simulations
Validate Against Real-World Outcomes
Run simulations in parallel with real-world testing. Compare the outcomes and continuously tweak the model. This helps align the digital twin with the physical system.
Use Diverse Data Sources
Feed your models with varied, high-quality data. Mix real-time sensor data with historical datasets to improve robustness.
Perform Sensitivity Analysis
This involves tweaking one variable at a time to see how much it affects the output. It helps uncover which inputs are most critical to digital twin accuracy.
Audit the Model Regularly
Create a standard checklist for simulation models. Review logic, data inputs, and output patterns every quarter. In regulated industries, document these audits for compliance.
The Role of AI and ML in Enhancing Digital Twin Accuracy
While buzzwords abound, AI and machine learning can help spot patterns that rule-based models miss. They can adapt in real time, improving digital twin accuracy as new data flows in.
However, blindly applying AI can backfire if:
-
The training data is biased
-
The model lacks transparency
-
The system can’t explain its results
Use explainable AI (XAI) and transparent algorithms for better trust and reliability.
Real-World Examples: When Digital Twins Go Wrong
Case 1: Boeing’s Flight Simulator Missed a Fault
In one well-known case, the flight model used in simulation didn’t account for sensor malfunctions. The oversight caused flawed testing results, leading to tragic real-world crashes.
Case 2: Smart Cities With Poor Data Integrity
A major city used a digital twin for traffic flow modeling. But sensor data had gaps. Predictions were way off, leading to gridlock during peak hours. Digital twin accuracy was never validated post-deployment.
FAQs
What is digital twin accuracy?
It’s how well a simulation model matches the performance of the actual system.
Can we trust simulation results?
Only after validating with real-world testing. Blind trust in simulations is risky.
How do you validate a digital twin?
Compare it with real-world outcomes, audit inputs, and run regular sensitivity analyses.
What industries use digital twins?
Manufacturing, aerospace, healthcare, energy, and smart city planning.
Can AI improve digital twin accuracy?
Yes, but only when used transparently and with good data.
Should You Trust Digital Twin Accuracy?
Digital twin accuracy can be powerful, but blind trust is dangerous. Always validate, audit, and test your simulation results. Treat digital twins as advisors, not oracles.
By building models carefully, auditing them often, and testing in real environments, you can improve trust—and outcomes.
Find more about Choose the Right Platform for Digital Twin Development
Author Profile
Latest entries
Computer Aided-EngineeringJuly 11, 2025CAE Technology Advancing Electronics: Future Trends
Computer Aided-EngineeringJuly 11, 2025Best Practices for CAE Simulation Validation
MLOpsJuly 8, 2025Scaling MLOps Kubernetes with Kubeflow Pipelines
MLOpsJuly 8, 2025How to Manage Feature Stores in MLOps Effectively